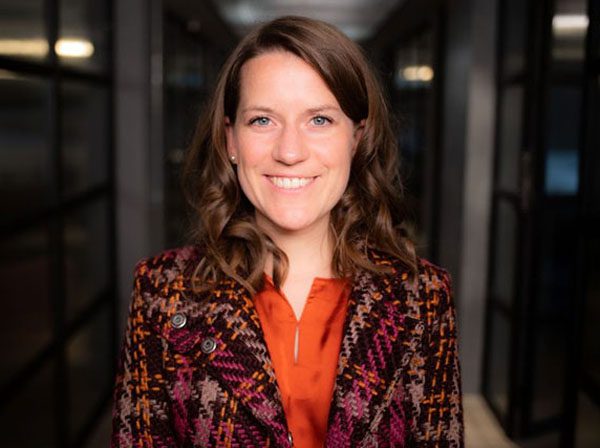
Click to learn more about author Eva Murray.
The push for digital transformation is nothing new. Yet the accelerated adoption of digital transformation inspired by the coronavirus pandemic is unlike anything we have ever seen before. Companies have been forced to quickly ramp up their digital strategies in order to survive in our new world of virtual business. Those that could not quickly pivot and reset their business strategy did not survive.
Now that we are moving beyond the initial rush to adapt to the digital workplace, what did we learn from that first phase of the pandemic? Companies that can evolve and retune their business strategy endure. This has never been more evident than in today’s rapidly changing business landscape and uncertain economy.
Advanced Data Analytics Is King —Going Beyond Data-Driven
Historically, being a data-driven business has been the goal. That was fine then, but times have changed. Today, companies have to be data-driven just to compete in the chaotic post-COVID-19 world. The ability to pivot and quickly change your business strategy as it relates to people, products, and/or processes based on current events — whether they are happening in the world market or internally — has never been more apparent than it is today.
The goal now is going beyond data-driven, which means using advanced data analytics to drive real-time business strategy.
Drive Business Agility and Strategy with Advanced Analytics — Maximizing the Three Pillars of Data Analytics
The ability to harness the power of advanced data analytics that ultimately helps drive agile business strategies requires strong data analytics pillars. These pillars include the trifecta that is speed, agility, and scalability, and below is some practical advice on how to strengthen each one.
Speed (and Performance) — Supporting GPUs
According to Gartner’s 2019 CIO Agenda survey, between 2018 and 2019, organizations that deployed AI grew from 4 percent to 14 percent. With the adoption of AI continuing to grow, businesses need to look beyond the traditional use of CPU power and bolster their AI and machine learning (ML) applications with Graphics Processing Units (GPUs). This will allow them to develop, train, and retrain, and run their analytical models faster, which in turn can lead to better products and services for their customers.
GPUs enable organizations to massively parallelize operations to support the training of analytical models and/or inferencing, providing the scale and performance required to efficiently complete multiple epochs in a shorter timeframe and to fine-tune the model. Furthermore, using GPUs in the cloud gives organizations the ability to run different AI/ML workloads with the flexibility needed for a cost-effective, scalable, and secure AI solution.
Agility — Solving the Model Problem
Search engines today often lead people to pick the quick option instead of what they were looking for, so the algorithm might influence their search rather than the other way around. This happens because underlying data drives the suggestions for autocompleting a search query, a process that relies heavily on the data model. However, a data model is only as good as the information used to create it and the requirements communicated by the business to the data engineers.
A data model should bring together all the relevant data and related tables from different data sources so they can be queried by analysts in their entirety and in relation to one another. Otherwise, the information and the value of the insights that analysts produce is limited.
To account for all the necessary data that analysts will require, it is important that data engineers, data analysts, and business stakeholders communicate with one another to outline business requirements, the intended use of the data, as well as potential (current) limitations when it comes to data access. Such communication requires ongoing conversations, asking the right questions, and agreeing on the business needs and timelines everyone is working towards.
At the heart of the Data Strategy should be the consideration of the environment the business operates in. We see the impact that the environment plays right now during a pandemic amid so much uncertainty. One method of creating an agile data model, as well as a data warehousing approach, is to use data vault modeling. Data vault enables organizations to easily grow their data volumes and respond to rapid business changes, keeping their data model flexible while also maintaining a detailed data catalog. This proves very useful for compliance and auditing requirements as a full history of the data is available.
Performance — Including Semi and Unstructured Data
Semi and unstructured data is much trickier to analyze than structured data but is far more prolific in the enterprise. IDC predicts that 80 percent of worldwide data will be unstructured by 2025, with major factors including the rise of IoT and social media content. To remain competitive, organizations need a method of bringing a broad array of data together in a 360° view that provides deeper, more accurate, and more precise analytics insights. The ability to support semi-structured data formats like JSON is imperative to business success as it offers business advantages to companies that handle and analyze it well. In addition, AI algorithms can help extract meaning from large volumes of unstructured data, driven by data scientists and data analysts with deep expertise in developing the right models and approaches to work with this data.
By implementing these steps, enterprises can create a sustainable Data Architecture that enables them to go beyond being data-driven to truly drive business intelligence with advanced analytics. And while I cannot predict what will happen next in these unprecedented and wildly unpredictable times, strengthening the pillars of your data analytics strategy is one of the most effective ways to navigate an uncertain environment.