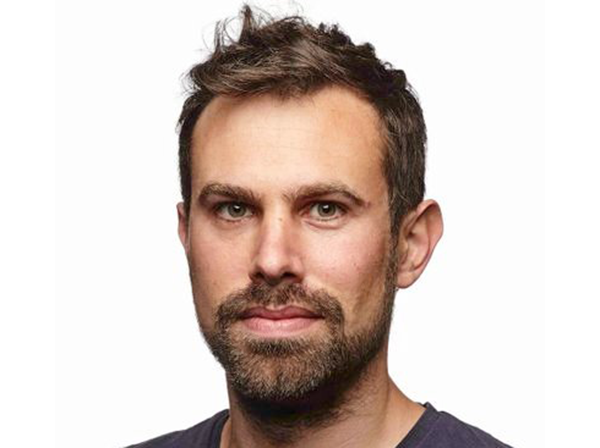
Artificial intelligence (AI) capabilities have led to groundbreaking advancements in various sectors, including banking, communications, and retail. As AI’s capabilities continue to expand, more companies are taking notice: According to a recent report by IDC, the retail industry is predicted to surpass banks when it comes to investing in AI technology. However, AI systems have yet to master a key factor in customer communication despite their powerful capabilities: the complexity of human language.
If all computers had the ability to understand human language, the way we engage with brands, businesses and organizations across the world would be completely transformed. With natural language processing (NLP), AI systems could better understand, speak, and write human language. According to IBM’s Global AI Adoption Index, roughly half of organizations globally are using applications powered by NLP, and over a quarter expect to implement them over the next year. NLP can be used for a variety of different applications – and with today’s consumers purchasing more via their mobile devices than ever before, investing in this technology is critical.
While the most commonly known applications of NLP are chatbots and voicebots, there are a vast number of additional uses for this technology such as language translation, search engine optimization, and survey analytics. As consumer behavior continues to shift towards more digital interactions, NLP will remain an important tool for any business – one that could take years to implement and perfect. However, the return on investment could be substantial if executed correctly. Here are some ways companies can better invest in NLP platforms.
Addressing Bias by Investing in Data
In 2018, Google launched the Bidirectional Encoder Representations from Transformers (BERT) model. The company recycled an architecture typically used for machine translation and made it learn the meaning of a word in relation to its context in a sentence. Then in 2020, Google pushed the BERT model architecture to its limits with an even larger network of data. By providing the algorithm with more information, the language-agnostic mT5 model can perform better than humans in labeling sentences and finding the right answers to a question.
The Google BERT model demonstrates the value of a plethora of data for AI systems. Many NLP models currently utilize websites such as Wikipedia to rely on these inputs. But like everything developed by humans, intrinsic and cultural biases are baked into these web pages and subsequently impact the information processing within the NLP system.
Strategically sourcing data can address this. Incorporating different sources provides the system with multiple points of reference when deciphering information. For example, ensuring information sourced from different cultures and underrepresented groups is integrated into the system can help offset bias.
Develop Measurable Metrics of Success
Determining your business goals is key before moving forward with integrating NLP into your business model. Your business goals should be a direct indicator of what kind of data is collected. If the objective is to have the chatbot accurately answer customer-facing questions, then a developmental goal should be familiarizing the system with sentence structure and context. If the chatbot is designed for language translation, the system should be familiar with multiple languages. Determining these factors in advance primes the system for success.
Prepping for the Future
In just the last two years, there have been more breakthroughs in NLP than in the past four decades. And yet, there’s still work to be done. Despite recent advancements in technology making AI more accessible, many companies don’t have the proper AI skills and are struggling to keep up with the complex data landscape. Yes, big tech companies have a huge advantage when it comes to machine learning and AI – because they have massive amounts of data, they have the budget and resources for software that can seamlessly process data. However, NLP can help businesses that don’t have this advantage but still need a way to efficiently process and analyze unstructured data.
Many pitfalls within NLP remain and these must be overcome before NLP reaches full potential. Some gaps in NLP technology stem from systems lacking common sense reasoning, a fundamental tool we use when communicating with each other. Lacking common sense leaves pitfalls in contextualizing a conversation, recognizing spelling or grammatical errors, and interpreting regional dialect. NLP technology shows tremendous promise – not only can it enable conversations to happen at scale, but it enhances customer engagement. This is especially critical as businesses start to shift away from high-frequency, one-way communications and toward two-way conversations.
Understanding human language is complex, but investing in and adopting NLP technology is imperative to supporting and reaching every customer on multiple platforms. And if previous years are an accurate representation of what’s to come, then businesses can count on rapid acceleration of NLP capabilities to enhance their platforms.