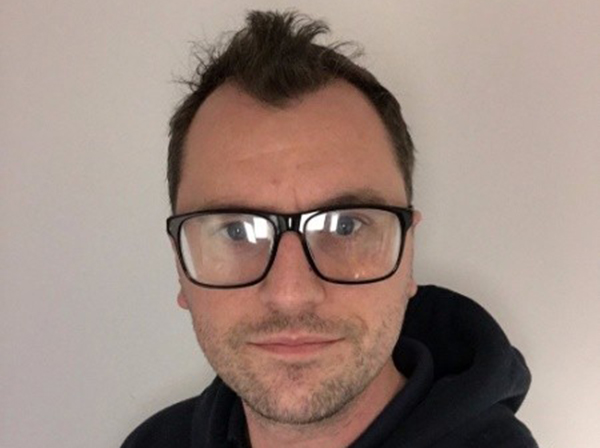
Data paradigms are changing. The concept of a data warehouse as the only solution for integrating data sources should be questioned. This approach is increasingly at odds with the realities of how data is transacted and used in enterprises. Instead of a few data sources, there can be 20, 30, 40, even more. Harmonizing and accessing that data from a single source is becoming more complex, costly, and inefficient. This leads to a fundamental question: Has the architecture of the traditional data warehouse become an obstacle to achieving the vision of the data-driven enterprise? Is it a fallacy that data warehouses solve all enterprise data integration problems?
It seems slightly heretical to me – an engineer who has worked in data warehousing for virtually my entire career – that I’m even posing this question. My answer is that it is not a fallacy. It can be done, but it presents a lot of challenges. Organizations need to make sacrifices, and the result is often better in theory than it ends up in practice. There are technical and human challenges to developing a data warehouse, and the human challenges are often greater than the technical. The effort to meet business expectations can result in data warehouse projects costing $200 million or more and taking as much as 24 months to complete.
That said, the advantages are significant. Data warehouses allow organizations to bring massive data sets together from many disparate sources and systems for analysis by AI and enable valuable (and often hidden) business insights. For example, it wasn’t until one of my enterprise customers built a data warehouse and correlated the data from all its source systems that it realized, due to a particular component of their best-selling product, they were losing money on every sale. Once this came to light, they saved themselves millions of dollars. Fantastic, but benefits like these come with a cost.
To determine the best data integration strategy, the most expedient way is to work backward from the problem you’re solving. Determine the question, then decide and build the architecture that answers that question and best supports the organization’s data needs.
A Matter of Risk Assessment
Data warehouses are both a challenge to the organization and a challenge to develop. They take months, even years to complete and deliver.
Much is built on top of a data warehouse to make it useful for business. The biggest sacrifice in this process is agility in favor of the utopia of one version of the truth for the whole organization.
It becomes a matter of risk assessment; specifically, how much tolerance an organization is willing to absorb. Safeguards and multiple data paradigms erode an organization’s ability to be agile with reports and self-service business intelligence applications. The more safeguards and slower the data structures, the more difficult it is for those kinds of tools to find the information they need. Enterprises can’t have self-service business intelligence that will be successful if the data is three months old or requires significant lead times to add a transaction. There’s a balance to be had.
By definition, a data warehouse is a data storage system that conforms and homogenizes multiple sources of data, but it’s not that simple. It’s rare to achieve the same data from different sources – harmonizing and accessing that data is becoming more complex, inefficient, and costly.
ERP systems are critical to making data useful and timely, but different ERP systems produce very different results. Data warehouses don’t match the granularity of those transactions because they can’t. Data consolidated into a data warehouse is not a bad thing, but there is a time and utility cost. Organizations need to pick their data paradigm based on the decision to select what’s most important and valuable to their enterprise: quick access or a consolidated single source of truth.
There is almost always a harmonization of master data in a reporting hierarchy, but the data is always changing as it’s brought together into a single report across multiple feeder systems. In a data warehouse, it’s designed to move towards that one source of truth to whichever is the organization’s database management system of choice; but again, that’s changing too.
The Benefits of More Diverse Data Integration
Enterprises are moving their data architectures to cloud-based and more flexible, heterogeneous data structures. Part of this relates to the enterprise’s bottom line. Enterprises want out of the hardware business. They are looking to invest in more flexible, consumption-based, subscription models that allow them to pay for data they use – and not for data they need to store on-prem.
Enterprises realize that there are no monolithic, one-size-fits-all ERP systems, and even if organizations have only one, an acquisition, merger, or other transformation will almost certainly result in more. When it comes to data being useful for strategic decision-making, it’s all about speed and accessibility. And the reality is that it’s not easy to penetrate and write reports against the big ERP systems. Enterprises are gravitating towards cloud-based, best-of-breed solutions, such as Salesforce, Concur, and Workday, that provide insights in just days instead of weeks or months.
For strategic insights, a mix of sources and solutions may be better than the benefits they may gain from one huge data warehouse or ERP system. Reporting can be quicker and more useful. While it doesn’t eliminate all risk if the data sources only provide 70% of the data answers, it minimizes that risk to an acceptable level.
Another benefit of a more diverse data integration solution is the fact that the larger the data warehouse, the more difficult it is to manage. There is also the investment loss when moving a large data warehouse to cloud. If there’s one thing we’ve learned over the past 20 years in tech it’s this: It changes more quickly than we do.
Organizations can spin up a database in cloud today to do what they want in just minutes. If it works, they can keep it. If it doesn’t, they can shut it down just as quickly and lose just one day’s cost. There is no need for expensive, inflexible hardware. Everyone is coming to realize that.
“Data Warehouse of Need”
So, what would I consider a better data integration strategy? I believe it’s one that involves multiple systems and sources that provides enterprises the answers they want when they need them. After all, what’s the point of having data if it can’t be monetized in some way?
Some call this concept a data lakehouse. I prefer the term “data warehouse of need” because the phrase “data lake” muddies the water. It suggests a data storage paradigm and not consideration on a case-by-case basis. Whichever we call it, it works because there’s not a single unifying structure but there is unification where unification is required. It’s a cloud data warehouse that uses cloud-based data to spin up databases for specific client needs, analytics, and real-time decision-making on the fly.
Is that the last word in data integration? In my experience, there is no silver bullet, but there are exciting developments happening regularly and with cloud, augmented business intelligence, and machine learning, solving data problems is more fun now than ever before.