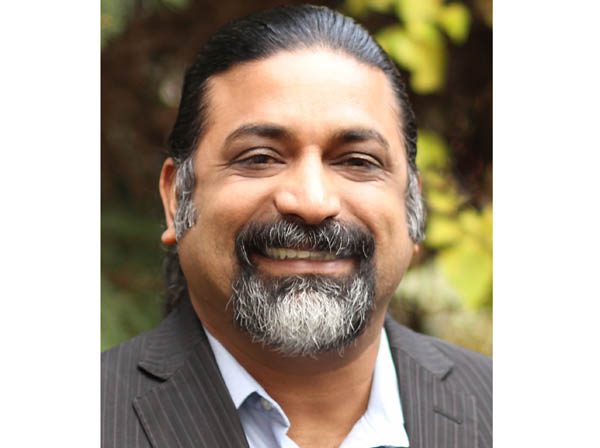
Click to learn more about author
Sam Mahalingam.
The profile of data analytics has never been higher. Faced with the unprecedented challenges of COVID-19, governments around the world are typically relying on advanced statistical modeling to make sense of a barrage of constantly changing metrics. Data scientists are playing a critical role, advising decision-makers as they seek to implement the right policies at the right time.
Of course, deriving deep insight from rich data is nothing new. In recent years, the rapid evolution of sophisticated tools that enable efficient real-time analysis has been among the defining trends of industry and enterprise.
Looking ahead to 2021, there is no sign of this momentum easing. The emergence of artificial intelligence (AI) and machine learning (ML) will continue to transform the ways that data analytics is designed, consumed, shared, and integrated. They will help set new benchmarks in terms of accessibility, the speed at which insight is delivered, and the extent to which it replaces as well as augments human decision-making.
Over the next 12 months, four key trends will power these developments:
Augmented Analytics
This represents the next wave of disruption in data analytics. Leveraging the latest natural language processing (NLP), AI, and ML techniques, it is already redefining how data analytics is used and by whom.
First identified by Gartner in 2017, there are compelling reasons why augmented analytics is coming of age. Above all else, the sheer volume of information available to organizations is making effective interpretation more and more challenging. And it’s only getting worse. To cite just one example, by 2025, there will be over five billion cellular IoT connections worldwide, up from 1.3 billion in 2019. What’s more, insight derived from data typically has a short shelf life. If it does not reach decision-makers in good time, the value soon fades.
ML and AI are ideally suited to automating and accelerating the most laborious elements of a data scientist’s work: gathering, preparing, cleaning, and finding patterns and correlations in data. However, the significance of augmented analytics will go far beyond easing the burden on overworked specialists. By making sophisticated tools accessible to a far wider audience, augmented analytics will empower the new generation of citizen data scientists. Frontline employees — business line managers, operations managers, and domain experts, for example — will be given control of the tools they need to reach better decisions faster.
Continuous Intelligence (CI)
The rapid growth of CI reflects the sheer speed at which modern enterprises operate. To get decisions right, we need to know what’s happening now — and the best course of action to take in response. In 2021 and beyond, more of these answers will come from CI, a design pattern in which real-time analytics is integrated within a business operation. Processing both current and historical data, it will increasingly be employed to automate rather than support the decision-making process.
The tools leveraged by CI will be rich and varied. They include augmented analytics, as well as event stream processing, optimization, business rule management, and ML. Moreover, 2020 has made it all too apparent that ”set and forget” is no longer an option. Markets change, behaviors evolve, events happen. Today’s predictor will not work forever. In fact, it might not even work tomorrow. CI will make an impact in 2021 because it delivers tools that learn on their own, continually adjusting and improving. Just as augmented analytics is fueling the rise of the citizen data scientist, CI will be embedded into existing workflows, not siloed with Data Science specialists.
Augmented Data Management, Data Discovery, and Data Catalogs
These will also be big news in 2021. Once again, it is ML capabilities and AI engines that are being put to work. In this case, they will be used to find and understand data and make enterprise information management categories — including data quality, metadata management, master data management, and data integration, as well as database management systems (DMS) — self-configuring and self-tuning.
Auto ML/Explainable AI
As I’ve already suggested, 2021 will see an accelerating shift towards automating the decision-making process. However, in many cases, organizations will still need to explore and justify how such decisions are made. Application leaders must, therefore, be able to build models that are interpretable and explainable. The “black box” approach taken by some solutions only serves to shut out users. Increasingly, analysts will instead be looking for responsible, explainable, and interpretable AI that lifts the lid on the models deployed. This transparency inspires greater confidence in the results generated and will give the growing army of citizen data scientists an opportunity to learn and grow with their systems. Moreover, models can be modified and fine-tuned in line with ever-changing environments. In 2021 and beyond, there should be no need to choose between automation and democratization. With the right solutions in place, the two will go hand in hand.