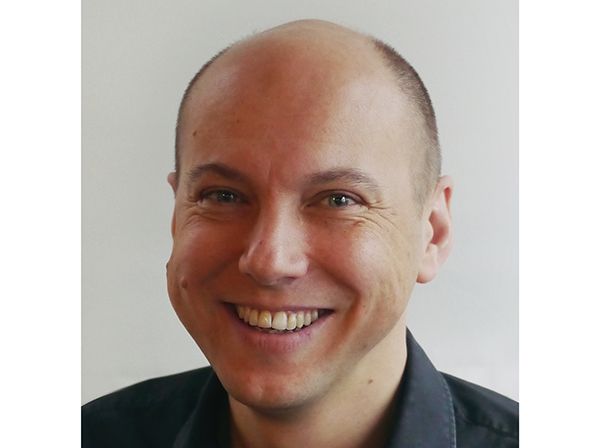
For the past year, we’ve heard about applications of generative artificial intelligence (AI) on the open web, achieving everything from writing articles and improving search to creating artwork and altering speech – some more successfully than others. But as the technology matures, we’re finally starting to see valuable enterprise use cases for generative and conversational AI. The intersection of knowledge management and AI is a particularly useful development, the need for which is undeniable. Enabling businesses to put their troves of internal data to work is a function that organizations have long hoped for. And while it sounds simple, without the help of AI, it’s nearly impossible.
Why Generative AI Now?
For starters, data is effectively useless if it’s too hard to find – and it often is. Even in rare cases in which pertinent information is not messy and disorganized, it’s still likely to be spread over various applications in text, image, audio, and other formats, not to mention over different organizational departments, teams, and individuals.
AI can analyze this data in a way that humans simply cannot. Its ability to understand, digest, summarize, and generate content at scale is unparalleled. It can perform these tasks on millions, even billions of documents in a relatively short amount of time. This not only augments human productivity, but it also leads to better decision-making in organizations. The perceived value is hard to argue with.
But the material value is even greater, saving organizations significant time and money. According to an estimate from Accenture, as much as 40% of working hours will be augmented or automated by generative AI in the future. Of course, this will vary by industry, but writing, programming, researching, and streamlining decision-making are all knowledge management areas where AI can shine.
The Challenges
Like any new technology, generative AI is not a fix-all and it’s far from replacing humans. While generative AI excels in delivering quick, contextually relevant content, there are limits to its accuracy and explainability, and it is prone to hallucinations. While this may be less problematic for recreational use, tools like chatGPT alone are often not reliable enough for enterprise use.
In fact, the aforementioned Accenture survey found that 90% of respondents believe data is becoming a competitive differentiator when assessing generative AI tools for business. Clearly, there’s an appetite for AI that meets the requirements for commercial use. But we’re grappling with the overpromise of quick, relevant, conversational answers and a slew of solutions that fall short.
Here’s why: GPT cannot answer questions specific to an organization’s internal data without being fed that information. A foundation layer that can securely connect, integrate, and search an organization’s documents while preserving user access to information sources is necessary to put large language models (LLMs) to work. And explainability is crucial for success.
This is where neural search – which provides more accurate and context-aware search results – comes in. This helps users efficiently implement semantic search to find relevant information needed to answer natural language queries, regardless of the keywords used. More relevant and transparent search results securely fed to an LLM like GPT demonstrate how knowledge management and AI can and should work in tandem.
The Benefits
You may be wondering if this is relevant for your own business. While knowledge management can provide value across industries, the ones that rely heavily on search will find enterprise AI most useful. For example, in high-tech engineering, and in the chemical, life sciences, and pharmaceutical industries where humans can’t keep up with knowledge discovery, major productivity gains will be realized.
It’s far more efficient for AI to summarize long, complex research documents and internal project documentation than it would be for a researcher to read it in full detail. But it doesn’t stop there. New generative AI-powered search can now add a layer of safety that open web tools do not.
Data safety – ensuring that the handling of sensitive data or personally identifiable information is secure and in compliance with industry regulations – is an important consideration for organizations. No one should have access to information that they wouldn’t typically. Safety also includes reliability, i.e., ensuring results are accurate, appropriately sourced, and free from hallucinations.
Getting Started
So how can enterprises get started? First, organizational leaders should start experimenting with generative AI technologies today. Focus on areas where productivity is paramount and build from there. Explore potential models and hosting environments that can be used according to an organization’s unique policies. It’s also important to take domain-specific factors into consideration, especially in highly regulated industries like healthcare or law.
The role of AI, LLMs, and GPT to make sense of vast amounts of unstructured data in enterprises is clear. Moreover, the opportunity for knowledge management professionals to drive change within their organizations is here, right now. The absence of AI means leaving productivity, time savings, money, and the ability to greatly improve enterprise search on the table. The future starts now – don’t miss the boat on the potential of AI-powered knowledge management.