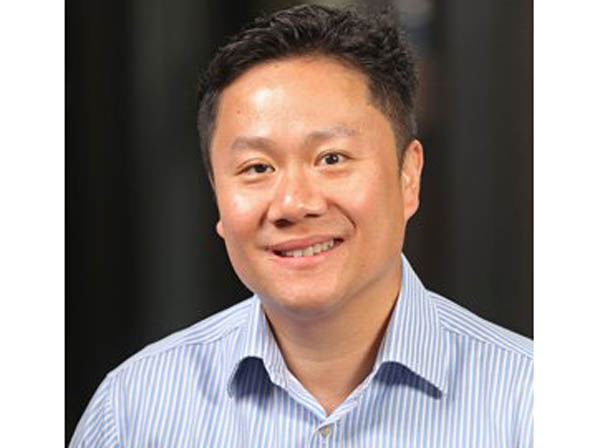
Click to learn more about author Kaycee Lai.
As analyst and journalist Bernard Marr put it, “Without data scientists on staff or available to interpret data and turn the intel into solid business activity, the benefits of data could remain unlocked.” Augmented analytics promises to improve the ability of organizations to derive benefits from data by:
- Taking more mundane analytics from Data Science teams so they can focus on more complex problems
- Shortening the data analytics lifecycle
- Giving decision-makers fast access to actionable intelligence
At a high level, augmented analytics involves using machine learning and natural language processing (NLP) to assist with data prep, analysis, and reporting, eliminating the things that slow down analytics. But it promises to do more than just that – augmented analytics also involves rewriting the entire analytics and BI workflow.
Augmenting the Data Analytics and BI Workflow
To do this, let’s start by examining the current typical workflow, which is composed of the following steps:
- Identify the KPIs and related questions
- Explore the data
- Prep the data for analysis
- Build views and dashboards
- Users explore those dashboards
- Users conduct root cause analysis
- Users combine and share their findings
- These findings are presented as recommended actions
- Actions are taken
Augmented analytics essentially takes all but the first and last portions of this workflow and “augments” them. Under this process, a user – who may not even be an analyst, but rather a non-technical business user who is empowered by no-code analytics – comes to the platform with a relevant set of KPIs and a business question, and queries the system, which then takes care of the rest and comes back with recommended actions.
If this sounds far-fetched, consider that the three components that Gartner has defined as being foundational to augmented analytics are not part of a next-gen wish list, but are well-developed technologies that have been broadly put to use in many other arenas:
- Machine learning
- Natural language generation and natural language processing
- Automation
Machine learning is something of an umbrella term describing algorithms that make “decisions” based on weighted probabilities. It allows advanced analytics but also serves as the basis for the other two technologies identified by Gartner as critical components of augmented analytics.
Removing the Gatekeepers
Natural language generation (NLG) and natural language processing (NLP) translate computer-speak into human language, and vice versa, allowing humans and computers to interact without the help of someone who knows how to write computer code. We’re familiar with this by now. When we ask our phone to navigate us to the nearest pizza parlor, we’re essentially using augmented technology to gather data, perform a calculation, and then translate that calculation into something we can understand – directions in natural language.
Applying this augmented technology approach to answering a business question based on KPIs really isn’t that big of a leap. Imagine the following scenario:
The user asks, “What were sales of frozen pizzas in 2021 compared to 2020?” The augmented analytics solution responds with “Sales of frozen pizza declined by 30% between 2020 and 2021.” The user then asks a follow-up like “In which region were sales of frozen pizza the lowest in 2021?” The platform responds, “U.S. Western.” The user might continue to query the system, learning that a certain line of pizzas, which sold very well in other regions, was severely understocked.
It’s not difficult to imagine how this would be a big time-saver. But to truly appreciate the value in terms of what the user does not have to do, let’s take a look at what is going on under the hood of this process and see what is happening.
The user’s question – “What were sales of frozen pizzas in 2021 compared to 2020?” – is converted through NLP into a SQL query. That query is executed through not just one database, but through potentially a dozen or so systems that the company might use – think about a query being executed simultaneously across databases in Teradata, Oracle, Hadoop, and so forth.
If the data required to answer the question resides in different systems, the augmented analytics platform automatically joins those tables.
It performs the query, which returns the data. But the system doesn’t just spit out the data. Instead, it uses NLG to translate that data into human speech.
This process is cycled through several times until the user finally arrives at an actionable conclusion. The user then makes this recommendation to the appropriate decision-makers. This augmented process happens at a speed that is many times faster than traditional human-led analytics, making it possible to iterate and validate decisions at an infinitely greater scale.
The user doesn’t have to code. Nor do they have to consult a data analyst, which may entail waiting for weeks or even months for an answer. They don’t have to wade through the company’s vast, siloed data reservoirs – and consequently, they don’t have to deal with any of the gatekeepers at the various departments that might slow or prevent their access to the data.
Give the Really Tough Questions to the Experts, and “Augment” Everything Else
In summary, augmented analytics promises to add unprecedented speed and scalability to the data analytics process. This doesn’t mean that SQL coding won’t continue to be a valuable skill, or that data scientists’ expertise won’t be required. There are certain questions – particularly those that involve predictive machine learning – that will require a highly trained human brain.
What it does mean, is that those highly paid data scientists, analysts, and architects won’t be spending their time doing rote work and answering more common questions.