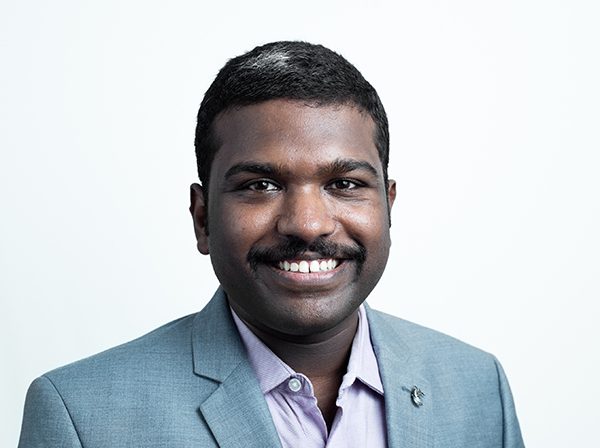
Click to learn more about author Ramprakash Ramamoorthy.
With Big Tech, police departments, insurance companies, and college admissions offices increasingly relying on algorithms to make long-lasting decisions, it’s vital that we take a step back to consider the societal effects of algorithmic decision-making.
There have been numerous examples of AI algorithms exhibiting gender and racial biases within society, including those making decisions across criminal justice systems, corporate hiring, and other business strategies. It’s unlikely that companies and institutions seek to exacerbate racial, gender, and socioeconomic inequities, but how do their algorithms end up doing so?
Where Algorithmic Decision-making Goes Wrong
A focus on explainable AI algorithms is essential for preventing such biases. When a technology vendor builds an explainable version of AI – one that justifies all their predictions – this provides enhanced transparency and can help identify any potential issues to prevent biases. However, it is up to the human to identify patterns. Feedback and analyses are essential for identifying any limiting classifiers that may be causing bias.
Explainability is key, and without transparency, algorithmic bias is inevitable. Also, machine learning algorithms are only as good as the data that they’re built upon. If algorithms are fed incomplete data, unrepresentative data, or data infused with historical human biases, problems will arise.
As mathematician Cathy O’Neil warns in her book Weapons of Math Destruction, if not properly mitigated, algorithms can codify the past, reinforce the status quo, and perpetuate societal inequities. With an incomplete data set, algorithms reinforce the status quo.
What Are the Solutions?
In order to protect against biases in algorithmic decision-making, companies must conduct periodic audits to ensure “algorithmic hygiene” before, during, and after implementing AI tools.
- Conduct Audits:
Be sure to frequently examine your algorithms for biases and delete any biased associations you discover. It’s important to classify things appropriately, as these decisions are inherently political. As O’Neil notes, “These models are constructed not just from data but from the choices we make about which data to pay attention to – and which to leave out.”
- Get Feedback from Users:
In addition to internally gauging your algorithms’ performance, it’s vital to seek out feedback from your customers as well. Users can identify content that was marketed to them inappropriately, including odd recommendations from conversational AI assistants, irrelevant emails, and a host of other algorithmic errors.
- Ensure Your AI Tools are Transparent and Explainable:
Although software companies’ algorithms are often part of their intellectual property, it’s important to avoid the “black box effect.” When you automate your emails, processes, and decisions, your AI needs to be explainable. For any given automated behavior, developers must be able to explain why the algorithm engaged in that particular course of action.
One reason why Billy Beane’s Moneyball theory was so persuasive was because Bean’s decision-making algorithm was transparent. O’Neil writes, “Baseball models are fair, in part, because they’re transparent. Everyone has access to the stats and can understand more or less how they’re interpreted.” Software companies should follow this lead by making their decision-making algorithms independently and ensuring that these algorithms are as transparent as possible. With transparent algorithms and frequent auditing, you’ll ensure that your customers are satisfied and your algorithms are free from biases.