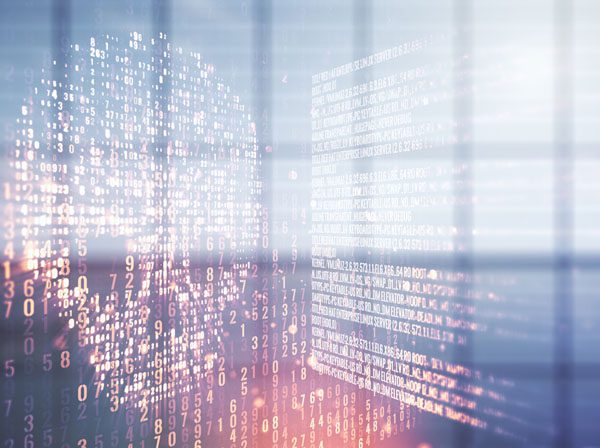
Combining ethics with Data Management and artificial intelligence can build an organization people will trust. Ethical behavior promotes the smooth functioning of human interactions, which includes business, and supports the overall community. AI has the potential to make ethical decisions and can be used to create a healthy relationship with the customer base.
Businesses can be separated broadly into two basic categories — those that rely on a steady flow of new customers and those striving to build a repeat customer base. For an internet business to achieve true, long-term success, it must apply basic ethical safeguards in collecting and using data. An organization’s long-term sustainability is based on the expectation of trust. For companies handling data, its ethical use has become a central feature in the design of all trust models.
People who have the ability to see reality from a big picture perspective are typically ethical. There are also people who seem to be ethical by nature, as though it is a part of their genetic structure. However, roughly 30 percent of the human population seems to be comfortable with varying degrees of unethical behavior (essentially, theft and deceit). They typically lack a sense of empathy, either through training or genetics. Generally speaking, unethical behavior damages individuals and the community as a whole, while rewarding an individual, or small group. (At least, in the short term).
Data Management and Ethics
The ethics of Data Management can be ambiguous and have the potential to limit short-term profits. Consequently, some companies in the past just simply avoided discussing the issue — empathy and ethical concerns were easy to block and ignore when dealing with the faceless customer base the internet provides. This lack of ethical behavior led to the enactment of laws (society’s effort to enforce ethics), as the general public becomes increasingly aware of how their personal data and behavior patterns can be manipulated.
In Europe, the lack of ethical behavior on the part of internet businesses was taken seriously and led to the creation of laws referred to as the General Data Protection Regulation(or the GDPR). These laws protect the rights of European Union citizens’ data privacy, and must be considered when doing business in the EU. From a Data Management perspective, these laws must be obeyed, or the business risks having to pay significant fines. (After their withdrawal from the EU, Britain passed its own version of the GDPR.)
In the United States, California has implemented similar laws (CCPA), but on a national scale there are no laws or regulations comparable to the GDPR. Most internet consumers in the U.S. do not have the same legal protections, and “ethical considerations” are ignored in the pursuit of profit. There are four hard questions that may help businesses when choosing how to include ethics in their Data Management program:
- Will the sale of this data allow individuals to be unknowingly manipulated?
- Is the data both honest and accurate?
- Are an individual’s rights being violated? (Who owns the data?)
- Is the data being used appropriately? (Sharing data about sexual behavior could benefit science, but may be considered unethical if it is about specific, named individuals.)
Data is simply a tool. By itself, it has no ethical implications. How it is used, on the other hand, does have ethical implications. What is done with data (or not done) raises ethical questions on how we collect data, how we protect it, and how we use it.
The Trust Factor
Trust is not a fixed, permanent feature. Though it may be given freely initially, it can be lost in an instant, and can then only be regained with time and a series of positive experiences. Trust is based on having expectations met — and honesty is an expectation when doing business within most industrialized countries. Honesty is the primary component of ethical behavior, communicating “reliable information,” which is quite useful overall, and also necessary for maintaining data integrity.
The classic example of deliberately distorting data to support a hidden agenda is a few bank employees, in positions of authority, who have secretly decided to include racial prejudice in the bank’s loan screening process. Unable by law to use race in making loan decisions, these bank employees create a program that screens out people based on the neighborhood they live in. From a big picture perspective, this kind of behavior skirts the law, distorts the integrity of the data, reduces potential profits, and damages the community by hindering the growth and improvements of people in specific neighborhoods. In other words, it’s unethical. (When discovered, this kind of distortion is often called a “mistake that is being corrected, immediately.”)
Another example comes from Google, and shows how algorithms can unintentionally shift to unethically “biased” behavior. In 2016, Google had to deal with one of its algorithms promoting prejudice. Google has an algorithm that predicts the question being asked, based on the popularity of the question. For example, in 2016, when a partial query about a minority group was typed into the search engine, the algorithm would present various endings to the query, with the first option a stereotyped response that led to a number of anti-minority sites, promoting prejudice in the process. Google fixed this specific issue immediately, but the example shows how easily accidental unethical behavior can take place by way of algorithms, if no one is paying attention.
How Bad It Can Get
Fake news is a well-known phenomenon now and will become even more difficult to detect, thanks to generative adversarial networks. Though still a fairly new technology, it is dangerous enough to raise some serious ethical concerns.
The process uses algorithmic architectures in building two neural networks. These two AI networks are then “pitted” against one another (“adversarially,” applying game theory techniques), with the goal of building illusions comparable to reality. A “generator network” builds a vector into an audio matrix or an image. The output is fed into what is called a “discriminator network,” which learns how to discern between real content and computer generated content. The two networks work and learn in tandem. As the generator network continues to learn and improve its techniques for tricking the discriminator network, the discriminator network develops better and better techniques for identifying artificially generated content.
This process produces results that look and sound like a recording of reality. It is, however, fake news. While these generative adversarial networks do have great potential for creating art and political humor, there is also the dangerous potential of using it to create fake news and advertisements. A single deceitful video could do significant damage to an organization’s, or a person’s, reputation.
Building Artificial Intelligence with a Code of Ethics
In 2017, Amazon’s CEO, Jeff Bezos, wrote a letter to shareholders, saying:
“Over the past decades computers have broadly automated tasks that programmers could describe with clear rules and algorithms. Modern machine learning techniques now allow us to do the same for tasks where describing the precise rules is much harder.”
While there are several ways humans can apply a standard of ethics to their collection and use of data, AI provides a novel, standardized method of including ethics in the handling of data. (It can also keep a business from breaking the law — GDPR and CCPA.)
Artificial intelligence/machine learning can provide strong governance mechanisms when collecting and processing data. The ethics used by the AI should reflect the ethical codes of the organization, which will involve discussing and establishing what those ethical codes are. If the organization is large enough, creating an ethics committee would be appropriate. Including someone opposed to ethics and favoring profits while using a “consensus-based” decision making model would be disastrous. Advertising and actually using a code of ethics does have the effect of gaining the trust of customers and the public.
While creating algorithms that support ethical decisions may sound simple enough, the issue of bias can surface, unintentionally. We all possess unconscious biases that can be magnified with machine learning. An awareness of how data impacts an ethical algorithm design can help to prevent biases from developing in an AI system, and regular monitoring can prevent it from accidentally developing and maintaining biases. At some point, the installation of ethics programs in artificial intelligence/machine learning may become a legal requirement — society’s effort to enforce ethics.
Image used under license from Shutterstock.com