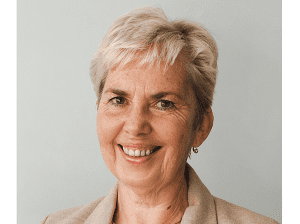
I was privileged to deliver a workshop at Enterprise Data World 2024.
Publishing this review is a way to express my gratitude to the fantastic team at DATAVERSITY and Tony Shaw personally for organizing this prestigious live event. Participating in such events has multiple advantages, including becoming familiar with trending topics in the data management community worldwide, sharing your experience, and learning from leading data management practitioners in face-to-face mode.
I want to share some insights I gained during this event. This article will review the key trending topics discussed at Enterprise Data World 2024. Please note that this review is general and does not reference particular presentations delivered at the conference.
Data governance, data modeling and architecture, data science, and other data management capabilities were core subject areas discussed at Enterprise Data World 2024, as shown in the figure below.
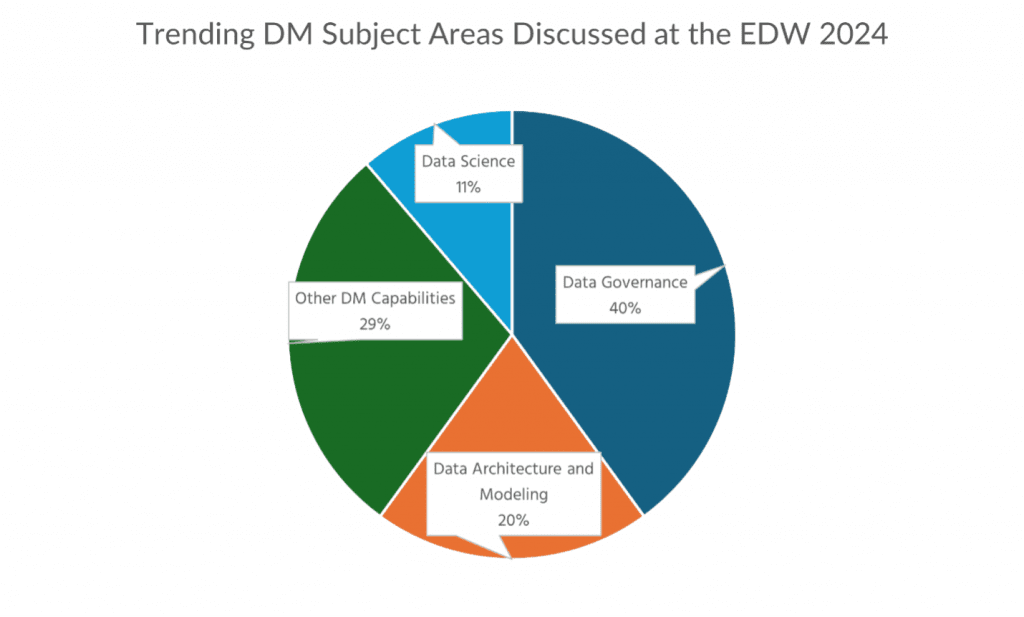
Let’s examine these subject areas in greater depth and discuss the most popular topics in each area.
What Is Data Governance?
The biggest challenge with data governance is that this concept has no aligned definition in the data management community worldwide. DAMA-DMBoK2 defines it as “the exercise of authority, control, and shared-decision making (planning, monitoring, and enforcement) over the management of data assets.” The essence of this definition is that data governance governs data management, not data itself! However, somehow, we have lost this key statement and interpret data governance quite differently. DAMA-DMBoK2 considers a data management operating model, policies, processes, and roles as the core deliverables of data governance. However, to some extent, DCAM and Gartner substitute data management in the DAMA-DMBoK2 context with data governance. If you are interested in this topic, I recommend you read this series of articles.
At EDW 2024, participants focused on the following six key topics:
Data Governance and Stewardship
The key takeaways to think about are:
- Data management professionals use different frameworks to describe the content of data governance. For example, the DCAM data governance model includes multiple deliverables that, from the DAMA-DMBOK2 perspective, can be considered deliverables of data modeling and architecture (i.e., business glossary, data products) and metadata management.
- Different approaches exist to define data management roles. However, there is no distinction between functional roles (included in the organizational structure, such as data architect) and virtual roles (to be assigned to the functional roles, e.g., data owner). The role of a data user that balances the role of a data owner along a data chain is often omitted.
- Data policies are essential to data management, as they define how the data management function should operate.
- Data management operating models again get attention. There is growing interest in a federated operating model, which is one of the key components for successfully implementing a data mesh. It is worth noting the assumption that different data management operating models have various levels of agility. A company should seek the opportunity to create a democratized operating model as an alternative to centralized and federated. I don’t entirely agree that some models are generally better than others. An operating model must be aligned with the company’s size and data architecture type. However, embedding the democratization approach can increase the efficiency of any operating model.
- Implementation of data governance in public and scientific institutions has its specifics.
- Involvement of an organization’s executives in data governance activities requires many communication efforts, but it’s worth it.
Data Culture
Data culture is a set of collective behaviors, values, and norms in an organization related to the business value of data and its importance for achieving its goals.
It would be best if you thought about the following:
- The maturity level of data culture can be measured. Each organization should strive to reach the level where data culture is fully embedded into the organizational culture and is a part of business operations. Each employee must be involved in the journey.
- Building robust data management requires an entrepreneurial mindset. It includes strong stakeholder management and communication strategy.
Data Literacy
Data literacy is the ability to read, understand, create, and communicate data as information. It encompasses the skills required to ask the right questions about data, interpret data and its analysis, and understand the ethical implications of data use.
The key topics to think about are:
- Many business challenges are rooted in poor understanding of data and insufficient data management. This leads to a situation where data is considered a debt, not an asset, and causes the depletion of organizational resources. Enterprise leaders must support the establishment of data literacy programs.
- Gamified learning is one of the ways to promote and increase the efficiency of data literacy programs.
Data Monetization
Data monetization is an organization’s ability to generate economic benefits from its data and data assets.
The key takeaways regarding data monetization are:
- Economic benefits can be monetary and non-monetary.
- An organization can get monetary benefits by selling data, directly or indirectly, to third parties.
- Non-monetary benefits can be achieved by enhancing existing products and services, improving internal efficiency, and decreasing costs through data-derived insights.
- A data monetization strategy encourages organizations to consider data as a product and apply the corresponding general process or program to develop, implement, market, and deliver these products to the right consumers.
Data Privacy and Protection
Data privacy and protection refer to an organization’s ability to safeguard personal or sensitive information from unauthorized access, use, disclosure, disruption, modification, or destruction.
An organization should keep in mind the following:
- Personal data has a wide range of identifiability. To protect data, organizations should use multiple techniques, technologies, and tools like data anonymization, encryption, de-identification, hashing and tokenization, cryptographic computation, statistical disclosure limitations, etc.
- Implementing data protection requires changes in data governance and architecture.
- Protecting data in the cloud requires different approaches.
Data Strategies
In my practice, I use the following definition of a data strategy: “A data management strategy is a long-term future state document that demonstrates the intention of a company to manage and use data by its business strategy.”
Many organizations nowadays put efforts into developing data strategies. It is worth noting the following:
- Different frameworks and models of data strategy exist. In developing strategies, organizations do not always follow the recommended content (more in the article: “A Data Strategy: Theory vs. Practice.”
- A data strategy should include strategies for developing various data management capabilities.
- In developing a data strategy, an organization can use commonly used techniques, such as a business model canvas and SWOT analysis.
- Even ChatGPT knows that the core pitfalls in data strategy implementations are a lack of skills, clear business objectives, resistance to change, and cultural barriers.
- Organizations should consider the “green information management” approach when developing data strategies. This approach focuses on minimizing the environmental impact of information-related processes.
In Part 2, I will share key takeaways on data science, data architecture and modeling, and other data management capabilities.