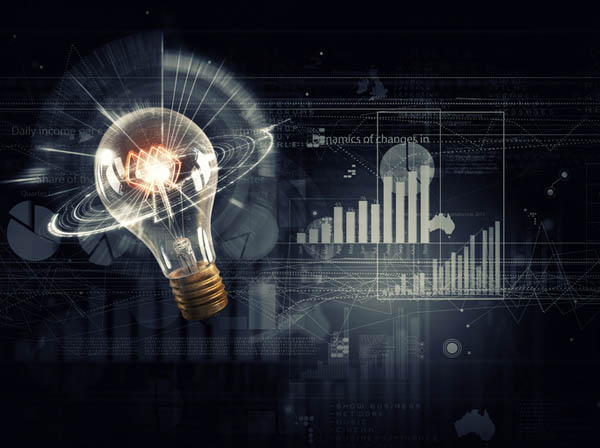
“Graph is leaving a larger and larger footprint. And that is good,” said Thomas Frisendal in Knowledge Graphs and Data Modeling. Gartner named knowledge graphs as part of an emerging trend toward digital ecosystems, showing relationships among enterprises, people, and things, and enabling seamless, dynamic connections across geographies and industries.
Elisa Kendall and Deborah McGuinness, presenting at a recent DATAVERSITY® Data Architecture Online Conference, shared use cases and some of the reasoning behind the expanding use of knowledge graphs. Kendall is a partner at Thematix Partners, and McGuinness is CEO of McGuinness Associates Consulting and professor of computer and cognitive science at Rensselaer Polytechnic Institute.
Origin of Knowledge Graphs
Though the term “knowledge graph” is more recent, the underlying technology has been around for decades, Kendall said. According to Lisa Ehrlinger and Wolfram Woess in Towards a Definition of Knowledge Graphs by the Institute for Application Oriented Knowledge Processing, the term “knowledge graph” originated in the 1980s, when researchers from the University of Groningen and the University of Twente in the Netherlands used it to formally describe a system that represented natural language by integrating knowledge from different sources.
The term came into wider use in 2012, when Google used it to describe the process of searching for real-world objects rather than strings. Other companies, such as Yahoo and Bing, followed suit, and its use with search engines continues today.
Search engines collect user information throughout the click stream, then encode it in a knowledge graph so that the engine can provide better contextual answers. Although not always a perfect match, when enriched with metadata, sensor data, video, location information, and collected analytics about users they think are similar, relevance is greatly increased.
Terminology: Knowledge Graphs, Databases, and Ontology
Kendall introduced three key terms associated with knowledge graph use:
An ontology is the conceptual model of some area of interest or discourse. It:
- Represents elemental concepts critical to the domain
- Typically includes definitions and relationships, not the actual data elements or instances
- Can provide users with queryable local access to common, standardized terminology with unambiguous definitions
A knowledge base is a persistent repository for metadata representing individuals, facts and rules about how they are related to one another (a knowledge graph). An ontology can be included, or separately maintained.
A knowledge graph links collaborators, ad hoc captured knowledge, and workflows. It:
- Provides repository integration of source datasets, analytics workflow code, results, and publications
- Enables knowledge-enhanced search capabilities
Ontologies
Although it’s possible to use data science and machine learning to extract the necessary elements for an ontology, Kendall said that it’s not quite that simple with today’s massive data stores:
“In order to find the needle in the haystack, or to actually be able to reuse the training sets, or leverage any of the knowledge out of the organization itself, what you really want to do is first be able to access what appears to be a global or distributed graph, so it looks consistent.”
The end result may look like a single source to the data scientists, but in fact, it’s using multiple protocols, multiple kinds of databases, different vocabulary, and different assumptions that are highly distributed within their domain, she said.
Use Case: Global Supply Chain Challenges
A large pharmaceutical manufacturer Kendall worked with was using machine learning to manage supply chain incidents, such as unsatisfactory tolerances in raw materials, ships being delayed by monsoons, or delays with just-in-time manufacturing. Most of their databases were structured, but they also included fields within the database written in natural language, using jargon describing raw materials, or weather, or other comments that were used to describe reasons for each incident. Their machine algorithms hadn’t learned how to address these fields, so Kendall worked with them to provide an ontology that included all their chemicals, raw materials, suppliers, and manufacturing facility processes.
The company was then able to augment what they already knew from generic machine learning and natural language processing (NLP) representation with this custom ontology to get better reporting. There is an increasing demand for this type of hybrid solution, she said, where controlled vocabularies are added to existing standard ontologies, as well as a growing demand for more custom work.
Custom ontologies enable larger companies to use a much richer and more relevant set of terms and queries, and more accurately describe their products and services for reporting, regulatory compliance, or decision support applications.
Use Case: The Story of Tuna
In its simplest form, a knowledge graph can connect a consumer to the story of a product. Kendall showed how Bumble Bee Tuna gives customers the opportunity to trace the origin of the tuna in the can they’ve bought to the precise location where it was swimming, how and when it was caught, the name of the ship, how it was processed, and the location of the cannery.
On Bumble Bee’s Trace My Catch website, customers can enter a code from the bottom of a can of tuna, salmon, or any other Bumble Bee product, and the site displays all the information about the contents of that particular can. In terms of understanding what has impacted a product throughout the food chain, she said, “This is just the tip of the iceberg.” The implications for food safety are significant, not the least of which is enabling the possibility of quicker containment in the event of a contaminant or other food safety hazard.
Use Case: Post-Crisis Regulatory Compliance
In recent years, regulatory agencies worldwide have implemented measures to correct the issues that led to the financial crisis of 2008, and financial organizations have struggled to comply. Kendall cited a group of 30 banks subject to principles set by the European Union Banking Commission, and only five were able to comply with the requirements set for 2016.In subsequent annual analyses, not only had the banks not met those standards, but as of a report that came out this year, they made no effort to do so, essentially moving even farther from compliance, Kendall said:
“They could not implement the principles that were required by this legislation, mainly because of issues with Data Architecture, Data Governance, Data Management, data lineage, and related IT infrastructure.”
Common Trouble Spots
Kendall described the regulatory compliance challenge facing analysts in organizations with many different data stores and data warehouses, where acquisition of necessary information requires depending on multiple people, departments, and data sources, not all of which are automated. Data is often pulled into multiple Excel spreadsheets — all potential points of failure located on some person’s desk — “and God forbid if that person is hit by a truck,” she said.
The challenge is not only that the data is not well governed, but that the analysts themselves can’t even talk with one another cogently. In one case, a bank had 11 different definitions across the organization for a common term, primarily because their 11 different systems each defined it differently.
New Insights Through Knowledge Graphs
Kendall said that to get the answers they need to comply with regulations, business has to take responsibility and ownership for Data Strategy and Data Governance, as well as joint responsibility with IT for Data Quality and operations.
A knowledge graph can help by linking and integrating silos using terminology derived from the business architecture, providing a more flexible environment and quicker answers, while leaving existing technology in place. At the same time, she said,it allows the reuse of global standards and alignment of data sources based on the meaning of the concepts in each of the sources.
Use Case: Mapping Data to Meaning
To illustrate how a knowledge graph can provide a bridge from data to meaning, McGuinness showed a use case from a knowledge graph she created for the Child Health Exposure Analysis Repository (CHEAR). The purpose of the program is to study the impact of genetic predisposition and environmental exposure in childhood on health outcomes.
Patient data from the National Health and Nutrition Examination Survey (NHANES), genomic data from the National Cancer Institute’s Genomic Data Commons (GDC), and data from the Surveillance, Epidemiology, and End Results program (SEER) were combined with large, existing health knowledge sources, using NLP and semi-automated mapping. As a result, biostatisticians were able to use a larger population sample by combining multiple studies, therefore enabling them to draw more meaningful conclusions.
NLP and Automation Enable Widespread Use
Although the practice of using graphs to display knowledge has been around for many decades, McGuinness said that recent maturation of natural language processing technology has made it accessible to a much wider audience. Companies are using knowledge graphs much more effectively than they were a decade ago, she said.
Automated techniques, when properly combined and leveraged with the right use case, can provide an efficient way to build something scalable, and knowledge graphs can make it clear where all the pieces fit, but “It’s critical to understand what your terms mean.” It’s also important to know the reliability of the content.
At scale, manual curation is impossible, so reliance on automatic and semi-automatic approaches is required. “It becomes critical in this time-sensitive and very impactful decision-making situation to really understand where that content is, and when it makes sense to tie it together.”
Want to learn more about DATAVERSITY’s upcoming events? Check out our current lineup of online and face-to-face conferences here.

Here is the video of the Data Architecture Online Presentation: