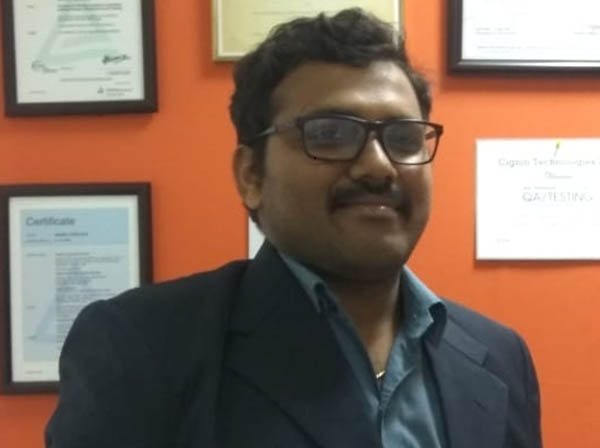
In the rush to adopt AI across diverse sectors, today’s enterprises face a common hurdle: efficient data labeling at scale. Numerous enterprises are grappling with generating usable data despite having huge amounts of raw information. Organizations are overwhelmed by the influx of image data, highlighting the need to process and label it for practical use. Data labeling is a crucial step in the model training pipeline. Choosing an efficient data labeling tool can directly impact the performance of machine learning (ML) models.
Accurate labeling and annotation are crucial for reliable ML systems, but applying complex ontologies consumes up to 80% of AI project time. Data scarcity and quality issues remain major obstacles for 19% of businesses and indicate the critical role precise data labeling plays in successful AI projects.
Challenges in Data Labeling That Enterprises Need to Overcome
Navigating the complexities of annotating diverse datasets presents a myriad of challenges. Identifying the roots of these annotation challenges is the first and most important step to solving them and improving the success rates of AI projects.
Data Diversity and Complexity
The diverse and complex nature of datasets presents a significant challenge in data labeling. Images, videos, text, and even sensor data each require unique approaches to labeling. Furthermore, lighting conditions within every data type vary along with perspectives and object occlusions, introducing further layers of complexity. Traditional labeling tools often struggle to handle this diversity, leading to inaccuracies and inefficiencies.
Scalability and Volume
The growth in dataset size amplifies the importance of data labeling’s scalability. However, manual annotation of vast datasets proves time-consuming and susceptible to errors. Automation becomes indispensable to match the escalating need for labeled data. Designing a scalable and accurate data labeling pipeline poses a substantial obstacle, particularly for real-world unstructured data.
Subjectivity and Ambiguity
Labeling subjective or ambiguous data may introduce another level of difficulty. For example, identifying subtle features in images and sentiment analysis in texts can be difficult due to the inherent subjectivity involved. To address these challenges, you need to develop a standardized guideline and ensure consistency among annotators.
Data Privacy Compliance
Organizations confront an escalating challenge amidst the growing accumulation of data in the face of proliferating regulations such as GDPR, DPA, and CCPA that emphasize data confidentiality compliance. They must carefully label unstructured data, often containing personal information like faces or license plates within images.
The principles of GDPR bind enterprises, thereby necessitating lawful, fair, and transparent data processing. To prevent unauthorized handling of sensitive data, organizations must implement stringent security measures, including on-premises storage and restricted device access.
Compliance complexities can arise, particularly about outsourcing data labeling services to third-party providers. Navigating these regulations becomes an imperative for organizations. They must ensure a secure and compliant process with the help of an efficient data annotation platform.
Solutions to Overcome Data Labeling Challenges
Leveraging advanced algorithms and crowdsourcing, along with active learning and clear guidelines, can act as effective solutions to conquer data labeling challenges with precision and efficiency.
Advanced Algorithm Solutions
Using sophisticated algorithms for labeling is essential to managing the diversity and intricacy of data. Methods based on deep learning, like convolutional neural networks (CNNs) designed for image information, can automate the task of labeling as they learn patterns and characteristics straight from the data itself. These algorithms enhance accuracy and efficiency, especially when dealing with intricate datasets.
Crowdsourcing and Collaboration
Crowdsourcing emerges as a valuable solution to address scalability issues. These platforms facilitate collaboration among numerous annotators and efficiently label colossal datasets. The workload, collectively handled by crowd workers, benefits from quality control mechanisms, redundancy checks, and consensus-based labeling, which ensure accuracy.
Active Learning Techniques
The active learning process begins with the model choosing the most informative instances for annotation. This action reduces the overall burden of labeling. It also improves efficiency by iterative training on a subset of data and selecting uncertain samples for manual labeling while maintaining accuracy.
Clear Annotation Guidelines and Training
To tackle subjectivity and ambiguity, it is essential to set up clear guidelines for annotation. Ensuring annotators receive thorough training, ongoing feedback, and calibration sessions goes a long way toward establishing consistent labeling practices. Equipping annotators with a deep understanding of the task and its context greatly enhances the quality of labeled data.
Conclusion
To navigate the complexities of annotating diverse datasets, a thoughtful approach and innovative solutions are required. By combining advanced labeling algorithms, crowdsourcing, active learning techniques, and clear annotation guidelines, they can effectively address the challenges of data labeling, including diversity, scalability, and subjectivity.
Machine learning relies increasingly on robust data labeling with a human in the loop. These tools incorporate cutting-edge technologies and methodologies, enabling practitioners to overcome challenges and unlock the true potential of labeled data in training high-performance models. In the evolving landscape of AI, efficient and accurate data labeling platforms are key to unlocking the full capabilities of machine learning systems.