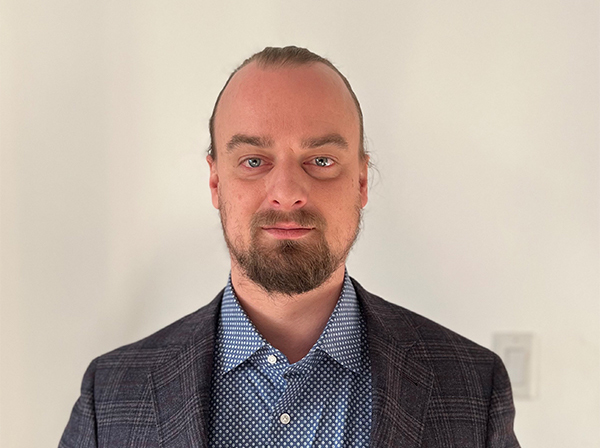
The demand for Data Science talent far exceeds the talent pool, making employee retention challenging. Demand is expected to remain high throughout 2022, providing ample opportunity for talent to move upwards through competing organizations. A vast amount of project familiarity and understanding is built on the backs of data scientists and is lost when they leave. In the midst of unprecedented industry opportunity and demand for data scientists, it is important for businesses to focus on retaining top talent in order to minimize transitional losses such as training costs, team efficiencies, and expertise.
According to the U.S Bureau of Labor Statistics, the expected job market growth rate for Data Science and related fields is 31%, far greater than the overall average job growth rate of 4%. Data scientists know this and are able todemand more for their skills. In 2021, the median salary for data scientists topped $100,000, exceeding that of engineers. As artificial intelligence (AI), machine learning (ML), and other emerging technologies are further adopted, demand for top talent will only increase. As a result, data scientists will have increased bargaining power to lobby for what they want, and employers will need to work even harder to retain Data Science talent.
One study found that like many other roles in the tech industry, job satisfaction for data scientists suffers a sharp decline after approximately two years. What causes this decline in satisfaction, and how do we solve it? Here are some clues.
1. Documentation is tedious and distracting.
As applications for machine learning advance and its impact broadens, increased public scrutiny and examples of irresponsible AI are catching the attention of policymakers. And as AI/ML matures, expectations are heightening around transparency, effectiveness, and ethics. These expectations create added pressure on Data Science teams and a critical need for documentation.
Rigorous documentation is now required throughout the AI model lifecycle to ensure models are transparent, unbiased, and safe. Documentation, while necessary for responsible AI, is repetitive and time-consuming. It often distracts from other work data scientists prefer to do. Fortunately, there is a solution: automation.
Automating documentation processes helps data scientists and ML teams recoup time to work on tasks they are truly passionate about. Technology solutions can help automate tasks and guide data scientists through documentation checkpoints that map to evolving policies and regulations. Adopting a solution that streamlines documentation is an effective way to empower data scientists to focus more on the work they most enjoy.
2. Delayed and abandoned projects are discouraging.
Eighty-seven percent of Data Science projects never make it into production. When data scientists put their all into a project that is later abandoned, it’s disappointing; they don’t get to experience the fruits of their labor, so to speak. Businesses may find that improving their model risk management process, and even involving data scientists and embedding controls during model development, will result in less unneeded work and fewer abandoned projects. It also provides greater opportunity for data scientists to take pride in their work and improve ROI on their efforts.
Even when models are put into production, the model-to-market process can sometimes be painfully slow. Examining where and why projects get held up, and then putting solutions in place to address delays and expedite processes can speed the satisfaction data scientists get from seeing their models in use.
3. Teams and workflows aren’t structured for seamless cooperation.
Moving AI models from development into production takes contributions from multiple groups. For example, data scientists and AI model developers need to work in cooperation with validators and quality assurance teams as models are ushered through compliance checks, tested for bias, etc. If teams and processes exist in separate silos, validation can be chaotic, clunky, and repetitive.
Bridging gaps between teams, improving communication and cooperation, and expediting transfers of information while maintaining strict control and segregation of duties is important for regulatory compliance – especially in the financial services industry. At the same time, data scientists need and want to be able to maintain their independence, and not be micromanaged. To satisfy all these needs, organizations should look to implement a model risk management solution that gives data scientists tools to record data and decisions, while still maintaining control over their work. Having a smart solution in place will help make cooperation and communication smoother.
4. Organizations are slow to adopt innovative technology.
There is a clear correlation between job satisfaction and access to state-of-the-art technology, supported by studies done by Deloitte. Data scientists are driven by and aware of new innovations that can help make their jobs easier and more engaging. However, many businesses have been slow to adopt technology solutions that could make life easier for them.
New technologies are readily available that offer solutions to common AI challenges. Organizations will have better luck retaining top talent if they support their data scientists and AI teams with smart tools and powerful technologies that assist with capturing evidence, simplifying documentation, streamlining reporting, identifying risks, checking for compliance, monitoring performance, and other key functions along the AI model lifecycle.
As the AI race continues, the demand for talent is growing rapidly. Successful businesses will not only compete to build the best AI, but also compete to create the best work environment for those developing it.