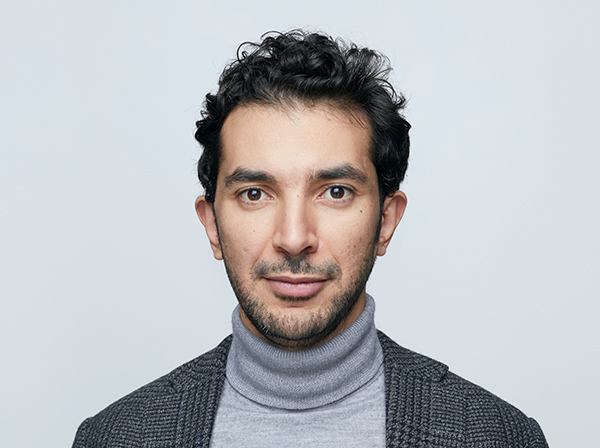
Synthetic data has emerged as a technological solution for organizations struggling with data access and privacy compliance. As a privacy-enhancing technology, it has grown in popularity over the past years, with new predictions forecasting that by 2024, 60% of data used for AI and data analytics will be synthetic.
But what is the reality of synthetic data adoption? What concrete applications do we see in 2022? This article shares the learnings and perspectives gained from four years of supporting the integration of synthetic data in organizations.
Innovation begins with data. Organizations that can utilize the right data in the right way can benefit their users and provide better services. However, data projects come with many challenges. Hindered by the talent gap, ROI uncertainty, data availability, inconsistency, or biased datasets, data initiatives are often doomed before they can even start.
And let’s not forget the ever-changing global regulatory landscape and privacy risks associated with data projects. When it comes to working with data on a global scale, companies based in multiple countries and subject to multiple jurisdictions may find the complexity of data regulations to be one of the most challenging aspects.
So how can synthetic data help solve these challenges? In the next paragraphs, we will focus on several use cases in finance, insurance, health care, and telco industries.
But first, let’s take a quick look at the technology itself.
What Is Synthetic Data?
Synthetic data is an outcome of artificial data generation. The new dataset resembles the quality of the original data and retains the statistical distribution. This means synthetic data looks like and behaves like real personal data.
Synthetic data is defined by two aspects:
- It’s artificially generated. In other words, synthetic data has no direct relation to real events or real people. These datasets are generated artificially by algorithms.
- It is realistic. Artificial data that doesn’t mirror original data is not useful for analysis.
Synthetic data can be created in two ways:
- Based on the previous knowledge – if you know the laws that govern your model, you can use them to generate and simulate new data. For example, if you have customers over 20 who are female and have certain characteristics, you can use that knowledge to artificially simulate data points.
- Created directly from the real data, which is usually accomplished by using machine learning or artificial intelligence algorithms that learn the distributions and the relationships within the original dataset. Once the relationships are learned, you can create new records.
In many industries, the majority of data initiatives focus on internal data, but in other industries such as health care the focus is on external collaboration and external data. Synthetic data helps with both, and its use cases revolve around product development, gaining access to testing data, validating external vendors and partners, and more.
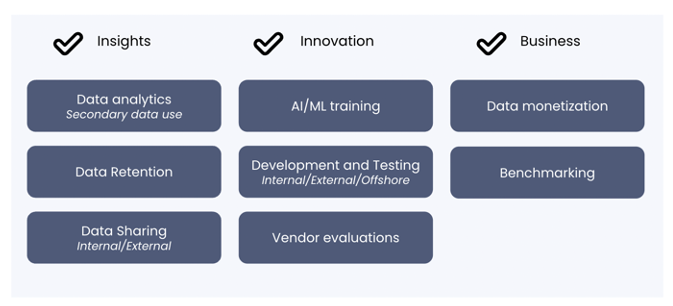
Enabling Granular Data Sharing in Health Care and Pharma
Perhaps more than in any other sector, health care relies on access to and analysis of data for research and innovation. The ability to use granular, statistically representative data is essential.
In addition to being one of the most regulated industries, health care and pharma are also faced with difficult issues like data access, patient privacy, and mitigating the risks of data breaches and siloed information.
Synthetic data do not contain any personal data and do not require additional patient consent, thus opening up a world of new possibilities. The flexibility of this data type can drive innovation and help companies understand patients and diseases in completely new ways:
- A synthetic data analysis can contribute to faster disease or drug discovery, a more personalized approach to patient treatment, and improved patient outcomes.
- Data science teams can use synthetic data as a foundation for clinical trials, when real data isn’t available or such data is scarce.
- In many cases, machine learning models need high-quality data that comes in large samples, which is usually extremely hard to obtain. The use of synthetic clinical health data can be of great importance when training machine learning models.
- Access to patient data is a real problem for the health care industry. Patient data samples are often too small or difficult to use. With underrepresented patient groups, synthetic data can be used to complete existing datasets and improve data accessibility.
For example, Roche, a Swiss multinational healthcare company, already tested synthetic clinical trial data for training machine learning applications. Another company, Newsenselab, leveraged synthetic data for advancing migraine research while preserving patient privacy.
Facilitating AI-Driven Data Agility in Insurance
With changing practices, new demands from consumers, and intense competition, insurance companies are compelled to make the most of the data they collect. Their ability to do so is constrained by data security and privacy regulations as well as legacy and siloed systems.
As regulations and customer demands of data privacy and security continue to rise, privacy-preserving synthetic data can help insurers address some of their data processing challenges.
Most tabular customer data in the insurance field can be synthesized, including claim data, sales and churn data, as well as market and survey data. It’s an opportunity to identify, develop, and test new products that answer customers’ needs from data that complies with the strictest privacy and legal frameworks:
- By enabling real-time and secure exchange of information across departments and jurisdictions, the customer journey can be improved to increase conversion rates.
- Improves underwriting precision using accurate statistical insights to model risk or anonymized metadata.
- Scales AI and takes advantage of cloud technologies with data assets that comply with governance and security requirements
- Strengthens fraud detection systems with large volumes of data to train detection models.
- Improves accuracy of claim predictions with data patterns to identify risk group characteristics.
Synthetic insurance data is already being used by European insurers. In Switzerland, insurance company Die Mobiliar validated the use of synthetic churn data in the context of data privacy protection, adding a new tool to their digital transformation toolbox. Public authorities in Europe are also investing in synthetic data, like in the U.K. and Germany. And Gartner is ranking it as a forward-looking privacy technology for the coming years.
Long-Term Data Retention in Finance
The finance sector has received more EU General Data Protection Regulation fines than any other industry. Non-compliance involves not only fines settlements but also business disruptions, productivity loss, and revenue loss.
In addition, legacy systems with proprietary formats and siloed IT infrastructures prevent data teams from quickly accessing data due to prolonged data access processes.
Moreover, even when data is available, its quality might not be sufficient for cutting-edge applications.
To preserve the privacy of their data, financial companies can use synthetic data.
Compliance with personal data processing regulations is a guarantee for enterprises, making it a crucial asset. A bank, for example, would have to delete all personal information and financial information after a customer contract ends to comply with GDPR data retention requirements.
By using privacy-preserving synthetic data, the enterprise would be able to run the long-term analysis on the synthetic financial data generated during the contract period, and delete the customer information as required by applicable regulations.
The largest companies in the world are starting to work with synthetic data. Amazon is already using this technology to improve customer purchase prediction. American Express is also exploring the topic. The data teams are researching synthetic data to train machine learning and improve their fraud detection algorithms.
Enhanced Analytics in Telco
Data retention is a constraint in the telco industry as well. Most regulations require that data be deleted after a specific duration. As a result, it is almost never possible to produce an analysis that covers a longer period of time.
For example, a company that wants to understand seasonality would need access to at least two years of data. A data retention policy renders it difficult to understand why your customers churn or go to your competitors, or to analyze the long-term impact of decisions.
In this case, synthetic data can be used to create a pipeline of synthesized customers based on learnings derived from the original data. The original data patterns will be preserved with synthetic customer data without retaining any specific information about the customer. A company can use this data for almost all forms of analytics without having to retain the original data.
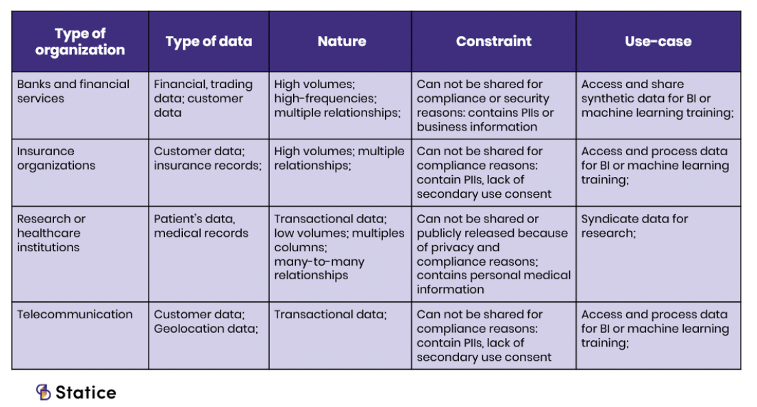
How to Successfully Integrate Synthetic Data in Your Organization
We noted at the beginning of this article that many data projects remain at the idea stage. The success of synthetic data integration requires teams to plan and take into account a variety of factors:
- Involve all stakeholders from the beginning: Business, data and analytics, IT, legal, and privacy must have a voice in the project and understand each other’s concerns and requirements.
- Determine all relevant use cases that relate to the big picture. However, start small and figure out how to measure the success of your synthetic data integration.
- Synthetic data is an emerging topic and adoption is still in its infancy. Therefore, when you choose a synthetic data vendor, invest time in training and developing the capabilities, making sure your team understands what this technology is, its limits, and its true potential.