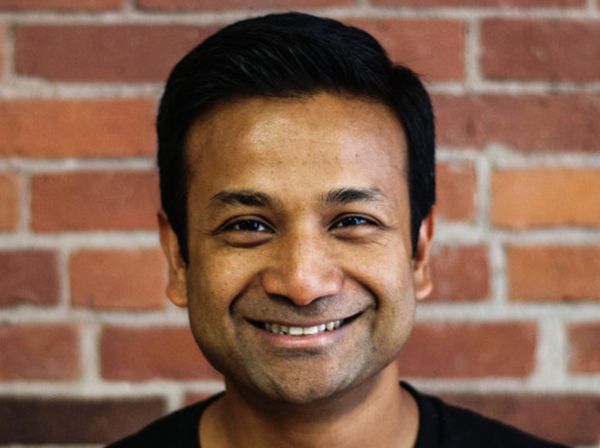
The digital industry has evolved massively and consistently over the years, often taking off in surprising directions. But one thing has remained constant: The role that data plays in driving business success has only grown. Looking toward the future, data-driven decision-making is an increasingly complex process, involving more and varied sources of data to stay ahead of the curve. To understand the value of those data sets, marketers need to understand the distinction between fast and slow data – which, when combined, can give stakeholders the rich insights they need to come out on top in the marketplace.
Understanding and Capturing Data from Digital and Analog Spaces
Marketers find themselves surrounded by data – digital signals, and those from the physical “real world.” That data comes in both fast and slow form. But marketers need to start by understanding the difference between the two categories, so they can get to work measuring their data properly and normalizing it for a single source of truth.
Fast data is designed to be readily available to the marketer, and it comes in large quantities. Typically made up of bite-sized digital signals, this type of data includes insights like email open rates, click-throughs, registrations or form fills, conversions, Google and social media analytics, and quick user polls. Marketers can find this data on-demand in a CRM, marketing automation platform, or analytics platform – all places designed to process these types of signals in real-time and display them in a way that creates meaning for the marketer. The downside is, fast data in itself lacks depth. It doesn’t reveal the underlying causes of trends beyond the inferences that marketers use to make themselves. But often, it’s the only type of data that marketers have at their immediate disposal.
Slow data, by contrast, requires considerable time and resources to gather, but sheds much more light on buyers’ intentions and pain points. Slow data is the result of businesses connecting with buyers on a deeper, more insightful level and can come from individualized customer feedback, surveys with more open-ended questions, and even one-on-one conversations. For example, whereas fast data can show you how many people and which people attended an event, slow data can offer deeper insights about why they attended the event, and the takeaways they derived.
While slow data has the power to truly immerse the marketer in the buyer journey with valuable information that fast digital signals can’t replicate, its clear downside is that it is often not practical, economical, or even possible for marketers to collect at scale on their own. And if they can accumulate meaningful amounts of slow data – either in-house or through outsourcing – it can be challenging to normalize the insights found in slow data with the signals of fast data to be equally represented and appropriately scored in the business’s CRM. Fast and slow data come in different formats that may not be immediately compatible. But it’s a challenge that must be met by the modern marketer.
In spite of the challenges in data collection and processing, the most accurate and complete view of intent is one that represents both slow and fast data signals. And in order to do that, the marketer needs to merge and normalize data – to assign the same column headers, formats, nomenclature, and scoring framework to all relevant data sets. With a consistent framework, marketers can realize the rightful scale and meaning of many varied sets of intent.
AI Puts the Marketers’ Foot on the Gas
This is where artificial intelligence (AI) comes in. AI can speed the processing, merging, and normalization of all of these data sources, so marketers may put it to work. AI algorithms demand a high level of sophistication to carry that load, though – which can be difficult for businesses to write on their own. It requires an engineering lift that businesses often have a hard time justifying. What’s more, because of the complexity of meaningful slow data – it often doesn’t come from consumers making binary decisions, and it may be structured or unstructured – these data sets will require an element of human verification. And this will likely always be the case. This is difficult and resource-draining work to conduct at scale, and it hinders many businesses in their capacity to layer this valuable data into overall intent data.
Marketers need to advocate for their teams – and the business – by pushing their higher-ups to adapt solutions that analyze slow and fast data together to drive both strategy and success. Because of the scope of this project, a business needs to partner with a data provider that can prove strong results in revealing actionable insights. Marketers should look for intent data from a provider that can pull rich, relevant data and consolidate it from multiple, diversified sources, to provide a more holistic view of intent from slow and fast data together that pays off in real performance and profits.
Know What a Strong Data Solution Looks Like
In today’s marketplace, it’s essential that businesses gain meaningful, accurate insights from robust data sets. As business becomes even more data-driven, they can’t afford to focus predominantly on easily accessible fast data. The signals that take time to reveal themselves in full, or that take place in offline settings, are just as meaningful – and frequently more so. To be competitive, businesses need to combine fast and slow intent signals to draw out lessons that can move the bottom line.
So, what does that mean, beyond the abstract? The solution should look like one single source of truth for all data sources and types. It should bring in fresh, reliable data from businesses with which the provider has strong first-party relationships. And ultimately, it should help the marketer realize what intent data truly represents: signals, both fast and slow, that have human origin and real-world context.