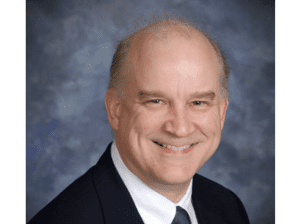
Welcome to the inaugural edition of Mind the Gap, a monthly column exploring practical approaches for improving data understanding and data utilization (and whatever else seems interesting enough to share).
This month, we start not with a gap but with a chasm – one that’s at the core of a bewildering paradox. We continue to see overwhelming numbers of analytics, artificial intelligence, machine learning, information management, and data warehouse project failures, despite the equally overwhelming availability of resources, references, processes, SMEs, and tools. In this blog post, we look at why this is happening and what we can do about it.
—
Here we are. Again.
Data is back in the corporate limelight – again. Seems we’ve been here before. In years past it’s been data warehousing, metadata, big data, and advanced analytics. “Data-driven” has been the “new” buzzword for more than a quarter-century. Now it’s artificial intelligence and machine learning. Management is recognizing that Data Quality is required to produce quality AI and ML models. For data professionals, this is another opportunity to leverage executive attention to drive information management progress.
So, we dutifully revisit our Data Governance and Data Quality plans. We get some new books and read some new articles. We package up a comprehensive step-by-step method, get head nods, and start to implement. But problems arise almost immediately. It’s too hard. It takes too long. We don’t have the resources. Before long we’re getting thanks for the good work and told that now might not be the right time. The plans are returned to the shelf and the project team is dispersed.
Again.
This lack of progress is certainly not the result of a lack of knowledge or resources. So many experts, instructors, mentors, and practitioners willing to share. We have professional organizations, references, vendors, software products, process templates, subject matter experts, consultancies, dozens of books, thousands of articles and white papers, and innumerable PowerPoint presentations and strategic plans. The technology is getting better. The processes are getting better. AI is being applied.
We know what to do and we know how to do it. Most everybody understands that it’s important and recognizes the value. You’d think that information management would be thriving everywhere. Yet, that’s not the case. We’re still fighting the same battles and we’re still making the same arguments 25 years later. And we’re still seeing the same failure rates.
Why?
Because before we can fully realize the benefits of information management, we must first have a basic understanding of the data. And the most basic understanding requires that we know two things:
1. What the data element means.
2. The values that it’s supposed to contain.
In other words, its definition and its expected content. Without those, you can’t do anything else, or at least not easily, sustainably, or at scale.
Too often we bypass data understanding and jump directly to, say, Data Quality. Everybody knows how to do Data Quality: Select a data set, examine its contents, and identify any errors and inconsistencies. Myriad tools can run the profiles and report the results. It’s even a great summer intern project. But Data Quality requires a standard against which to measure variance in the actual data content.
A simple query can tell you that a data element is populated most of the time and contains the letters “A” through “J” distributed roughly evenly. But that simple query cannot tell you whether those are the values that the data element is supposed to contain.
Artificial intelligence and machine learning are two of today’s most exciting and potentially impactful technologies, yet take for granted the existence of a foundation of data understanding that has often not been built. And without that foundation these efforts are likely to fail. Models trained using misunderstood data will yield unexpected, likely incorrect, and potentially misleading results.
In short, the benefits of information management lie at the far side of a chasm which many organizations have yet to cross. The results can be seen in the high failure rates of data warehouse, data quality, analytics, and AI/ML projects. It is a spectacularly poor track record that is too easily accepted as “normal.”
I do not believe that we have to accept that.
Data understanding is the key to success. It’s the fuel that accelerates application delivery, business and operational analytics, and AI/ML model development. It enables faster responses to changing market conditions. It facilitates communication between development teams and business units.
The challenge, of course, is making that happen. Demand exists for information management, or at least the products of information management. It’s just that nobody wants to do it. If, like so many of us, you’re starting at square one (or two) here are five steps that will help you to build a bridge across your data chasm. (You can read the full five-part Data Chasm series here.)
1. Recognize that the incentives of application development teams, and often their business artners as well, are completely misaligned from information management.
Development teams produce applications that implement business processes and capabilities. The demand backlog is always growing, and managers are pressured to deliver more capabilities in less time with fewer people. Given the choice between correcting data errors and delivering applications, we all know which will be prioritized.
Worse, the development team is disincentivized from even participating in the discovery process. Why would they go around looking for more work to do when, after all, product is moving out and revenue is flowing in just fine.
2. Start profiling some data … any data … and start communicating the results. Today.
In the absence of a management mandate or business and development teams willing to engage, then you have to be the one that does something that you’re not already doing. It is unreasonable to expect that anyone else will.
Don’t delay.
You don’t need anything fancy or automated or purchased. Pick frequently used tables and critical data elements. Write a program or script or SQL query that does a COUNT and GROUP BY. Publish the results on your departmental website or in your quarterly newsletter. Report them during your next project status meeting and post them with the minutes.
Start generating profile data and asking questions. You may discover that everything is great, and that’s great! But experience suggests that you will quickly find something interesting.
3. Focus your efforts on finding and cultivating allies in the development and business areas as well as in management.
In many companies, information management professionals have been ignored for so long that we have come to seek validation in each other, forming closed groups and developing artifacts for ourselves. As long as we focus inwardly, we will remain stuck in a cycle of quality deliverables within an echo chamber of corporate irrelevance.
Turn your efforts outward. Transform your Information Management Center of Excellence into an Information Management Center of Evangelism.
Teams might not engage, but you can find individuals that recognize or can be convinced of the benefits of data understanding to their own careers. It just takes one. You probably know someone already, and you can find more. Be creative in your outreach. Then, give them reasons to become more active in their support. Nurture these new relationships. Support your new recruits. Direct your efforts toward their data domains and applications. Make sure that they are receiving, recognizing, and communicating the benefits.
4. Critically review your processes, especially those that have already been defined and implemented.
Seek first to facilitate the work of your customers.
Too often we look to simplify our own tasks and then have the nerve to be surprised when our users don’t embrace the complicated or unintuitive solution that we present to them. I’ve found that data people tend to be good at defining process. After all, we are detail-oriented. Yet, too often our attention returns inward and perfecting the process and deliverables becomes the objective. We want the metadata and models and everything to be complete and accurate and reviewed and polished and tied up with a red bow before anybody else looks at them. We carefully map out every step, and each can be justified in the interest of metadata quality.
But would you want to use your processes? Are they intuitive? Do they answer the right questions? What if reimbursement or travel or procurement subjected you to processes like yours?
5. Incorporate information management (at minimum, expected content) into application requirements.
You’ve demonstrated value and you’ve increased the number of partners and allies. Your processes are customer-oriented and easy to use. Now, sustainability is your goal. Work with your project management leadership and the business to make information management part of their standard operating procedures. Their processes, not yours.
The point isn’t necessarily to create the task, “enter the expected content values into the metadata repository” (although that would be a good one), but rather to ensure that expected content is at minimum part of the test case definition. The details can always be extracted and stored in the metadata repository later. Eventually it will become easier to just put the details into the repository in the first place, but you need to make the barrier to adoption super-low.
I always make the following offer whenever I talk with a project team: if you want to write the data element definitions in crayon on a napkin or whatever, I’ll type them into the repository. That’s the least you should be willing to do to facilitate the work of your customer.
—
Process integration completes your bridge across the data chasm. Maybe it’s just a rickety swinging rope bridge, but it’s a start.
And now may be the most precarious point of the whole journey.
You can begin taking advantage of some of the great resources that live on this side of the data chasm, but please, please, please don’t rush headlong into new processes, deliverables, requirements, governance councils, and tools. Don’t neglect the connections that you worked so hard to establish. Continue to find and nurture new allies. Continue to focus on your customer. Success begets success.
Martin Luther King, Jr. concluded his 1960 Founder’s Day address at Spelman College with one of his most famous quotes: “If you can’t fly, run; if you can’t run, walk; if you can’t walk, crawl; but by all means keep moving.” This applies in so many areas of life. It applies here too.
Never stop. Never lose focus. And in time that rope bridge will become a highway across the data chasm.