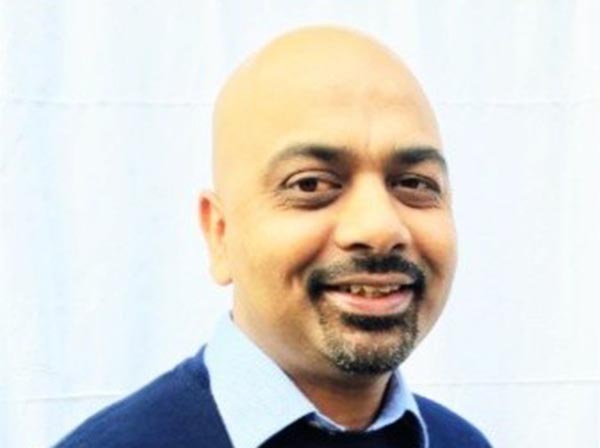
Click to learn more about Abhi Yadav
In most large organizations, artificial intelligence (AI) and machine learning (ML) are powering key business functions, from big data analytics and customer service to fraud detection and personalized marketing. Insights that AI and ML can produce are powerful, but it’s often difficult, if not impossible, to explain how these algorithms arrived at them. This limitation could pose significant problems for compliance with the EU’s General Data Protection Regulation (GDPR), the California Consumer Privacy Act (CCPA), and other laws governing data and privacy.
Let’s look at GDPR first. When an automated process such as AI or ML makes a decision about an individual based on personal data, GDPR requires the organization to supply an explanation if requested. But what GDPR means by “explanation” isn’t very clear. There are two competing interpretations. The direst for AI would require organizations to detail exactly how an algorithm arrived at its conclusions. Thankfully, the most common thinking is that GDPR simply requires letting people know that decisions pertaining to them will be made by an automated process.
GDPR makes AI Illegal?
Dr. Pedro Domingos, author of The Master Algorithmand a professor of computer science at the University of Washington, falls into the camp of those who subscribe to the first interpretation. He tweeted in 2018 that GDPR would “require algorithms to explain their output, making deep learning illegal.” He says this because most AI is a “black box,” and even the people who create ML algorithms and manage their use often cannot explain how a decision was reached – the processes are complex and opaque.
For example, Google’s AlphaGo AI achieved a long-time goal of artificial intelligence researchers when it beat a professional Go player in 2016. But while its play was clearly superior to that of its human opponent, the AI could not explain the rationale behind its moves, which were often bewildering even to professional players. For most AI and ML algorithms in use today, explanations are impossible to provide.
However, this is not how most AI and legal experts interpret GDPR’s explainability requirements. Dr. Sandra Wexler, a research fellow at Oxford’s Alan Turing Institute, writes in a 2017 blog post, that “GDPR is likely to only grant a ‘right to be informed’ about the existence of automated decision-making, (the fact that these methods are being used) and about system functionality (logic involved) as well as the significance (scope) and the envisioned consequences (intended purpose e.g. setting credit rates which can impact payment options) rather than offering reasons for how a decision was reached.”
In other words, if I apply for a credit card and an algorithm denies me, the credit card company would only need to disclose to me that they’re using AI or ML to determine eligibility, and not how the algorithm came to a decision in my specific case. Wexler notes that GDPR provides protection for “trade secrets or intellectual property and in particular the copyright protecting the software.” How these protections can be squared with requirements for transparency is still unresolved.
CCPA and AI/ML
The CCPA also has ramifications for AI and ML explainability. For example, the CCPA doesn’t just cover data that’s collected from consumers, but also additional data on consumers that’s created through inferences. A video streaming service, for example, might use AI to determine that a subscriber doesn’t like movies with subtitles. CCPA requires that this service be able to disclose not just the inferred data about the customer’s viewing preferences, but also all the personal data used to come to that conclusion, any of which may need to be deleted upon request. IT and marketing will likely have no idea what personal information the algorithm used to make these inferences, especially if a company is using the third-party data to create user profiles.
So, what is a business to do? Increasingly, they’re turning to third-party experts to help them manage and analyze their data, in part because compliance with data privacy requirements is rapidly becoming too complex and cumbersome to do in-house. And there are versions of AI and ML that are more explainable than the traditional “black box,” thanks to the work of projects such as DARPA Explainable AI (XIA) and Local Interpretable Model-agnostic Explanations (LIME). But for the most part, organizations are in a “wait and see” mode until the courts clarify AI explainability requirements.
In the meantime, it’s critical that organizations set up policies to govern the proper use of consumer data with AI and ML, and make sure that they can, at the very least, identify where AI and ML are being used to make decisions about individuals and the data sources these algorithms are using. That’s not a simple task in many organizations because so many different functional units are taking advantage of AI and ML, including IT, marketing, product design, customer service and many more.
Everyone needs to be on the same page so that, when a decision is finally made on explainability, your organization will be prepared.