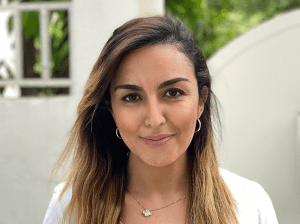
Do you know the costs of poor data quality? Below, I explore the significance of data observability, how it can mitigate the risks of bad data, and ways to measure its ROI. By understanding the impact of bad data and implementing effective strategies, organizations can maximize the benefits of their data quality initiatives.
Data has become an integral part of modern decision-making, and therefore, data quality is paramount to ensure that business stakeholders are making accurate conclusions.
But here’s the catch that every modern data leader will tell you: Data quality management is hard. It takes time and effort. Furthermore, the ROI (return on investment) is often difficult to measure.
How Bad Is Bad Data?
Bad data can lead to significant financial losses. Gartner estimates that every year, poor data quality costs organizations an average of $12.9 million. In 2022, Unity Software reported a loss of $110 million in revenue and $4.2 billion in its market cap. “Consequences of ingesting bad data from a large customer,” the company stated. Similarly, bad data caused Equifax, a publicly traded credit reporting agency, to send lenders inaccurate credit scores on millions of customers. More recently, a data incident caused huge disruption to U.K. and Ireland air traffic. It is reported that over 2,000 flights were canceled, which left hundreds of thousands of travelers stranded, the accumulated financial loss to airlines is estimated at $126.5 million.
The Implications of Bad Data
Data is at the heart of every modern business. The data team’s key responsibility is to build and maintain data products that are served to customers internally and externally, while allowing the organization to scale and meet its objectives.
When it comes to ensuring that the organization’s data initiatives are poised for success, some baseline expectations from a data team can be summarized as follows:
- Uptime: Data is a service, and therefore ensuring it is available when needed is key.
- Security: Compliance with regulations (such as GDPR or HIPAA). The team is responsible for the implementation of measures and practices to protect sensitive information and maintain data privacy.
- Reliability: Of both the data and the data platform. Part of this is covered with uptime, but also data quality and accuracy in their traditional sense.
- Scale: The data platform should allow for scalability to accommodate growing data volumes, the number of use cases, and the needs of the business.
- Innovation: Data should drive innovation, and this is an area where it is important that the data team lead by example by bringing innovation to and outside of data practices.
Achieving Data Quality Through Data Observability
Data observability is a solution to proactively monitor and maintain the health of data throughout its lifecycle. By implementing logging, tracing, and monitoring techniques, organizations gain visibility into data streams, quickly identify and troubleshoot data quality issues, and prevent disruptions to analytics dashboards. Data literacy, involving sourcing, interpreting, and communicating data, is essential for decision-makers to translate data into business value effectively. Cultivating a data-driven culture and investing in the right tools are crucial steps toward achieving data quality through data observability.
Quantifying the ROI of Data Observability
Measuring the ROI of data observability helps business leaders understand the value and benefits associated with investing in this practice. Several quantifiable metrics can serve as a starting point for evaluating the cost of bad data, including the rate of occurrence or number of incidents per year, time to detection, and time to resolution.
The impact of data quality issues can vary depending on the size and complexity of business operations. In order to assess the damage and build a strong case for a data observability solution, we propose five key metrics that data practitioners can easily implement and monitor that can be used to support a case internally:
- Number and frequency of incidents: While some companies may experience data incidents on a daily basis, others may go days – if not weeks – without one. The criticality of the incidents can vary from something “minor,” such as stale data linked to a dashboard that nobody has used in ages, to a data duplication problem causing the server to overcharge and ultimately go down (true story, Netflix 2016). We find it is often linked to: the size and complexity of the data platform, the company’s industry (some industries are inherently more data mature than others), data architecture type (centralized, decentralized, hybrid), etc. Documenting the incidents will give a better idea of what to look for next time there is one, repeated incidents are often a good indicator that something underneath needs closer attention.
- Incident classification: Not all data incidents are of the same severity; some may be minor and easily mitigated, while others can have serious consequences. Documenting the criticality of the incidents is important to ensure proper escalation and prioritization. This is where data lineage can be instrumental, as it allows the assessment of the downstream impact of the incident to better understand the criticality. An incident that is linked to the CEO’s favorite dashboard, or a production database, or an important data product is likely to be of high criticality.
- Mean time to detection (MTTD): When it comes to building trust in the data and the data team, every data practitioner’s nightmare is when business stakeholders are the first to detect data quality issues. It can really hurt the team’s credibility and the company’s ability to truly become data-driven. As you start to document the incidents and classify their criticality, it is important to also keep track of how they were detected and the time it took for the data team to acknowledge them. This metric can be a good indicator of the robustness of your incident management but also reducing it means you reduce the risk that the incident could cause more damage.
- Mean time to resolution (MTTR): What happens once an incident is reported? MTTR is the average time spent between becoming aware of a data incident and resolving it. The resolution time is greatly influenced by the criticality of the incident and the complexity of the data platform, which is why we are considering the average for the purpose of this framework.
- Mean time to production (MTTP) is the average time it takes to ship new data products or, in other words, the average time to market for data products. This could be the time spent by an analyst “cleaning” the data for a data science model. In fact, according to Forbes, data preparation accounts for about 80% of the work of data scientists. In a world where we want to treat data as a product, improving data quality can have a direct impact on reducing the time to market.
In addition to the above quantifiable metrics, others that are less easily quantifiable but just as important are worth considering when looking at the cost of bad data.
- Erosion of trust: In the data and the data team. This is, in my opinion, the most dangerous consequence of bad data, which can result in bigger issues like turnover in the data team or loss of trust in the company’s ability to become data-driven and keep up with the evolving digital landscape. And once the trust is broken, it is very hard to regain it. In a previous experience, I worked around data consumers who would rather not use data and would rather rely on “experience” and “gut feeling” in a very volatile stocks-trading environment than use it knowing that it had a high chance of being inaccurate.
- Loss in productivity: With bad data, teams are forced to firefight and correct errors as they arise. This constant firefighting is not only exhausting but also counterproductive. Valuable time that could be spent on strategic planning and growth initiatives is squandered on troubleshooting, diverting resources from more critical tasks.
- Regulatory and reputational risk: Errors in financial reporting or mishandling of personal data can result in costly fines and legal battles. Dealing with compliance issues is a significant drain on productivity, not to mention the financial burden they impose.
- Poor business performance: In addition to losing productivity within the data team, bad data can hinder overall business performance as the company struggles with digital readiness and credibility in front of its customers, and becomes vulnerable to external threats.
Data quality issues can result in various problems, including loss of trust in data, reduced team productivity and morale, non-compliance with regulations, and diminished quality of decision-making. Siloed data within departments or business units makes it challenging to gain a holistic view of the organization’s data landscape. This can lead to ineffective decision-making, hinder data culture, and jeopardize compliance with regulations like GDPR and HIPAA. Moreover, data teams can become frustrated by spending excessive time troubleshooting data issues, negatively impacting their job satisfaction and potentially leading to employee churn.
The 1x10x100 rule
The 1x10x100 rule, a widely recognized principle in incident management, emphasizes the escalating costs associated with bad data quality. According to this rule, the cost of addressing a data quality issue at the point of entry is approximately 1x the original cost. If the issue goes undetected and propagates within the system, the cost increases to about 10x, involving correction and remediation efforts. However, if the poor data quality reaches the end-user or decision-making stage, the cost can skyrocket to a staggering 100x the initial expense due to significant business consequences, including operational disruptions, lost opportunities, and customer dissatisfaction. This rule underscores the exponential impact of bad data quality, making it crucial for organizations to invest in data observability, which helps keep problems, if they occur, closer to the root cause vs. downstream.
Conclusion
Data quality issues significantly impact businesses, leading to wasted resources and missed opportunities. Investing in data observability is essential to prevent and mitigate the risks associated with bad data. By leveraging quantifiable metrics and considering non-quantifiable factors, organizations can measure the ROI of data observability and demonstrate its value to decision-makers. Ensuring data trust, promoting effective domain decision-making, complying with regulations, and fostering a satisfied data team are all critical aspects of maximizing the benefits of data quality initiatives. Embracing data observability is a strategic investment that safeguards the accuracy, reliability, and utilization of data in today’s data-driven world.
Organizations that build a rich observability practice have more visibility into their interwoven environments, which translates into fewer outages, faster issue resolution, greater confidence in their apps’ reliability – and, ultimately, more revenue and happier customers.