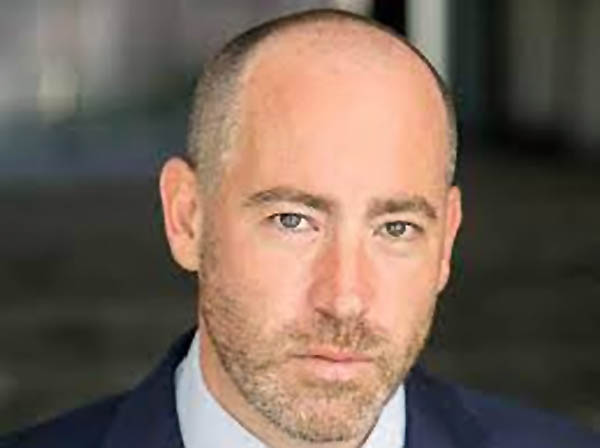
Click to learn more about author Assaf Egozi.
Modern organizations house a growing number of “citizen data analysts.” These individuals hold a wide range of positions in the enterprise, from executive and business roles through to data, business, operations, marketing, and sales analyst roles. They work across a wide range of functions, striving to improve insights into an organization’s products or services, better understand customer requirements, identify competitive threats and market opportunities, optimize resources, or drive other process efficiencies. But the one thing that they all have in common is the need to use data as a core part of their business workflows and decision-making processes.
To do their jobs effectively, these analysts need the right operating environment. Understanding the business is crucial. That typically means they are embedded within specific business units rather than organized as centralized teams. This provides them with much deeper insights into the challenges faced by each unit, as well as “skin in the game” when it comes to solving those challenges.
In addition to developing a detailed understanding of business challenges, they also need to be supported by the right information and tools. While most analysts are highly competent with numbers and well versed in at least the basic forms of statistical analysis, they are mostly neither technologists nor data scientists. Rather than write code, they are typically more comfortable with user-friendly data analysis applications such as spreadsheets and visualization tools. For the most part, these relatively simple applications have served them well for the last few decades.
However, while spreadsheets and BI tools continue serving a vital function, analysts are increasingly running into limitations that can be solved only by a new generation of analytical tools.
What’s Driving Demand for AI/ML?
The term “big data” may no longer be hyped, but that is not because growth in data has diminished. Rather, the ongoing data avalanche has simply become the new normal. With an ever-greater variety of data being generated from more sources, at faster velocities, and in larger volumes, organizations need to scale their ability to collect, process, and analyze that data.
AI/ML offers tremendous capabilities in each of those areas. Machine learning models are ideal for quickly interpreting data in real time (addressing data “velocity”), processing unstructured data (addressing data “variety”), and scaling to spot patterns, detect outliers, or generate predictions across very large datasets (addressing data “volume”).
The fact that AI/ML algorithms are particularly adept at processing the velocity, volume, and variety of data available to modern analysts, while traditional approaches and applications struggle, is one of the biggest drivers for AI/ML adoption.
Overcoming Barriers to Adoption
While AI/ML offers great promise, there is one major impediment to its widespread adoption at the analyst level: Most individuals simply do not have the requisite skills to develop algorithms from scratch. Neither should we expect them to. Analysts are hired for their business acumen, data savviness, and communication skills, not for their ability to write code.
As such, the adoption of AI/ML by analysts needs to be supported by a platform that is specifically designed for their use, not by trying to force them to become data scientists.
What would such a platform need? At its core, it would need to offer four key capabilities:
(a) simple integration with input data sources;
(b) a wide collection of preset data enrichment and analytics blocks;
(c) the ability to connect blocks into customized data workflows; and
(d) an automation engine to allow for scheduled data runs.
What about an integrated spreadsheet or visualization component? Most analysts strongly prefer to keep using their own spreadsheets and visualization tools, and so rather than creating a new BI tool, the AI solution should simply allow for simple integration with existing solutions. Equally, many organizations will already have invested in data warehouses and/or data lakes to aggregate enterprise data, so the ability to easily work with existing data stores is also key.
Another potential impediment to the adoption of AI/ML is fear that these technologies will displace jobs. In that sense, it is important to note that the goal of AI/ML should not be to substitute the role of analysts but rather to enhance their abilities. Some enterprises mistakenly believe that the main role of AI is to provide prescriptive analytics, essentially replacing the person-in-the-loop. This couldn’t be further from the truth. While prescriptive analytics can definitely be used where appropriate, most business processes should leverage descriptive and predictive analytics to help analysts synthesize masses of data, leaving human experts to conclude final recommendations.
Enhanced Intelligence for Next-Gen Analysts
Analysts should ideally see AI/ML as an extension of their own brains. The Wikipedia definition of a cyborg is “an organism that has … enhanced abilities due to the integration of some artificial component or technology.” By that definition, analysts have been cyborgs for several generations. Spreadsheets have long enhanced our numerical processing abilities, allowing us to build models quickly and easily. But recently they have increasingly run into limitations.
AI/ML has great potential to further enhance the role of technology in business analysis. But to do so effectively, it must be easy to use, enable orchestration of data/analytics pipelines, and integrate with existing tools. With those conditions met, AI/ML promises to open up a multitude of new use cases. In a follow-up blog post, we will explore those use cases in more detail.