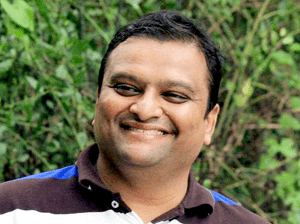
Click to learn more about author Chirag Shivalker.
Today there is harsh competition in the market for companies to grow and even to survive. Data is the most important factor now for organizations and is being seen as the cause of all successful or bad decisions. Data is rightly said to be the factor which enables businesses to make confident business decisions and gain actionable insights. Clean, accurate, validated, and standardized data is what forms the backbone of any strong and successful company. Such data also helps in delivering a superior customer experience, gain a competitive advantage, and make profitable growth.
Dirty Data – Game of Garbage
Research suggests that on an average, companies across the globe feel that 26% of their data is dirty. This contributes to enormous losses. In fact, bad data costs the average business 15% to 25% of revenue, and in the US economy over $3 trillion annually. Dirty data is what makes things more complicated in this rapidly changing business environment. It is because of this that companies make wrong decisions resulting in poor customer satisfaction and a great amount of money and energy getting wasted.
Without a standard process to start and keep data clean, bad or dirty data problems are bound to happen. Operational productivity is lost as the users waste their valuable time in checking and confirming the accuracy and reliability of data on hand. The effectiveness of data scientists is wasted as they are mostly occupied in cleaning, normalizing, and organizing data.
Data Cleansing – A Savior
Data cleansing is a process used to determine inaccurate, incomplete or obsolete information, and then improve quality by correcting unusable data, duplicates, and omissions. The process can include checking the format, completeness, consistency, limits, analyzing the data to identify faults (address, statistics, emails, etc.) or other errors, and evaluating the data. Verification before validation ensures compliance with the standards and rules. Listed below are some of the top benefits of data cleansing for a company:
- Enhanced decision making
- Improves operational efficiency
- Increases customer acquisition
- Increases revenue
- Streamlines business process
- Increases employee productivity
- Minimize waste of time and money
- Increases market credibility
- Enhanced market competitiveness
How to Cleanse Data?
A data cleansing strategy should be backed with rule-based best practices. It may be cleaning up the source data or cleansing the already existing datasets;but in both the scenarios there is a sequence of processes which is to be followed:
- Be specific about data integrity rules and data cleaning rules. Integrity rules refer to how the data must comply with respected business rules. Cleansing rules combine the definition of the integrity rule with the action to be taken in the event of a violation.
- Use data models to develop robust and complete set of data
cleansing rules for:
- Segmentation
- Data audit
- Filter the data
- Correct (enrich or delete) the data
- Improve the data sources
- Validate the rules and make them prerequisites for each existing source and for those to come in order to avoid having a database of re-polluting.
- Once defined and validated, the rules are integrated into the feeding process.
What are Data Cleansing Best Practices?
Here we have some data cleansing best practices known for giving better results in terms of data accuracy and time consumed to clean data sets:
- Implement an overall strategy for data cleansing.
- Create standards for how data is initially captured.
- Validate the data to make sure that it meets the required standards.
- Append missing data.
- Streamline the process through automation.
Conclusion – Adopt Automation and Technology for Efficient Data Cleansing
Looking at the dependability on data; it is critical for businesses to not to ignore the importance of data cleansing. Though repetitive and time consuming, data cleansing process needs to be dealt with aggression. Managing it through in-house teams becomes a big and hectic work for companies and organizations. Data cleansing professionals, expert at leveraging tools and technology, walk in to the picture. Digitization and automation of data cleansing processes has increased data accuracy, ease of use of that data and result in overall increased efficiency and productivity.
Robotic Process Automation (RPA) backed with Machine learning (ML) and Artificial Intelligence create sophisticated data cleansing handling solutions. RPA provides a record of the transformations it undertakes. Process optimization, regulatory compliance and maintaining transparency in a complex data environment is taken care of to help you make profitable growth.