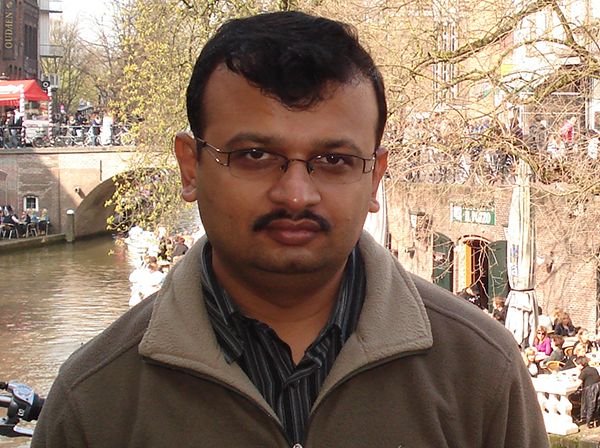
Clinical trials are critical in developing and approving new medical treatments and technologies. These trials generate massive data that needs to be managed efficiently and accurately to ensure patient safety and successful research outcomes. The good news is that advances in AI and automation technology, such as AI-based data extraction, virtual clinical trials, and predictive analytics, are making it easier for clinical trial managers to streamline their clinical trial Data Management processes and gain insights that can help improve patient outcomes.
Here are three AI technologies for clinical trial Data Management:
- AI-Based Data Extraction: AI-based data extraction automates the extraction of data from unstructured clinical trial documents, such as electronic case report forms (eCRFs), clinical study reports (CSRs), and adverse event reports. AI-based data extraction saves time and resources, improves accuracy, and efficiently handles large volumes of data. AI-based data extraction tools use machine learning and template-based methods to extract and convert data from unstructured documents into structured data.
- Virtual Clinical Trials: Virtual clinical trials leverage telemedicine, wearable devices, and other digital technologies to conduct clinical trials remotely. Participants can participate from the comfort of their own homes, reducing the need for travel and in-person visits. AI-powered virtual assistants can be used to engage with participants, monitor their symptoms and adherence, and collect data. Virtual clinical trials are particularly useful for rare diseases, where patient recruitment can be challenging, and for populations with limited access to healthcare.
- Predictive Analytics: Predictive analytics uses machine learning algorithms to analyze large datasets and identify patterns and correlations. In clinical trials, predictive analytics can be used to identify patients more likely to respond to treatment, predict adverse events, and optimize the trial design. Predictive analytics can also be used to personalize treatment plans based on patient data, improving patient outcomes.
The Benefits of Automation in Clinical Trial Data Management
Automation technology can help clinical trial managers streamline their Data Management processes and reduce the risk of errors. Here are five benefits of automation in clinical trial Data Management:
- Increased efficiency: Automation can help reduce the time and effort required to manage and analyze clinical trial data, allowing researchers to focus on higher-level tasks.
- Improved data quality: Automation can help reduce human error risk, leading to more accurate data and better research outcomes.
- Faster data processing: Automated tools can quickly process large volumes of data, which can help speed up the clinical trial process.
- Enhanced patient safety: Automation can help identify potential safety issues earlier in the trial, allowing researchers to take corrective actions quickly.
- Cost savings: Automation can help reduce the need for manual labor and improve operational efficiency, leading to cost savings for clinical trial sponsors.
Considerations in Implementing AI and Automation in Clinical Trial Data Management
While AI and automation technologies can offer significant benefits to clinical trial Data Management, there are also some challenges that organizations may face when implementing these solutions. Some of these challenges include:
- Cost: Implementing AI and automation technologies can be expensive, and organizations may need to invest in new hardware, software, and personnel to support these solutions.
- Data privacy concerns: Clinical trial data is highly sensitive and must be kept secure. Organizations need to ensure that their AI and automation solutions comply with relevant data privacy regulations.
- Lack of internal expertise: Implementing AI and automation solutions requires specialized expertise, and many organizations may not have the necessary personnel in-house.
To overcome these challenges, organizations can consider partnering with third-party providers who specialize in AI and automation solutions for clinical trial Data Management. These providers can help organizations select the right solutions, implement them effectively, and provide ongoing support and maintenance.
Conclusion
Leveraging AI and automation technologies can help clinical trial managers streamline their Data Management processes, reduce the risk of errors, and gain insights that can lead to improved patient outcomes. While there are some challenges to implementing these solutions, partnering with experienced third-party providers can help organizations overcome these obstacles.