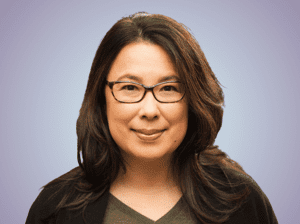
It’s easier than ever to use AI-generated images or text and to create synthetic data for use in research. A recent high-profile story got me thinking more about this question:
When is it OK to use “fake” data?
Before we dive into this, I’m putting “fake” in quotes because I’m taking a wide perspective on this topic of what might constitute “fake” data. I’m including times when the content might be of an actual person in whole or in part, but the manner in which they are depicted is not forthright or honest. I’m also going to look at entirely made-up data including synthetic data.
Deepfakes and Misrepresentation
Let’s start with the obvious – it’s clearly unethical and, in some cases, also illegal to create or use deepfake content to cause harm. This could be non-consensual intimate images, or using a deepfake to commit fraud. There are numerous examples, and there are currently not enough protections in place for people who are victimized.
But, this is not just a column about deepfakes.
The story that caught my attention involved a business owner who was recently removed from the Toronto Police Services Board following a CBC investigation about the use of “fake” images of supposed employees on their company website. Two of the images appeared to be of actual people being misrepresented as employees, while the third image did not appear to be of an actual person. While City of Toronto officials were tight-lipped about the details of the case, they clearly felt that some breach of conduct warranted what is being called an “unprecedented” step to remove this person from the board.
The use of “fake” photos being positioned as employees of a company is not a new practice. There have been other investigative reports on similar issues. I’ve also seen some of this first hand. Someone contacted me last year saying they were launching an AI ethics company and wanted to have a chat. When I went to their website (which no longer exists) there were a slew of “employee” images – all of which looked highly suspect. It raised many red flags, but with AI-generated images, it’s a lot harder to tell who is real or who is not. However, it was enough for me to disengage with this person, whose identity I was beginning to question at this point.
What makes these stories relevant for this moment is just how easily this could be done using generative AI and how difficult it is to effectively fact-check whether or not a person depicted in an image is real or fake. Depending on how much of a backstory someone wanted to create, they could make things appear legitimate. There’s also an irony to the story of the Toronto Police because police do background checks all the time. Of course, those kinds of checks are not necessarily designed to catch this kind of issue.
Context Is Key
Context is an important factor when it comes to determining the legitimacy of using an image. Stock photos are a long-time staple in marketing campaigns, including company websites. This is an acceptable practice (with proper licensing, of course!). Nobody thinks these are your real customers, partners, or employees when they are used as background or design elements. AI-generated images used instead of stock photos to add background or design elements are also fine to use in this manner. You may wish to also label that the image is AI generated for further clarity and transparency.
What crosses a line is labeling the image in such a way that suggests they are an actual employee, customer, or partner when they are not. The issue is not the image, the issue is deception. There are laws against deceptive business practices, in addition to the clear ethical violation.
But What if My “Employee” Is an AI Agent?
Let’s flip this situation around: What if your actual senior executive is really a bot? There are more companies that are selling AI agents to perform particular tasks. One that I’ve been looking into is Hippocratic AI.
Hippocratic’s business model is to provide healthcare AI agents that can perform a particular task such as following up with a discharged patient. The human-like image (which I’m assuming is AI generated) and human names attached to the agent give an impression of humanized care. However, the website copy makes it clear – they are in the bot business.
Transparency is a core principle in AI ethics. Ensuring people know they are interacting with an AI bot helps protect against deception and upholds human agency. If a healthcare service provider is deploying a healthcare AI agent, it should be very clear this is not a real person.
Going back to our original example, the reason why companies might use “fake” employee images is to bolster their profile. It’s an effort to make the organization seem bigger or to give it some air of credibility. Yet, as we move into a world where AI agents might be doing the work previously done by employees, even senior ones, maybe this kind of deception goes away because it doesn’t achieve its intentions (and to be clear – it was never a good idea!). AI agents also raise a whole bunch of other ethical issues, which we’ll have to save for another time.
Fake Research Data
While we’re on this topic of “fake” data, the book “Complicit: How We Enable the Unethical and How to Stop” by Max Bazerman is a relevant read. Bazerman outlines his own complicity in a research scandal outed by the team at Data Colada. The quick version: Bazerman, who is an ethics expert, is listed as a co-author on a paper where the underlying data and its distribution were horribly off. Data Colada called the data “impossibly similar” to the point there was evidence of fraud. As this article points out:
“To determine the likelihood that these sets would be so similar, Data Colada ran one million simulations in an attempt to replicate the similarity. “Under the most generous assumptions imaginable, it didn’t happen once,” the article stated. “These data are not just excessively similar. They are impossibly similar.” –Big Think
The research study was a big deal – it led to high-profile careers for those involved, even as the work itself was not valid. Even more ironically, the research in question was about honesty!
The book explores not only Bazerman’s own complicity in this matter but also the bigger idea of complicity. It’s a great reminder that for every Bernie Madoff, there is also a team of people willing to look the other way, gloss over inconsistencies, or even actively cover things up. We might also think about the ways that data can cover things up, the ways we might manipulate it to gloss over inconsistencies, or perhaps even the ways we can just make it up to suit our goals.
Synthetic Data
Clearly making up research data is wrong – or is it? Once again, AI tends to complicate what seemed to be a clear-cut matter. Since machine learning is data intensive and since it can be complicated to get access to certain kinds of data, some researchers are choosing to make their own data. Synthetic data is constructed to resemble the underlying representation of real-world data. It’s become popular, particularly for healthcare and life sciences research. Some say it’s the answer to ethical issues such as privacy. In my experience, those are usually people who have some vested interest in a company marketing synthetic data.
Synthetic data raises many new ethical questions. Who gets to justify why using synthetic data is appropriate in a particular context? Who determines how it will be created and what might the implications be if it does not accurately represent real-world data? What harms or risks might ensue? There are many more questions – this paper Getting Real About Synthetic Data Ethics is a wonderful resource that goes into much more depth.
Send Me Your Questions!
I would love to hear about your data dilemmas or AI ethics questions and quandaries. You can send me a note at hello@ethicallyalignedai.com or connect with me on LinkedIn. I will keep all inquiries confidential and remove any potentially sensitive information – so please feel free to keep things high level and anonymous as well.
This column is not legal advice. The information provided is strictly for educational purposes. AI and data regulation is an evolving area and anyone with specific questions should seek advice from a legal professional.