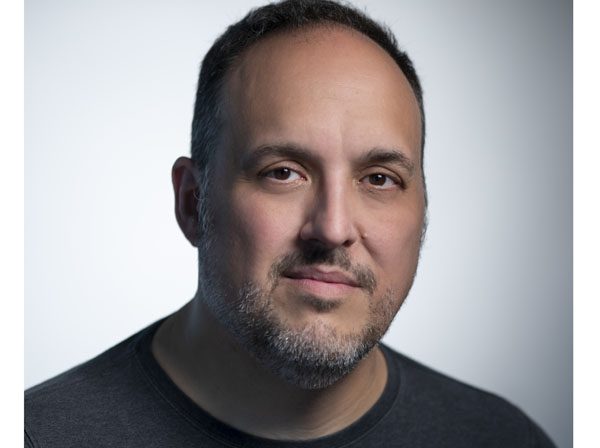
As enterprises continue to struggle with the effects of the global pandemic, the modern data analytics stack is undergoing a shock of its own. The world has changed, and we’re living in a new hybrid multicloud reality. Lower levels of the IT stack, which is to say, data centers, networks, raw storage and compute, are making their way up stack and are impacting both how we analyze and integrate data. Organizations want to empower data and analytics teams to connect any type of data, uncover impactful insights, and speed time to market, so expect to see the following key shifts.
The Rise of the “Just-in-Time” Data Analytics Stack
There’s a small, but fast-growing, segment of the data analytics space that is focused on new approaches to the enterprise stack, including continuing to move all the things to the cloud. However, the hybrid multicloud imposes requirements of its own, most notably the ability to manage and analyze data no matter where it lives in the hybrid multicloud environment.
Startups like Starburst, Materialize.io, Rockset, and my own company develop platforms that are designed to query, search, connect, analyze, and integrate data where it lays without moving or copying it, in a just-in-time fashion. In a world where the number of places that data may be residing in storage is increasing rather than decreasing, enterprises will continue to seek data analytics solutions that are not coupled to where data lives, especially as data movement between storage systems continues to be removed from the stack in order to accelerate time to insight.
Knowledge Graph-Enabled Data Fabrics Become the Connective Tissue for Maximizing Analytics Value
Gartner indicates that data fabric is the foundation of the modern Data Management platform, with capabilities for data governance, storage, analytics, and more. Relying on traditional integration paradigms involving moving data and manually writing code is no longer acceptable, as data scientists and data engineers spend almost 80% of their time wrangling data before any analytics are performed. Shrewd organizations looking to adopt this approach are realizing that the centerpiece of a properly implemented data fabric is an enterprise knowledge graph, which compounds data fabric’s value for better, faster, lower-cost analytics while hurdling the data engineering challenges obstructing them.
Organizations are adopting enterprise knowledge graph platforms to support their data fabrics that use a combination of graph data models, data virtualization, and query federation – along with intelligent inferencing and AI – to eliminate this friction by simplifying data integration, reducing data preparation costs, and improving the cross-domain insights generated from downstream analytics.
The Era of Big Data Centralization and Consolidation Is Over
The importance of centralized or consolidated data storage has also become apparent. To be clear, this isn’t the end of storage, but it is the end of centrally consolidated approaches to data storage particularly for analytics and app dev. We are seeing the continuation of the big fight that’s brewing in the data analytics space as old ways of managing enterprise data, focusing on patterns of consolidation and centralization, reach a peak and then start to trend downward. Part of what we’re about to see unfold in the big fight between Snowflake and Databricks is a function of their differing approaches to centralized consolidation.
But it’s not just technical pressures. The economics of unavoidable data movement in a hybrid multicloud world are not good and don’t look to be improving. Customers and investors are pushing back against the kind of lock-in that accompanies centralization approaches, so anticipate the pendulum swinging in the direction of decentralization and disintermediation of the data analytics stack.
Data Fabric Goes Mainstream
Data fabric is the future of Data Management according to analysts, but the maturity of enterprise data fabric as the key to data integration in the hybrid multicloud world is becoming more commercially evident. High-profile enterprise adoption around use cases like analytics modernization, acceleration of insights from data lakes, digital twin in manufacturing and supply chain, as well as drug discovery and supply chain control tower in pharma and life sciences will become even more prevalent.
Just as race cars without high-octane fuel sources are no more than beautiful, static sculptures, analytics platforms including AI/ML without total data mastery, accessibility, and innovative data integration solutions will fail to live up to their potential. Market signals also suggest that the enterprise itself will get serious about finding new ways to integrate and connect data in the new hybrid multicloud world we all live in.