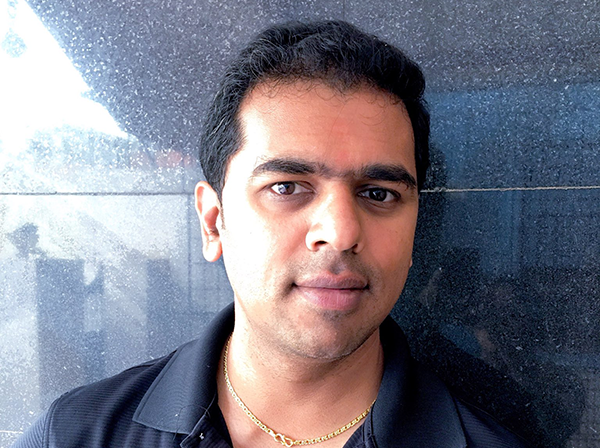
The recent hype surrounding AI makes every organization feel like they must rethink their strategy to ensure they are aligned with the market expectations and not let the competition gain an advantage. AI has been in the news for a while, but when ChatGPT was introduced, people outside of business started to explore the technology to the extent that it started to impact their lives directly. It also opened the door for people to be interested in exploring the possibilities of AI overall.
The key ingredient to a successful AI strategy is the data. The larger the training dataset is, the more accurate the model is expected to be. With data being generated from different data centers at the edge, and from the cloud, it is critical that the right data sets are used for training purposes and then deployed appropriately to get the best results.
This article explores the challenges associated with deploying AI solutions at the edge and the key measures needed to execute a successful AI strategy.
Why AI at the Edge?
The primary intent of having an edge solution is to deliver low latency because the solution is closer to the customer and can handle requests quickly. With AI solutions for various technologies such as autonomous vehicles, health care, IoT devices, personalized advertising, and others that deploy at the edge, there are multiple associated benefits:
- Cost optimization: Moving all the data to the cloud creates operational and cost pressure due to the time, money, and value involved. The higher the data volume, the more financial burden an organization incurs. Processing data at the edge relieves these burdens.
- Reliability: For organizations to provide low latency and a quicker response to requests, high-speed connectivity between various modules is a must. Sending all the data to the cloud is not a feasible option when there are constraints on network bandwidth and its effectiveness.
- Security: With the AI models at the edge, all the data is processed at the edge. This reduces concerns about potential security exposures during transmission to the cloud.
Challenges to Edge AI Solutions
As mentioned above, data is the biggest factor when it comes to building an effective AI-based solution. However, there are multiple challenges that organizations need to understand and overcome in order to implement an effective AI strategy with edge deployments.
Disparate Data: Organizations are most likely expected to collect data from various sources, and each edge center is expected to generate a tremendous amount of data. In this kind of scenario, data generated from multiple data centers is not expected to have a uniform pattern. In situations where the data being generated is disparate and there are missing patterns in data being generated from various edge data centers, the model complexity is very high. In this kind of situation, a single model for all edge deployments is not feasible.
Compute Power: From a hardware perspective, AI requires a lot of computing power. Running AI-based applications at the edge would require the following:
- Central Processing Unit (CPU) for processing the model
- Graphics Processing Unit (GPU) for when there are some massive and complex compute algorithms with deep learning
- Memory in high volumes to process AI applications
- Networking for when data or models need to be transmitted to multiple locations
- Storage needs to be local to deal with data
The hardware requirements for edge deployments in general also have a lot of constraints due to local regulations and legalities, so meeting the criteria for deploying AI-based models is a big challenge.
Regulations: For AI to perform effectively, the model needs to be trained with significant amounts of data. In the case of edge deployments, data can come from any geographical location. Unrestricted access to data is required for the model to perform effectively. However, with GDPR regulations and other international laws, the free flow of data is a huge challenge; therefore, building an effective model is not feasible when data from edge deployments has transport-related restrictions.
How to Implement an Effective AI Strategy for the Edge
An effective Data Management strategy that can handle all the challenges associated with edge deployments is essential when considering AI at the edge. The following needs to be taken into consideration:
- Initial data analysis needs to be performed and edge sites that produce similar data should be grouped together. This activity needs to be done so organizations can then look at potentially deploying similar models at these edge sites. This approach also would potentially reduce duplication efforts when building models.
- If the legal regulations allow for data to be sent to the cloud, training data should be sent to the cloud and models should be built there as well. After a model is successfully built, it can be deployed on the edge site. This will ensure edge sites are focused on processing critical data and appropriate usage of resources, and not on building models. The performance of the model should then be reviewed on a periodic basis and should be updated appropriately. However, if there are legal and regulation issues in place that do not allow for data to be sent into the cloud, offline processing of training data should be considered, and models should be appropriately built in the best location.
- With the recent advancements in the field of computing, GPU processors are now available for deployment at the edge. Wherever feasible, organizations should consider deploying for greater processing speed, specifically when edge sites handle videos, images, and other complex data.
- To mitigate concerns around limited network connectivity to the cloud, organizations should consider leveraging the 5G network. The 5G network has the capability to provide higher bandwidth, low latency, and connectivity to remote sites. This enables data sync-up and migration of models to happen at a quicker pace. This also allows organizations to consolidate results from edge sites and provide a comprehensive view to the appropriate stakeholders.
Summary
Cloud-based AI solutions offer many benefits. However, there are also limitations associated with data movement from various sources to the cloud, and apart from that, there are security and latency concerns. Due to these concerns, edge-based AI is an attractive option. Edge deployments are in a mature stage now, and with advancements in machine learning models and the proliferation of various smart systems – along with focused enhancements towards edge-based hardware – edge-based AI will continue to see expansion and adoption in the near future.