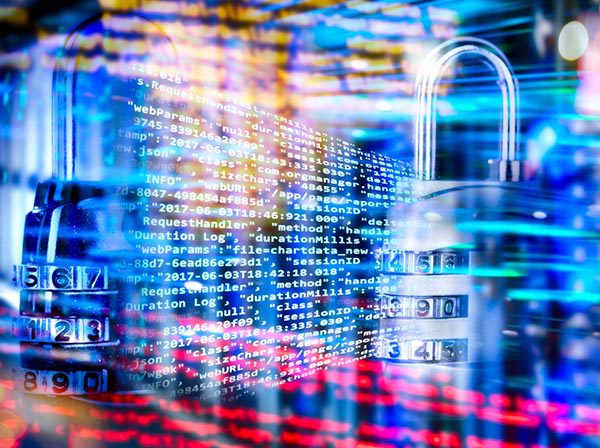
Sutton Bank is an FDIC-regulated, Ohio state-chartered bank. They have been in the payment business about 20 years, specifically in the prepaid space. Jeff Lewis, Senior Vice President of Payments and Prepaid Card Services at Sutton Bank, says that payment and card services are an increasingly popular offering for financial institutions, provided they can keep up with the steep data storage and analysis requirements.
Historically, smaller regional banks have been unable to compete in these card markets because of the high costs of hardware, software, and staff. With the growing availability of open source software and the emergence of integrated Big Data Analytics platforms, those barriers are lowering, Lewis said. This “technology shift,” as he calls it, has caused massive growth in the market.
Technology Shift Creates Opportunity
When it first entered the market, Sutton Bank used a complicated process that relied on a third-party vendor for the data warehousing, formatting, and analysis needed to add new customers, spot fraudulent transactions, and meet the bank’s regulatory requirements. This process kept their card business from scaling to meet demand. As an institution that provides access to the federal reserve system and other national networks, Sutton Bank also needed to move from their traditional spreadsheets, reports, and other paper-based items to a more efficient way to provide oversight.
“We had some programs coming on that were growing at incredible rate of new card accounts per month,” Lewis said, and this massive growth meant that they needed a solution that could offer a short implementation period and a quick time-to-value. Between their use of manual processes for reporting data and reliance on external vendors, the bank was spending more time “wrestling with data” than they were servicing existing clients and landing new ones, he said.
Needs Assessment
Sutton initially identified three areas where they wanted to see improvement:
- Increasing the speed at which new client data was added to the system
- Improved fraud reporting and regulatory compliance
- Greater flexibility and expanded choices for data end-users
Because Lewis had previously worked with Adwait Joshi, founder and CEO of DataSeers, he invited Joshi to a strategy session at the bank. “We talked about what we were doing from a growth perspective and how we wanted that growth to be profitable as well as sustainable from a regulatory standpoint,” said Lewis.
The regulatory environment for banks has a robust set of required rules for oversight, and compliance is complicated when working with disparate types of data from multiple processing platforms. In the payments space, Joshi said, it’s all structured data, so from a data perspective that should be a very simple problem to solve, yet it becomes one of the most complex problems to solve when that data is not used in the right way.
“Because only those things that make sense to the human psyche are what people pay attention to,” useful data goes unnoticed or unused, he said. “We take the approach that data is the lifeblood of an organization, and banks and credit unions are sitting on a lot of data.” Sutton Bank is unusual in its commitment to using updated technology to produce competitive insights, he said.
And the cost of using outdated technology can be significant. More than a billion dollars in fines have been paid so far because companies aren’t able to catch fraud patterns in the data by using manual detection processes. Yet Joshi says that everything that is needed is available in some shape or form somewhere in the company’s data — it’s just not readily accessible to the user.
Finding a Solution
Speed of implementation was an important consideration in their search for a solution, and they discovered that the existing systems on the market were complex and time-consuming to implement — involving months, if not years, he said. “Then you spend an exorbitant amount of money attempting to get the rules right and get your people trained. So, it isn’t an ‘install the system-have it up and running-learn-change’ situation.”
With Sutton Bank’s growth, a manual system that relied heavily on increased staff resources was not possible. Ideally, they wanted a way to use technology to assist with fraud detection, regulatory compliance, and to produce useful insights without a long implementation trajectory.
Machine Learning
Joshi said there are differences in how transactions are handled, depending on whether or not there are specific regulatory rules that apply. A transaction that exceeds a certain amount in ACH for cash deposits, for example, requires a report to Financial Crimes Enforcement Network (FinCEN). “That’s rules-based. There is no intelligence required.”
But there are a variety of issues that go beyond rules, he said. When some users are prevented from withdrawing more than $500 from an ATM, they will attempt to find other ways to exceed that limit, perhaps by going to a store and attempting to get cash back. “So, there are patterns people exhibit where you can’t rely solely on the traditional rules. You have to use algorithms to find unusual activity patterns.”
With typical money laundering, where overall there is too much money coming in and too much money going out, there is no set threshold. If several $10 credit and debit transactions occur in a day, that’s not a problem, but if thousands of $10 credit and debit transactions are occurring every second in the day, the volume indicates that something might be going on, he said. Machine learning offers certain algorithms and behavior-based metrics to identify when there is a potential problem.
Although the terms “machine learning” and “artificial intelligence” are often used interchangeably, Joshi stresses that he draws a clear line between the two:
“When you talk about AI, you’re talking about deep learning and automated unsupervised learning, which exist today in image processing and facial recognition. They don’t really exist in the mathematical space that payments are in.”
Transaction-monitoring algorithms require training, which comes under the category of supervised machine learning, he said. Over time, the algorithm learns from what it is told, and its accuracy improves. Because human behaviors and patterns change over time, machine learning is truly the only way to keep up, he said.
Rather than compromise on any of their priorities, Joshi and Lewis came to the conclusion that the only way to meet their needs was for Joshi to build a custom solution using machine learning technology. That custom solution became FinanSeer.
Program Development/Steps Taken
According to the company’s website, FinanSeer is a fast, redundant, and secure Big Data Appliance with a built-in visualization engine. DataSeers created a data lake where the bank can aggregate all of their data repositories formerly separated in silos, and where the velocity, the variety, and the volume can be easily handled, Joshi said. “Our approach was that we can use that data and make sense of it, so we created a solution that users can trust, because veracity is a big issue.”
Lewis said that the platform integrates open-source software from HPCC Systems to provide data integration and analysis capabilities. It is also supported by a worldwide community of developers, and DataSeers works with that team closely to fine-tune the platform’s performance and develop new applications to better leverage data, all without the costly per seat licenses and support contracts required by proprietary Big Data solutions. “For Sutton Bank, this allowed us to support a larger customer base, letting us compete with the major national banks,” he said. “Our whole concept is to move data from being an expense to an asset.” said Joshi.
Results
Their need for a rapid time-to-value was met: The bank engaged DataSeers, and within four months, they were able to identify what was wrong with their data, and demonstrate their oversight process. Lewis said they showed which partner accounts weren’t meeting criteria, while still adding an exponential number of new card accounts a month. “Our success was amazing in what the bank could show and see to the regulatory bodies. They were amazed at the data we have and the information the bank can pull for them at the drop of a hat.”
Previously, the bank estimated that one employee could handle 500,000 end-user accounts. With FinanSeer, each employee is able to handle five million accounts. Their response time to solving problems flagged by the FinanSeer platform has improved so greatly that they are now spotting and fixing problems with incoming transactions before their customers do. “That wasn’t possible before we started using the FinanSeer appliance, and it’s a key competitive differentiator for us.”
When working with partners, the bank has reduced the usual 12- to 18-month implementation cycle to about ten days. “That’s really key for us as a bank — we don’t want to be the bottleneck. So that was another important part of our strategy.” Partner compliance audits, which formerly took weeks or months under their manual system, are now automated, and Sutton Bank can analyze and warehouse all of a customer’s data in one night, allowing them to spot compliance issues and fraudulent transactions in near real-time before they become too costly.
In the past, it routinely took them two months to get a new customer data stream fully onboarded. Now, he said, the bank can do it in seven days, putting them in a much better position to attract new clients. In the three years since implementation, Lewis said they’ve grown substantially: approximately 150 percent last year and they are on track for 150 percent growth this year. Despite the booming growth, the bank has kept up with this rapidly expanding data set without the need for additional IT staff. “It truly is the speed of change that we can implement,” he said.
Next Steps
Joshi sees future potential for FinanSeer to have an impact on the regulatory process, saving time and staff resources. “If everything is working perfectly, then the regulators don’t have to come in once a year and check on you.” He envisions a report that goes out at regular intervalsthattells the regulator all they need to know about the health and compliance of the bank.“Our ultimate dream is for banks to keep on growing, innovating on the payment side, and we keep on handling everything on the data side to keep them compliant.”
On the horizon for Sutton Bank, Lewis expects to see continued growth and expansion along with educating and getting staff more and more comfortable with machine learning, as well as bringing more of their legacy systems into the data lake environment.
Conclusion
“We as a bank have gained a reputation for being open to innovation,” Lewis said, so companies can focus on growing their product line without having to worry about the technology. “They’re saying, ‘Wow, this is better; this is faster.’” The bank now has the means to quickly respond to partner needs, and at the same time, make sure the proper controls are in place with DataSeers to protect itself. “And with payments, that speed is what this world is all about right now.”
Image used under license from Shutterstock.com