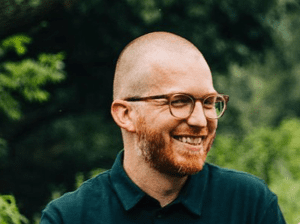
Artificial intelligence (AI) has the power to change the global economy and potentially, one day, every aspect of our lives. There are numerous possible uses for the technology across industries, and new AI projects and applications are frequently released to the public. The only restriction on AI’s use appears to be the inventiveness of human beings. AI workloads will undoubtedly be crucial in industries such as health care, finance, and leisure. But this begs the question: How can these critical AI applications be maintained without downtime, and how can the underlying data be secured without compromising its mobility?
Always Keeping AI Data and Workloads On
Many firms rely on the tried-and-true backup method to ensure safety and security against data loss and outages. This makes sense in terms of general data protection. However, backups are not the best method for disaster recovery and business continuity, particularly when it comes to the most critical data and workloads. Backup’s main shortcoming is that it can only safeguard individual servers – not entire programs. It is necessary to manually rebuild the programs from their separate components after recovering data from a backup. Restoration can take days, or even weeks, which is often an unacceptable amount of time. To ensure that vital AI applications are always available, businesses need advanced solutions that can recover data more quickly.
A growing number of businesses are using disaster recovery (DR) solutions to expedite the recovery of their most important workloads and data. Right now, the best recovery option is continuous data protection (CDP). When using CDP, every change to data is immediately documented in a journal as it’s written. CDP enables quick and easy restoration of data to the state that existed just moments before an attack or disruption without significant data loss.
The Lowwest Possible RPOs and RTOs Are Critical for AI Applications
To achieve the lowest possible recovery point objectives (RPOs) and recovery time objectives (RTOs) for crucial AI applications, near-synchronous replication provides the best of both worlds: the great performance of synchronous replication without the significant network or infrastructure demands it requires. Near-synchronous replication is comparable to synchronous replication – although it is technically asynchronous because data is written to several locations simultaneously, except for a brief lag between the primary and secondary locations. Near-synchronous replication is always on and constantly replicates only changed data to the recovery site within seconds. Because it is always on, it doesn’t require scheduling or using snapshots. It writes to the source storage without having to wait for the target storage to acknowledge it. One of the key benefits of near-synchronous replication is that it offers strong data availability and security at quicker write speeds than synchronous replication. Because of this, it’s a solid option for workloads like AI applications with a lot of data or heavy write loads.
AI Data Mobility Can Be a Major Problem for IT Infrastructure
AI is data-driven. The amount of AI data in existence is exponentially greater than anything IT has previously encountered, and the scope represents a totally new age of data generation. Exabytes of raw data are needed for even basic AI applications, which must be prepared for model training and subsequent inference. The data sets are frequently created on the edge and must be moved into a central data repository for processing. Additionally, the data needs to be stored for possible re-training at the end of its lifecycle. The need for continuous movement of enormous volumes of data has created new issues for IT infrastructure and management: Today’s network technologies and synchronous replication-based data management solutions aren’t equipped to lift and move these massive data sets. To move AI data with limited processing power and bandwidth, asynchronous replication is required. This guarantees block-level, continuous replication at low bandwidth, preventing significant data transfer peaks.
CDP and Near-Synchronous Replication Will Play Key Roles in AI’s Future
When many think of AI, they might first think of trendy use cases like AI chatbots or image generation. However, there are many ways that AI is currently being used and will be used to more broadly benefit humanity and society.
In addition to many other incredible use cases, AI will soon be able to assist us with disease diagnosis, cancer cell detection, autonomous vehicle driving, traffic jam resolution, multilingual translation, energy consumption optimization, crop disease detection, and climate, air, and water quality monitoring. Since these applications greatly benefit people and the world around us, it is imperative that they are safeguarded using the finest technologies currently available, such as CDP.
Simultaneously, the size of AI data poses a significant challenge for current IT infrastructure to store, manage, and transfer the massive volumes of data. Due to their size, AI data sets will require data mobility that current technology cannot offer. It will be necessary to implement new data mobility technologies in order to successfully manage AI data.