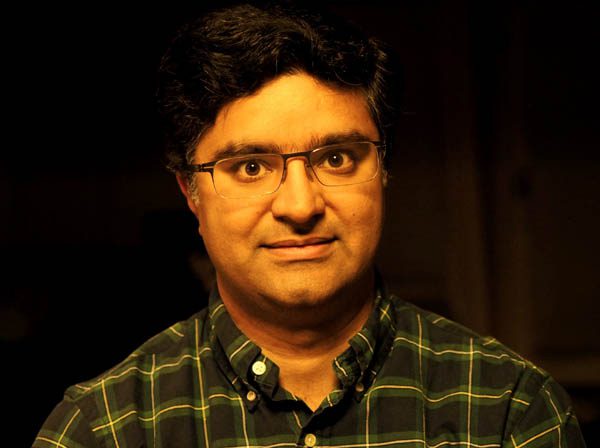
Click to learn more about author Digvijay Lamba.
Many organizations overcome a lack of data engineers by outsourcing their Data Management needs to IT service providers. Startups, small and mid-sized businesses, and even larger organizations are rich in domain expertise but inexperienced in preparing data for insights, which is why they hire outsiders to perform these vital tasks.
Although this approach offers some short-term value, the following three challenges reveal why they are inadequate in the long term:
- Loss of Control: Businesses should own the knowledge for extracting insights from data without being mired in the technical details required to do so. Dependence on service teams for Data Management prevents organizations from controlling access to their own data and data insights. Relying on these “middlemen” for this basic necessity delays time to value, impairs flexibility, and hampers productivity.
- Difficulty Scaling: Organizations must scale their data teams alongside their business, which quickly proves expensive and impractical. The more business units rely on data, the more data team members are required to match that demand. With this approach, it’s impossible to scale the business without increasing costs for data teams — which aren’t cheap, to begin with.
- Slow Iterations: The increased time to value of employing service teams makes iterations extremely slow, depriving organizations of agility and delaying time to market in today’s fast-paced, customer-centric world.
Companies can overcome these challenges — for the long and short term — by opting for pre-built data orchestration solutions that provide self-service access to the business without the need of data engineers for insights. These platforms productize the IT services necessary for capitalizing on data, eliminate the need for external parties, and significantly reduce the overhead for leveraging IT.
Characterized by a common data model for specific industries, automatic data mapping of the source data via AI, and a SaaS delivery model, this approach abstracts the technical know-how for engineering data so end-users can focus on exploiting data to better achieve business goals.
Pre-Built Common Data Models
Orchestration platforms with a common data model for specific industries like finance or pharmaceuticals minimize the technical expertise needed for assembling data for analytics or applications. With them, business users don’t have to learn to run ETL pipelines or how to code. Instead, these common data models make all data (regardless of differences in structure) conform to previously defined entities and concepts. In pharmaceuticals, for example, these might include things like doctor profiles or the patient journey. Business users simply connect to their data relating to these concepts to uniformly model them for any application.
These industry-specific common models also include various metrics and business objects in terms end-users can understand and clarify how data is used for different purposes. For example, if a pharmaceutical company were to get accelerated approval by the FDA for a certain drug, it could quickly use a pre-built commercial data model to prepare the data for a more successful commercial launch. Without this quick and painless method, they’re stuck hiring data teams to do this costly work or building their own infrastructure to do it themselves.
Automated Data Mapping
Regardless of the usefulness of a uniform data model, business users would still need to outsource their IT needs for wrangling data without an automated means of mapping it into that model. Competitive options in this space leverage multiple machine learning approaches to automate this critical step, truly making this approach self-service. Those algorithms enable end-users to map their data to the proper metrics in a no-code manner based on declarative language. Instead of writing SQL code, for example, users simply write a declarative statement about which data they want to be mapped to which object in the model.
Those statements correlate with the underlying data assets linked together in a knowledge graph that understands what those assets mean in business terms. AI does the work of actually mapping the data into the model. When integrating data for clinical trials, for example, biopharmaceutical companies look at an assortment of data from their own databases, healthcare providers, and insurance companies to understand a patient’s end-to-end journey. That knowledge becomes the basis for getting the drugs in the right hands at the right time, thus improving patient outcomes. Automating the rigors of mapping data into a common model for this goal makes the need for service teams obsolete — which dramatically reduces your overhead and decreases the risk of involving outside parties.
SaaS Delivery and Product Ownership
Many of these temporal and financial benefits come from productizing these data services via a SaaS offering. Accessing these capabilities in the cloud with serverless computing options means organizations aren’t required to purchase any infrastructure to map all their data to an industry-specific uniform model. Instead, they can begin these efforts immediately with an out-of-the-box functionality no service team can come close to duplicating. Organizations simply input their data into the model with self-service automation and get it back in a unified model ready for applications.
Finally, the significance of skipping over data teams in favor of employing a common data model for commercial analytics are huge. The questions of scale are no longer relevant, as organizations can scale as large as they want without increasing their costs. Iterations also become much faster with this method, as there’s no longer a need to wait on outsiders to do what organizations can do themselves. Implicit to this advantage, of course, is complete ownership of the knowledge of the data processing required to determine insights. Owning this aspect of data-driven processing underpins the previous two benefits while also decreasing the risk for security and regulations.
This approach represents a shift in focus that’s undeniably profound for business users. Organizations can hire domain experts and talented subject matter experts, as opposed to IT teams. Doing so enables them to concentrate on their business objectives and greatly enhances their means of achieving them, instead of just struggling with the basics of figuring out how to use data. The end result is a much greater capacity to get the best resources for fulfilling mission-critical objectives by remaining focused on business needs, instead of on IT needs supporting the former.