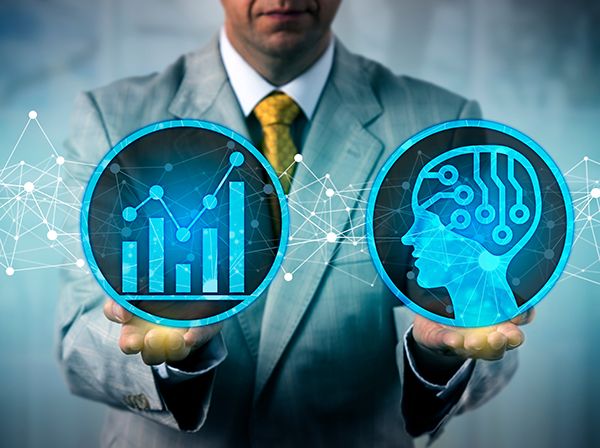
Predictive analytics and machine learning are extremely powerful tools used to predict future outcomes from large amounts of data. They help businesses gain insights, improve efficiency, and make informed decisions. With the big data space estimated to reach a value of $105 billion by 2027 (up from $37 billion in 2018), and 97% of organizations investing in big data and AI, the importance of predictive analytics and machine learning should not be underestimated. In this article, we will explore machine learning vs. predictive analytics, what each discipline involves, and how they intersect.
The terms “predictive analytics” and “machine learning” are often used synonymously, since both processes operate with similar goals, but they are not the same: While machine learning tools work by detecting and learning from patterns in data sets and can make increasingly accurate predictions over time, predictive analytics tools use predictive models to more accurately forecast events, such as the results of an advertisement campaign.
What Is Machine Learning?
Machine learning is an area of computer science in which computers discover patterns and trends in data sets. The resulting machine learning algorithms can be used for various purposes, including classifying images into different categories, predicting prices of commodities given some details, and detecting trends in the stock market.
Machine learning is an adaptive technique, where the system is designed to adapt and learn as and when new data is fed into the system. By detecting patterns in the data and continuously updating what they have learned, machine learning algorithms can solve complex problems.
Some other uses for machine learning include:
- Building recommendation systems
- Flagging errors in transactions or data entry
- Personalizing shopper experience based on browsing history
- Uncovering patterns in market research
- Automating chatbots in applications to provide users with rapid customer service on web pages
What Is Predictive Analytics?
Predictive analytics predicts future outcomes using a combination of statistical methods and processes, including advanced statistics, descriptive analytics, statistical modeling, predictive modeling, machine learning, and data mining. It is one of four main types of analytics that data analysts typically use.
The other types of analytics, from simplest to most complex, include:
- Descriptive analytics: Involves collecting, reviewing, and summarizing historical data to gauge what happened in the past
- Diagnostic analytics: Seeks to establish a cause-and-effect relationship of trends in data to identify why something happened
- Prescriptive analytics: Can make predictions about future events and advise on how to solve anticipated future problems; the priciest approach and not as widely adopted as predictive analytics
Predictive analytics includes aspects of both descriptive and diagnostic analytics, by examining historical data and figuring out the “why” of trends in that data. It goes one step further, however, by also predicting what could happen. These observations can be used to then make business or investment decisions. Predictive analytics can be used to find out when a sensor might fail or when to invest or trade stocks.
Some use cases of predictive analytics in different industries include:
- Factory owners employ predictive maintenance, where machinery health is monitored and based on the history of breakdowns, replacements are made before the machine fails.
- Health care providers use predictive analytics to understand when disease outbreaks will occur. This can then allow governments to gauge readiness and mobilize accordingly.
- Insurance providers use predictive models to analyze risk profiles and prepare payment plans for customers based on personal information.
- The sports betting industry analyzes past team performance and calculates the percentage chance of winning or losing compared to rival teams.
Machine Learning vs. Predictive Analytics: What Is the Intersection?
Machine learning and predictive analytics share many characteristics and can be used as collaborative tools, where one enhances the other. Below are a few of their similarities:
- Can be used to analyze patterns in data
- Require a large data set to be able to work effectively
- Usually used with the same end goal in mind: predictive modeling
- Commonly applied across similar industries such as manufacturing, finance, security, supply chain, and even retail
- Look at historical data to predict something about the future
Despite these similarities, machine learning and predictive analytics cannot be used interchangeably. The specific use cases for each differ, so looking at the differences between machine learning vs. predictive analytics is useful when making decisions regarding which process to employ.
Predictive analytics makes use of math and statistical models to identify patterns in data to generate predictions. Machine learning, on the other hand, works to automate predictive modeling by training with vast data sets and creating algorithms. The algorithms can detect patterns and anomalies in data. These algorithms are not explicitly told what to look for in the data.
Machine learning models are designed to evolve and learn from their mistakes as they process increasing amounts of data. These models learn from the data they process and can constantly evolve and improve their performance. Predictive analytics, however, uses only data from the past and does not evolve as more data is processed.
Machine learning is a technology that depends on algorithms to function. In contrast, predictive analytics is a practice and not a technology. It has been in use for far longer than machine learning, which is still a relatively nascent field.
Conclusion
In summary, predictive analytics is a statistical process, while machine learning is a computational process that data analysts can employ as a tool for better predictive analytics.
Whether used separately or together, predictive analytics and machine learning both provide organizations with solutions to real-world problems while also helping to boost their bottom line. Business leaders are investing heavily in artificial intelligence and machine learning to improve processes, drive insights from their data, and make confident, data-backed decisions.
Image used under license from Shutterstock.com