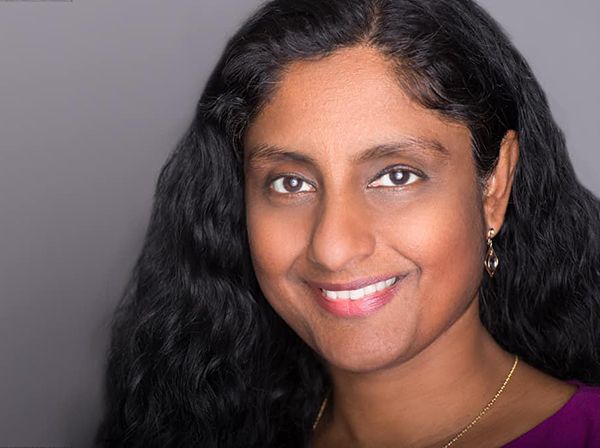
AI-based business models and products that use generative AI (GenAI) are proliferating across a wide range of industries. The current wave of AI is creating new ways of working, and research suggests that business leaders feel optimistic about the potential for measurable productivity and customer service improvements, as well as transformations in the way that products and services are created and distributed.
Most (90%) of enterprises allow some level of AI adoption by employees, according to my company’s 2023 Unstructured Data Management Report. In the same vein, the Salesforce State of IT report found that 86% of IT leaders believe generative AI will have a prominent role in their organization soon.
Yet, there are many potential hazards inherent in this new form of AI, from privacy and security risks to ethics concerns, inaccuracy, data bias, and malicious actors. Government and business leaders are analyzing the issues and weighing solutions for safely and successfully adopting AI.
This article reviews the latest research on AI as it pertains to unstructured data management and enterprise IT plans.
Highlights:
- Today, generative AI is a top business and technology strategy but also a leading priority for data storage managers.
- Though generative AI has much potential, it also presents a host of Data Governance concerns around privacy, security, and ethics, which is hampering adoption.
- Enterprises are allowing the use of generative AI, but they are often imposing guardrails governing the applications and data that employees can use.
- Most organizations are pursuing a multi-pronged approach, encompassing storage, data management, and security tools, to protect against generative AI risks.
Leading Enterprise Concerns About Generative AI
The concerns and risks associated with generative AI threaten to undo many of the technology’s benefits and to harm companies, their employees, and their customers. Violation of privacy and security is IT leaders’ top concern for corporate AI use (28%), followed by lack of data source transparency and risks from inaccurate or biased data (21%), according to my company’s survey.
Other research reveals additional concerns:
- The top three risks of generative AI, according to executives surveyed by KPMG, are cybersecurity, privacy concerns with personal data, and liability.
- Primary concerns cited in a recent Harris Poll were quality and control (51%), safety and security risks (49%), limiting human innovation (39%), and human error due to lack of understanding of how to use the tool and accidental breaches of organizational data (38%).
- 64% of IT leaders surveyed by Salesforce are concerned about the ethics of generative AI.
- About half (49%) of respondents in an IDC white paper noted concerns about releasing their organization’s proprietary content into the large language models of generative AI technology providers.
Let’s dig a little deeper into these areas of concern. Privacy and security is the most obvious one. Without guardrails on data use, employees may unwittingly share sensitive corporate data such as IP, trademark secrets, product roadmaps, proprietary images, and customer data hidden within files they feed to an AI tool.
A generative AI tool’s language learning model (LLM) would then contain that sensitive data, which could later find its way into works commissioned by others using the same tool. That data could even make its way into the public domain and remain there indefinitely. Newer AI features, like “shared links” of conversations generated by the tools, make it even easier to inadvertently disclose sensitive information if the link gets into the wrong hands. Conversely, a company may face liability if an employee creates a derivate work in AI containing protected data leaked from another organization.
Another top issue is the potential for inaccurate or harmful outcomes if data in the model is biased, libelous, or unverified. There has also been a spate of lawsuits by artists and writers concerning use of their works in training models.
Organizations may unwittingly be liable for a variety of potential claims when using general AI training models. This can lead to long-term damage to a company’s customer relationships, brand reputation, and revenue streams. Accordingly, KPMG’s research found that 45% of executives thought that AI could have a negative impact on organizational trust if the appropriate risk management tools were not implemented.
Preparing for AI
As commercial AI technologies rapidly evolve, IT organizations are thinking about and deploying AI strategies and policies. Preparing for AI is, in fact, the leading data storage priority of IT leaders in 2023, compared with a primary focus on cloud migrations in 2022, according to my company’s survey. Only 26% of IT leaders said they have no policy in place to govern AI, and only 21% allow AI with no restrictions on the data or applications that employees can use.
AI preparations may include the following investments and strategies:
Select the right tool: Major cloud providers, along with prominent enterprise software vendors, are all unleashing their own flavor of generative AI-related solutions to meet different use cases and business requirements. Take time to understand your organization’s objectives and risk profile. Part of the selection process involves determining whether you will use a general purpose pretrained AI model, like ChatGPT or Google Baird, or create a custom model. This blog post details the two different approaches. An organization with strict security and compliance requirements may choose the custom development approach, yet this will require hefty investments in technology and expertise.
Invest in AI-ready storage infrastructure: Running generative AI applications requires a lot of horsepower. An AI computing stack typically consists of high-performance computing capacity (CPUs and GPUs), efficient flash storage from companies such as Vast and Pure Storage, and appropriate security systems to protect any sensitive IP data used in the LLM. Top cloud providers AWS, Azure, and Google have released several new services to run generative AI projects and lessen the cost, energy usage, and complexity for IT organizations.
Consider the data management implications: There are five key areas to consider for using unstructured data management in AI tools, spanning security, privacy, lineage, ownership, and governance of unstructured data, or SPLOG. Consideration begins by gaining thorough visibility into file and object data across on-premises, edge, and cloud storage. Tactics include:
- Segregate sensitive and proprietary data into a private, secure domain that restricts sharing with commercial AI applications.
- Maintain an audit trail of who has fed what corporate data into AI applications.
- Understand what guarantees, if any, your vendors will make about the use of your data in their AI algorithms. This goes beyond AI vendors, since other enterprise software applications are now incorporating AI into their platforms.
- Ask AI vendors to share information on the sources of data curated for the LLM and how they will protect your organization against any harmful outcomes or liabilities related to the training model.
Forty percent of IT leaders in my company’s survey say they will pursue a multi-pronged approach encompassing storage, data management, and security tools in order to adequately protect against generative AI risks. Related findings include: 35% will work with their existing security/governance vendors to mitigate risk; 32% say they have risk mitigation capabilities in their data storage and/or unstructured data management solutions; 31% have created an internal task force to develop and execute a strategy; and 26% will only work with an AI vendor that has adequate protections and controls.
Beyond technology, IT and business leaders should invest in training and educating employees on how to properly and safely use AI technologies to meet company objectives and prevent the host of privacy, security, ethics, and inaccuracy issues that can arise. Despite a 20-fold increase in roles demanding AI skills, only 13% of workers have been offered any AI training by their employers in the last year, according to a survey commissioned by Randstad.
2023 will go down as AI’s year of transformation from an experimental notion to a strategic priority for most enterprises, with budgets adjusting accordingly. How IT and business leaders implement AI from a Data Governance and risk management perspective will dictate whether this will be an overall positive development for humankind or not.