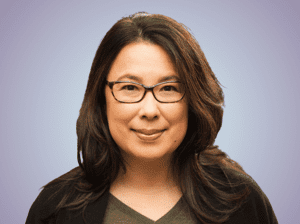
At a recent presentation for a local post-secondary institution, I fielded a number of questions related to the use of language, primarily English language texts, as training data for generative AI. There were questions around cultural impacts and related ethical concerns. These queries were more nuanced than the usual ones I get around copyright or data reuse, which we tackled in last month’s column. So, I did a little research to help better understand:
What happens when language becomes AI training data and what are some of the concerns that arise from this process?
We Are Creatures of Language
In one of those down-the-rabbit-hole internet journeys that we all take from time to time, I landed on an old blog by author and activist, Tom Athanasiou. He was talking about AI pioneer Terry Winograd, who received a letter from an unnamed author, that said:
“From my point of view natural language processing is unethical, for one main reason. It plays on the central position which language holds in human behavior. I suggest that the deep involvement Wiezenbaum found some people have with ELIZA [a program which imitates a Rogerian therapist] is due to the intensity with which most people react to language in any form. When a person receives a linguistic utterance in any form, the person reacts much as a dog reacts to an odor. We are creatures of language.”
The writer goes on to say that they consider churning out machine generated text akin to a criminal act – that is how seriously they felt we should take the issue of processing language texts!
While my own reaction to natural language processing (NLP) or the sub-field of generative AI is not quite as dramatic, I do think the author makes an interesting point. Language does hold a special place in human understanding, shaping behavior and crafting our cultural identity. It’s the means by which we relate to one another.
We tend to react to language in ways that play deeply on our psyche. This, in part, explains why there’s been such a popular and widespread reaction to chatbots that generate text – which, from a purely technical AI research point of view, isn’t all that innovative. It might also explain why people who use the tools the most seem to become the most enamored with them, even coming to believe that they might be sentient.
What happens when we use language, not as a communicative medium for humans, but as training data for an AI system? Before we dig into this question, it’s helpful to clarify what we mean by data.
What Is Data?
As a data ethicist, I push back on definitions of data as “facts” because many things that are data are not factual (such as inferences for starters). Instead, I think about Rob Kitchin’s definition of data as a representation of a phenomenon.
We can (and do!) turn a lot of things into data and data has some unique characteristics that make it useful. Philosopher C. Thi Nguyen describes data’s power as a function of its universality and portability, as something we can measure, collect, and exchange. This comes at the expense of other things, such as context and the devaluation of concepts that don’t fit neatly into being measured, collected, and exchanged. These are the limits of data as Nguyen explains:
“We gain portability and aggregability at the price of context-sensitivity and nuance. What’s missing from data? Data is designed to be usable and comprehensible by very different people from very different contexts and backgrounds. So data collection procedures tend to filter out highly context-based understanding.”
Yet, language is highly context-based and nuanced. Think about how a word’s use changes over time, or how the use of irony or a play on words changes meaning. Think of the inside joke that only you and your best friend “get” because you had to be there. Our lives are filled with examples of how language relies on context or shared social understanding. Language is the opposite of data.
From Tolkien to Token: Turning Language into Data
“Not all those who wander are lost.” – JRR Tolkien
Natural language processing involves turning language into formats a machine can understand (numbers), before turning it back into our desired human output (text, code, etc). One of the first steps in the process of “datafying” language is to break it down into tokens. Tokens are typically a single word, at least in English – more on that in a minute.
For example, our Tolkien sentence would tokenize as:
“Not” “all” “those” “who” “wander” “are” “lost” (7 tokens, 1 token per word)
There are various tools for tokenization and these might break down longer words slightly differently.
Tokens are important because they not only drive performance of the model they also drive training costs. AI companies charge developers by the token. English tends to be the most token-efficient language, making it economically advantageous to train on English language “data” versus, say, Burmese. This blog post by data scientist Yennie Jun goes into further details about how the process works in a very accessible way, and this tool she built allows you to select different languages along with different tokenizers to see exactly how many tokens are needed for each of the languages selected.
NLP training techniques used in LLMs privilege the English language when it turns it into data for training, and penalize other languages, particularly low-resource languages. Even when a prompt and requested output is given in another language, researchers have learned that the hidden layers of a model seem to be working conceptually in English. There’s also the obvious point – there’s more English language text on the internet to use as training data. Privilege begets more privilege.
Delving Deeper
We’re also learning about odd, unintended consequences, arising from the training process, such as the way large language models overuse certain words like “delve.” This appears to be the result of outsourcing data-related tasks to particular geographic regions, a practice that has ethical supply chain issues in addition to material impacts on the outputs. A recent article in the Guardian explains that:
“In Nigeria, ‘delve’ is much more frequently used in business English than it is in England or the US. So the workers training their systems provided examples of input and output that used the same language, eventually ending up with an AI system that writes slightly like an African.”
We’re still discovering the idiosyncrasies of text from ChatGPT, from its overuse of certain words, flowery phrasings, and a tendency to end with a literal conclusion. Identifying these quirks is one thing, but understanding how to best respond is another. I don’t think it’s about stripping the word “delve” out of everything, but now when I see the word “delve,” I can’t help but wonder … is that bot writing?
In Conclusion 😉
Some researchers are working to try and diversify the language inputs for training data. That’s not a bad thing, but it might have limited uptake for the industry if it means increasing the cost of already expensive training. Is there another way to train these models that would fundamentally rethink the idea of tokenization and the range of tools built to facilitate that process? Perhaps that idea is not impossible, but it feels rather unlikely. We’ve been going in this direction with NLP for decades. These are structural forces that reinforce English language hegemony through the products that are developed.
Circling back to the bigger issue of what happens when we encounter language, regardless of how it’s created, how can we use generative AI tools in ways that are “healthy”? Is that possible and what does that look like? Winograd’s friend would say no. It’s not possible. Don’t go there, because when you do, you’re at risk of being conned, of being taken in by a large language mentalist.
Should we drink from the (possibly) poisoned chalice? Right now, I’m cautiously sipping.
NLP Fun Fact: Here in Canada, our bilingual heritage helped us survive an AI winter in the 1970s when the government kept funding flowing for METEO, a system that helped translate weather forecasts into both official languages.
Send Me Your Questions!
I would love to hear about your data dilemmas or AI ethics questions and quandaries. You can send me a note at hello@ethicallyalignedai.com or connect with me on LinkedIn. I will keep all inquiries confidential and remove any potentially sensitive information – so please feel free to keep things high level and anonymous as well.
This column is not legal advice. The information provided is strictly for educational purposes. AI and data regulation is an evolving area and anyone with specific questions should seek advice from a legal professional.