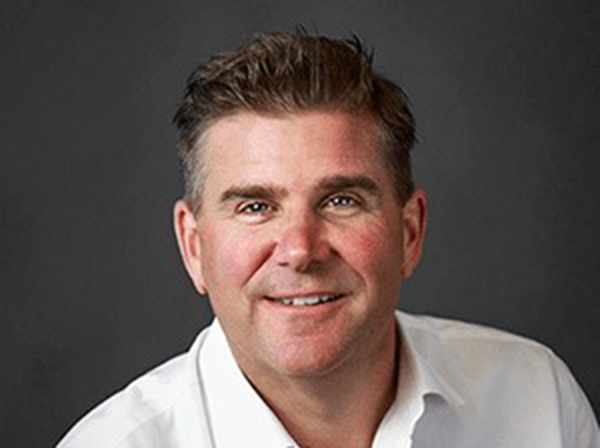
I co-founded my company to focus on the challenges of supporting a large number of data analysts working on disparate sets of data managed in a massive lake. We borrowed the term “semantic layer” from the folks at Business Objects, who originally coined it in the 1990s. The term was actually over 20 years old when we adopted it.
So what is a semantic layer exactly? If you Google the term, the following definition will pop up, which is a pretty darn good definition in my opinion:
“A semantic layer is a business representation of corporate data that helps end users access data autonomously using common business terms. A semantic layer maps complex data into familiar business terms such as product, customer, or revenue to offer a unified, consolidated view of data across the organization.”
Wikipedia defines a semantic layer as a business representation of data that allows end users to access data autonomously. Everyone can agree that a business-friendly view of data that provides users with self-service access to analytics is desirable – true data democratization. It’s easy to see why it is fundamental to scaling data and analytics.
Key Indicators That You Need a Semantic Layer
So how do you know that you need a semantic layer? In this article, we’ll ask some tough questions that can help you answer that question. If you answer “yes” to the following questions, your organization probably needs a semantic layer.
1. Do we use more than one BI/AI tool or data platform?
The larger the organization, the tougher it becomes to impose a single standard for consuming and preparing analytics. Not only is attempting to change user’s habits often futile, it creates a barrier to users making data-driven decisions because they need to learn new ways of asking questions. According to the Dresner’s Wisdom of Crowds® Business Intelligence Study, over half of enterprises report using three or more BI tools, with over a third using four or more. On top of BI users, data scientists have their own range of preference as do application developers.
In addition to a complex analytics consumption landscape, data storage and serving can be even more complex. Data can live in on-premise data warehouses, cloud data warehouses, data lakes, or SaaS applications, making it difficult for users to find, blend and query data.
A semantic layer provides a consistent, business-friendly interface for any query tool and hides how and where data is stored.
2. Do users show a lack of trust in data and analytical results?
Most organizations don’t trust their data, leading to slow decisions or no decisions at all. In fact, according to the recent Chief Data Officer Survey, 72% of data and analytics leaders are heavily involved in or leading digital business initiatives, but they are uncertain how they can build a trusted data foundation to accelerate them.
It’s not hard to see why a lack of trust in analytics outputs is so pervasive. Conflicting analytics outputs are all but assured when multiple business units, groups, business users, and data scientists prepare their analytics using their own business definitions and their own tools.
A semantic layer can drive trust in data by empowering data self-service while ensuring the consistency, fidelity, and explainability of analytic outputs.
3. Do users complain that they can’t get access to data when they need it?
With the fast pace of today’s business climate, waiting for a centralized data team to produce analytics for the business is a thing of the past. The self-service analytics revolution was born in response to the need for businesses to free themselves from the constraints of IT. What seemed like a success at first, however, slowly became a quagmire because self-service forced business users to become data engineers.
As a result, today’s data-driven decision-making is limited to the realm of the advanced SQL jockeys, leading to frustration for the majority of users and shifting the bottleneck to data engineers, instead of IT.
A semantic layer accelerates data access by making business-friendly data accessible to everyone, not just data engineers or SQL experts.
4. Are we reluctant to share data?
Data governance and security are not binary. It’s not enough to just restrict access completely. Rather, a useful data security and governance solution will make sure that data is visible (either completely or masked) to users and groups depending on their authorization level. For example, the finance group of a public company with insider status may have access to revenue data while the marketing team does not, and the HR department may have access to full social security numbers of its employees while the rest organization can only see the last four digits.
Implementing a comprehensive security and governance strategy for your data yields benefits far beyond just securing data access. With the confidence that your data is consistently secure for every type of access, organizations can make all data available to their employees and partners. However, achieving consistent governance in a complex environment with multiple access vectors (i.e., BI tools, AI/ML tools, applications) and multiple data stores (i.e., data lakes, data warehouses, SaaS applications) is impossible without a single control plane to apply data security and governance at query time.
A semantic layer applies data security and governance to every query by enforcing access policies and rules to users and groups in real time, making data sharing ubiquitous.
5. Are users resorting to creating data extracts or imports to get the query performance they need?
Users demand data access at the speed of thought. Cloud data platforms have improved query speed and scale dramatically, but they are still not fast enough to deliver queries under a second consistently. Waiting 10, 20, 30 seconds, or longer for a query is not acceptable and users will find a way to achieve the speed they desire by resorting to data copies or cubing solutions like Tableau Hyper Extracts and Power BI Premium Imports. This solution is suboptimal because it creates data copies and data latency, and requires processes to update these caches. Furthermore, external caching schemes also introduce security concerns and often create inconsistency in results given that data may be captured at different time intervals.
The alternative to avoiding data movement and external caches is to deliver a live, performant connection to data where query performance is adaptively tuned and queries are rewritten in real time.
A semantic layer leverages the power of cloud data platforms by autonomously managing query performance in situ using end user query patterns and machine-generated aggregates to deliver queries in under one second.
Summary
As you can see above, a semantic layer can remove friction and make data available to everyone in your organization, not just data engineers or SQL jockeys. Not surprisingly, a universal semantic layer is becoming a critical component in a modern data and analytics stack.