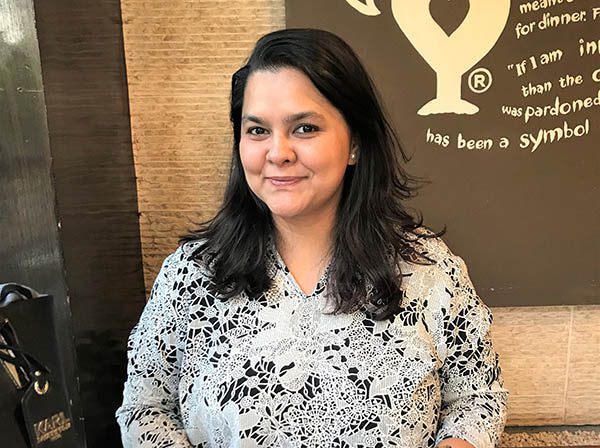
Click to learn more about author Farah Kim.
Although companies are insight-starved and are investing huge amounts of money in setting up data collection and storage infrastructure (tales of data lakes!), they are unable to formulate a Data Quality plan.
In my experience working with enterprise-level clients, the reason behind this is simple — Data Quality is never given the attention it needs.
94 percent of respondents in The Global State of Enterprise Analytics 2020 survey say that data and analytics are important to their organization’s digital transformation efforts.
Yet, a survey by KPMG shows that many CEOs have concerns about the quality of their data.
This begs the question: How can an organization achieve its data-driven goals if it does not have data it can trust?
And therein lies the rub.
In this piece, I’ll cover some basics on Data Quality, why it’s often missed, and what you can do about it.
Here goes!
Defining the Often-Missed Aspects of Data Quality
Data Quality is often lost in translation when bigger ideas like data transformation and data privacy are discussed. When companies talk of AI, ML, analytics, etc., they mean high-tech machines, software solutions, cloud structures, and fancy tech innovations. Data Quality only becomes part of the talk when an embarrassing mistake occurs, when insights and analytics give inaccurate results, when customers get angry, or when there’s a threat of a lawsuit because of data compliance violations.
Little do companies realize that Data Quality is not some random objective to tick off. It’s pretty much a reality that is interwoven into the organization’s business process, its culture, its mindset, and its profitability. Businesses start with the goal of being data-driven, but they completely miss the process, the culture, and the intention along the way.
Let me explain this with a real-life example.
A large online e-learning company decided to upgrade its digital infrastructure by replacing its legacy system. The IT department was tasked with the project and worked in isolation. They chose the new system, implemented it, and performed the data ETL, all without coordinating or communicating with business leaders who depended on that data for their day-to-day job. The company attempted a big bang migration. It was too early to celebrate, though. Post-migration, all hell broke loose. Business leaders from marketing, sales, and customer support discovered that key data fields they relied on for metrics were no longer available in the new system. As if that was not enough, they found out that most of the data got duplicated during the migration. A transformation that was supposed to help the company move forward failed spectacularly.
What were the reasons for this failure?
- They made a classic mistake — treating data and technology as an IT responsibility. Business users were not taken into consideration even though they were the true owners of the data.
- They assumed digital transformation was all about infrastructure when it’s actually about process and culture transformation.
- They underestimated Data Quality and overestimated the system.
This could have been prevented had the company focused on the process and got key stakeholders on board. In this operation, the CEO pushed the responsibility to the CIO, who then tasked his team with the project. With no one to understand the purpose of data, costly mistakes were made, and the company strayed further from its data-driven goals.
Defining a Data Quality Standard
Companies know data is inherently flawed. Some handle it by implementing a basic data cleaning ETL process, while others employ expensive teams to “clean” data. Some completely neglect bad data or fail to implement basic Data Quality standards.
This point is proved in this study conducted by the Harvard Business Review involving 75 executives. The results are staggering.
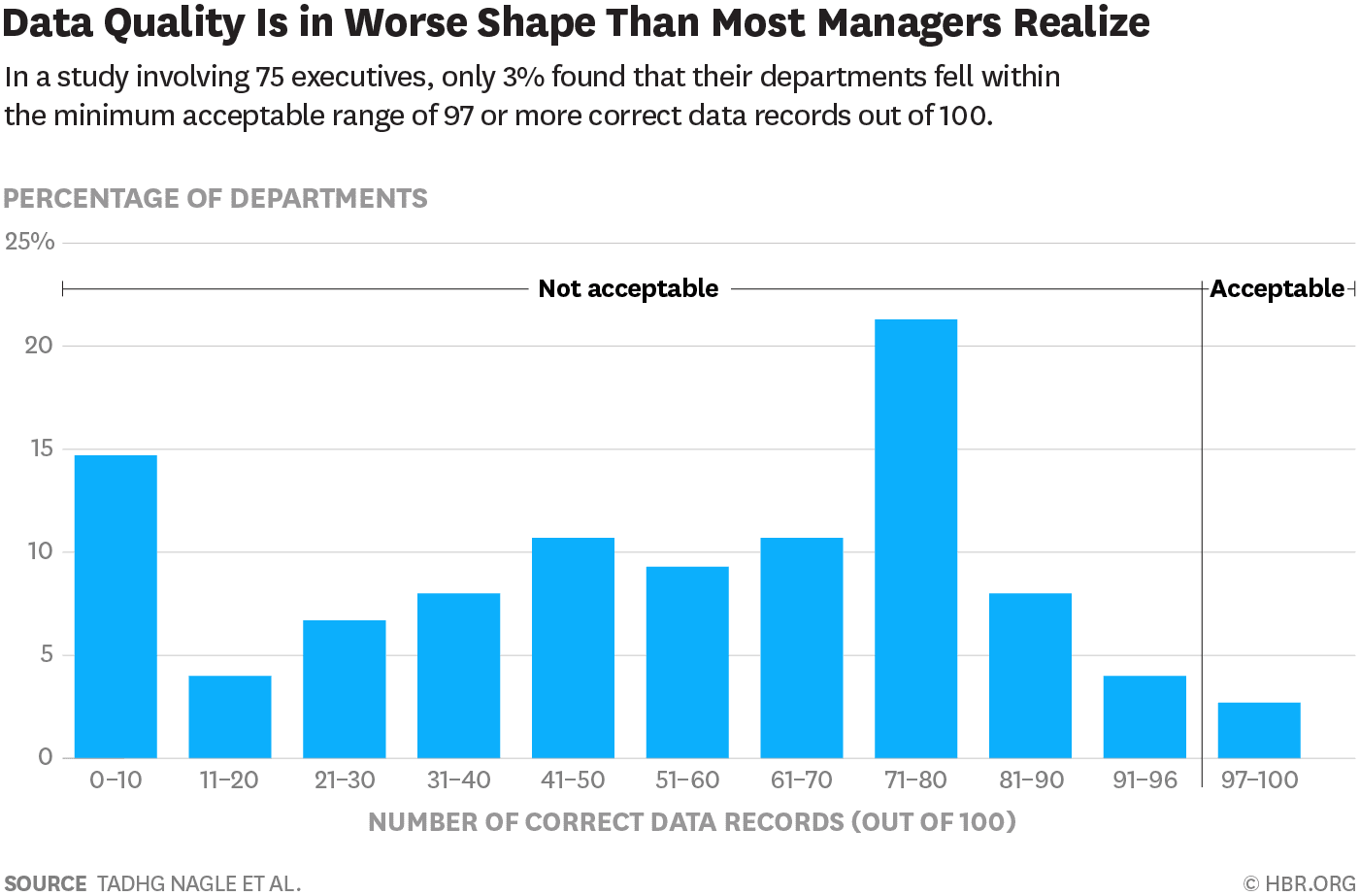
Although this study was conducted in 2017, in 2020, it still holds. As companies are getting more entangled in complex data formats and requirements, Data Quality must be prioritized over every other initiative.
The logic behind this is simple: High-quality data -> successful digital transformations -> better and accurate insights/analytics -> satisfied customers -> increased brand credibility -> profitable ROI -> scalable future.
The question is, how? Some recommended steps are:
- Run an Audit and Profile Your Data
- Use a Data Quality Solution
- Establish a Data Governance Policy and Process
- Set a Data Quality Conscious Culture
- Provide Data Quality Training
Let’s explore each of these steps in detail.
1. Conduct a Data Audit and Profile Your Data
How do you evaluate your Data Quality?
Here’s a summary of how to do this provided by the authors of the study above:
- Gather a list of 100 data records that you use or need to use.
- Identify fields that are important to your analytics (such as age, gender, phone #, etc.).
- Identify whether each of the records has complete, accurate, error-free information.
- If more than two-thirds of the records have errors, you need serious data improvements.
Here’s where a data profiling tool can also be used. A hundred records for a large enterprise cannot always give an accurate picture. Therefore, you need a tool that allows you to explore your data and discover problems for millions of rows of data quickly and accurately, as shown in the image below.
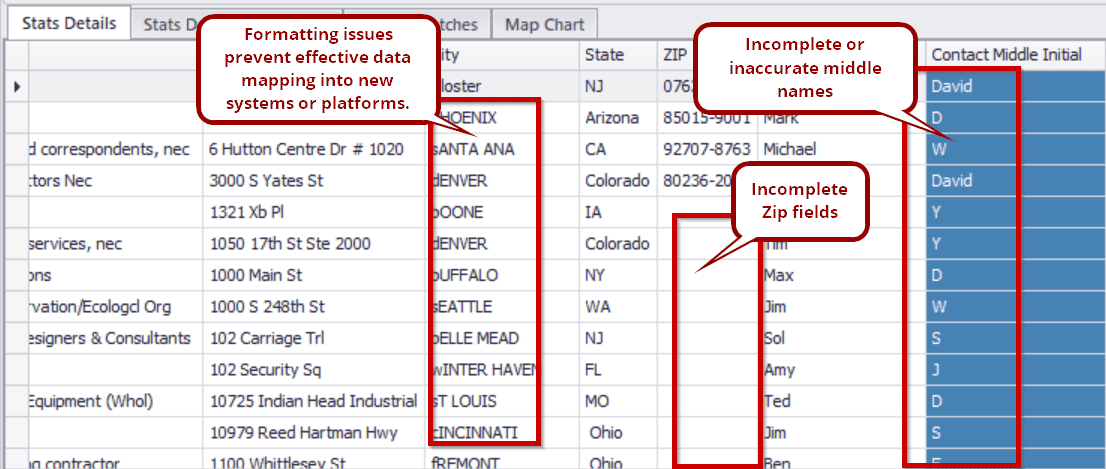
2. Use a Data Quality Solution
When companies realize they have bad data, their knee-jerk reaction is to hire business analysts or data specialists to solve the problem.
Well, you can hire a data specialist to “monitor” or “manage” the problem, but you cannot expect them to come up with algorithms to fix dense Data Quality issues. That’s wasting their talent and their time. Also, it’s not an effective solution. Companies receive GBs of data every single day, and if every record has flaws, it will take BIs forever to fix them — in fact, the fixing will be a permanent part of the job even though their actual job is to derive insights from data.
A best-in-class Data Quality solution encompasses the whole framework.
Fun fact: It also allows business users to manage their data *without* relying on IT.
An automated solution can integrate millions of rows of data from several data sources. It will let the user profile and clean that data, match records to weed out duplicates, and create clean master records within a short period.
To put this in data speak, a commercial solution makes data integration, data profiling, data cleaning, data matching, and data consolidation a breeze, all while maintaining a high level of accuracy. This chain of functions will take months of human effort, and the results will still be inaccurate.
3. Establish a Data Governance Policy and Process
As the Data Quality is evaluated, sources or reasons for errors will come to light. If the issues are caused by careless human-input errors, then data entry policies can be implemented. For example, some companies implement strict web form designs such as a mandatory ZIP field so users can only move forward if they provide their ZIP. This kind of front-end implementation reduces the chances of erroneous data, making it easier for the company to sort its data.
Similarly, a routine data cleaning process or schedule can be put in place to ensure that data is regularly cleaned and is readily available for analytics. Consider a Data Governance process or policy as adopting a proactive approach — you don’t wait for a project to fail to work for quality data. You prioritize quality data as part of your transformation objectives.
4. Set Up a Data-Conscious Culture
See how this is eventually boiling down to changing processes and cultivating a new culture? It’s imperative to reiterate that Data Quality is part of an organization’s culture. When employees are made to realize the importance of accurate data, they will be extra careful (if not innovative) in handling data. When leaders of different departments are synced under the common cause of being data-driven, Data Quality will automatically be on the agenda.
It starts with culture and ends at the transformation. Most companies do it the other way around.
5. Provide Data Quality Training
It cannot be denied — data and technology are everyone’s business, from the CEO to the office administrator. Therefore, everyone needs to receive basic Data Quality training. Employees should be able to understand their role in collecting, recording, managing, and handling data. They should know what constitutes bad data and what practices they can implement to reduce the occurrence of bad data.
Training will help every employee in the company adopt a data-driven mindset and enable the culture to change.
To Conclude
It is no longer enough to want to be data-driven. Companies must implement a data-conscious culture, which is only possible when Data Quality is prioritized, and employees are trained in Data Management best practices. In retrospect, ask yourself and your team — do you have data you can trust?