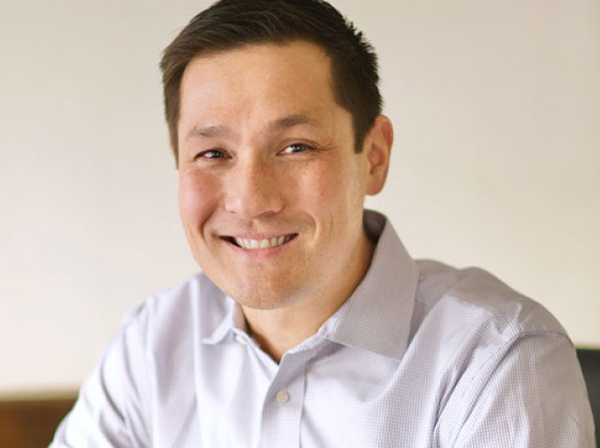
You know you’re not getting the most out of your data. How can your company redesign its data architecture without making the same mistakes all over again?
The data we produce and manage is growing in scale and demands careful consideration of the proper data framework for the job. There’s no one-size-fits-all data architecture, and not all platforms are created equal. Ongoing debates about the benefits and pitfalls of data lakes, warehouses, and other architecture have led many to shift toward hybrid models for managing their data.
Data lakes, once touted as the future of data architecture, have many limitations. For those who implemented data lakes, only to find that they do not optimize data management, the decision to redesign their organization’s entire data infrastructure can be daunting. However, with the right approach, you can make changes with low risk and significant reward. The process of moving away from lakes should be evolutionary rather than abrupt, and the sooner you implement those changes, the sooner organizations can reap the benefits of optimally managed data.
Building the Foundation
Changing your data architecture starts with recognizing that the process spans beyond IT – it’s a company-wide shift. Data literacy and culture are fundamental components of launching or changing data architecture. This shift begins with defining your business goals and value chain. What business problem do you want to solve, and how can your data be optimized to accomplish that goal? Different data architecture offers diverse possibilities for conducting analytics, none of which are inherently better than another. Having a company-wide understanding of where you are and where you’re going helps guide what you should be getting out of your data and what architecture would best serve those needs at each level of your organization.
Once you’ve identified how to manage your data better to serve your organization, you need to establish overarching data governance. Again, data governance is not a set of procedures for IT, but a company-wide culture. An impactful data culture involves a carefully curated ecosystem of roles, responsibilities, tools, systems, and procedures. A data culture tailored to business needs and goals ensures that everyone understands their relationship to the company’s data and contributes to the optimization of its management. By establishing these foundational elements of data literacy, you reduce the risks associated with implementing new architecture.
To further reduce those risks, the process should be gradual and experimental. Like the lean startup approach for launching new ventures, implementing new architecture requires ongoing validation. Validation can only come from making changes and evaluating their effects against the business goals you’ve defined. Instead of taking months of your time to plan before changing the entire data infrastructure at once, begin the process of validation as early as possible by making small, gradual changes that align with business needs. The experimental approach to change saves time and money while reducing the potential negative impacts of making the wrong choice for your business. This outcome could be dire if you’ve changed your entire data architecture without validating your assumptions.
Dialing into Dual Feedback Loops
Your validation comes through two feedback loops. The first is the data feedback loop itself – the process of cultivating useful insights from data. Are you receiving insights that help solve your business problem and propel your organization forward through this new architecture feature? If not, it could mean that you need to readjust your plan for data management. Understanding the effects of implemented changes and the reasons for those effects, will help guide your organization’s process for moving away from legacy data architecture and position you for successful data management.
The second feedback loop that is an integral part of architecture validation is human-centric. This loop involves the ongoing feedback and evaluation of the new roles, responsibilities, systems, and processes associated with changes you’ve made to your data architecture. How are these changes working for your organization? How aren’t they? People often overlook the human aspect of data management, but it is a critical component of optimizing your data and accomplishing business objectives.
Applying New Data Architectures
Successful data architecture looks different for every company. As a result, the processes for moving away from architecture that isn’t serving your organization are equally unique and custom-tailored. It will be messy as you figure out what works for your company. Just like launching a new venture, the gradual evolution of implementing new data architecture is not a linear progression but an ongoing experiment with surprises, failures, and problems to solve. On the other side of those hiccups are immense opportunities to optimize your data management and gain valuable insight.
Architecture decisions driven by technological considerations alone will not position your organization for success. Instead, data architecture decisions should stem from business objectives and needs. To benefit from optimal data management, consider how architecture changes impact the human side of your organization. These changes can’t happen overnight. It’s a process involving every aspect of the organization, from strategy to IT, that will unfold over an extended period of time. By shifting away from data lakes in this manner, you can minimize risk and create opportunities to validate the new architecture and processes along the way.