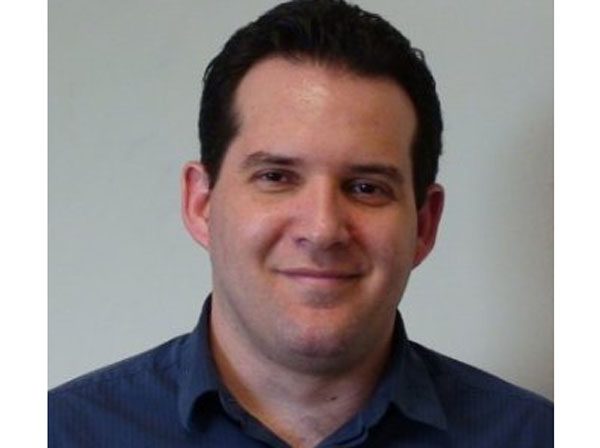
Last year, I wrote about my top four predictions for natural language processing (NLP) in 2021. As we approach 2022, a lot has happened in the world of artificial intelligence (AI) and machine learning, and NLP is behind a lot of that momentum. Growth has continued steadily, with 90% of tech leaders indicating that their NLP budgets have increased at least 10-30% over the last year.
While the pillars outlined in my previous article touch on some of those contributing growth factors, it’s interesting to look at what has come to fruition and what’s next for NLP. Most notably, low-code solutions have been a big topic of interest here. Although they still require programming skills, low-code applications have lived up to their promise of accelerating software development through pre-written code. According to Gartner, 65% of application development will be low code by 2024.
Low code is living up to its expectation, which is great news for overburdened data scientists. But simplifying workloads for programmers is only half of the equation. When you consider the worldwide AI skills shortage and the pace of business, easier-to-use solutions are helpful, but they’re not a fix-all. To really democratize AI and NLP, we’ll need to shift the conversation from low code to no code.
Simply put, no code requires no previous Data Science education or coding experience to put models to work. This shift from technical worker to domain expert puts the ability to code in the hands of businesspeople in the way that Excel or Microsoft are available to them. Beyond lowering the barriers to entry for AI, no code enables a level of interpretation of results that can help refine models.
For example, if an NLP model is trained to identify the optimal candidates for a clinical trial for a new COVID-19 vaccine, you’d want a medical professional to weigh in – not just a data scientist. Beyond health care, we’ve seen this trend evolve in other industries too. Building a website used to be a major software engineering project – now it is primarily a graphic design project. The shift from data scientist to domain expert will be gradual, but we’ll see a lot more easily applied no-code options to facilitate this transition in the coming year.
In the vein of making AI more accessible to the masses, I also predicted that more multilingual solutions will hit the market this year, making it easier for speakers outside of English and Mandarin to take advantage of NLP. While multilingual offerings have become more available, multimodal techniques will steal the spotlight in the coming year. Multimodal language models enable “shortcuts” for many languages for which there isn’t a large body of curated labeled data, or even a large body of written digital (i.e., low-resource languages). We are now able to bring state-of-the-art language understanding to many of them.
In a clinical setting, for instance, NLP can help scan text in Electronic Health Records (EHR) to find trends among patient populations. But not all pertinent information is stored as text. Diagnostic imaging is an important part of the comprehensive patient profile, and to accurately interpret those images, you need computer vision. As such, multiple modes of technology – in this case, NLP and computer vision – are far more effective together than when on their own.
Between streamlining Data Science and using techniques that augment the strengths of multiple AI technologies, my final prediction should come as no surprise. In 2022 we’ll see a lot more large-scale NLP deployments because we’re simply getting better at tuning models and extracting more accurate and actionable results.
Models degrade over time and behave differently in different production environments. For example, an NLP model created to identify toxic content online will not perform the same when applied in a health care setting. Even when graduating from research to a real production environment, technologists have to account for differences in performance and tune models accordingly.
Constant monitoring and tuning will be part of AI and NLP deployments for the foreseeable future, but who knows what capabilities we’ll have in years to come. In the meantime, let’s see where no-code solutions, multimodal techniques, and more successful deployments of NLP take us over the next 12 months.