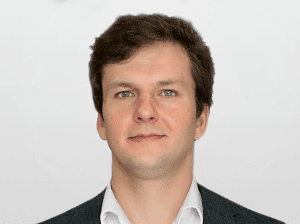
In the fast-evolving data landscape, understanding emerging trends and embracing technological advancements are key to staying ahead. As we approach 2024, this article explores the data trends that will define the strategic landscape for the coming year.
Trend: A Focus on Data Sharing and Data Collaboration
Improving data sharing and secure data collaboration between parties is becoming a key area. Companies like Snowflake and Databricks are embracing this idea, and it’s gaining traction across various industries.
Over the past decade, digital transformation has led to the breakdown of business processes and systems into smaller pieces. Some of those pieces remain within the company, while others are outsourced to external providers, creating a complex ecosystem. For example, global payment processing’s digital transformation efforts can now touch 10 or 15 companies, and data is spread across all these different parties. Data from multiple providers needs to be integrated in order to be viewed holistically, and this is a challenge.
So, data products are increasingly being built around the idea of merging data across different parties. This trend is expected to continue for the next few years, and many data products will be built around this process.
Trend: The Rise of Data Mesh
The concept of data mesh has gained traction over the last three years. It brings two key components to the forefront. First, it introduces the idea of “data as a product,” which involves packaging data in a well-defined, discoverable format that can be used in a self-service fashion, without direct involvement from the data producer. This concept includes not only raw data but also analytical models, such as those used for customer churn or fraud prevention.
Secondly, the use of self-service platforms for producing data products, not for business intelligence, enables various business units to create data products without the need for separate data platforms. This reduces costs and increases efficiency.
Major technology providers, including cloud services like Azure and AWS, are catching up and offering solutions to manage distributed data and analytics platforms in a data mesh fashion. This helps to connect data across various platforms and technologies, providing a centralized view of the data landscape.
Trend: LLMs Will Play a Crucial Role in Enhancing Data Engineering and Data Operations
Generative AI and large language models (LLM) have the potential to transform the data space. This transformation includes deploying GenAI models within existing data infrastructures for tasks like data engineering and data operations.
Even more interesting is the potential for these technologies to solve rudimentary tasks, such as profiling, modeling, and integrating data, streamlining processes, and improving Data Quality. LLMs are expected to play a crucial role in enhancing data engineering and data operations.
Trend: Companies Will Invest in Data Discovery Tools and Data Catalogs
Data Governance has evolved over the last few years. Previously, it was focused on securing data and managing risk, but it has since shifted to making data widely available while minimizing risks. The concept of data-as-a-product is the biggest change, because it shifts responsibility to the teams who are producing, owning, or serving the data.
Companies are investing in data discovery tools and data catalogs to gain visibility into their data, including its sources, ownership, structure, and quality. Data Governance now involves making data visible, discoverable, reusable, and useful.
Trend: Growing Emphasis on Data Quality
Data observability has gained popularity in the last two or three years, driven by the increased use of data analytics and the need for Data Quality. It offers a granular understanding of data at runtime, helping organizations track the flow of data and identify Data Quality issues, operational problems, and changes to data systems. It provides a lot of value to engineers and operational people in terms of visibility and an understanding of what’s going on.
Data observability tools like Monte Carlo and Soda have emerged to meet the growing demand for improved Data Quality and operational efficiency.
Another aspect of this trend is the increasing investment in data analytics. In the realm of data analytics, the value derived heavily depends on the quality of the data being analyzed. As a result, organizations are placing a greater emphasis on Data Quality. During this process, it becomes evident that many Data Quality issues do not stem from the absence of well-defined business rules or validation rules for the data. Instead, issues often originate from operational discrepancies, such as changes made by individuals or inaccuracies in data received from providers, among other operational challenges.
These are five of the most important data trends to be aware of in 2024. Which ones would you add to the list?