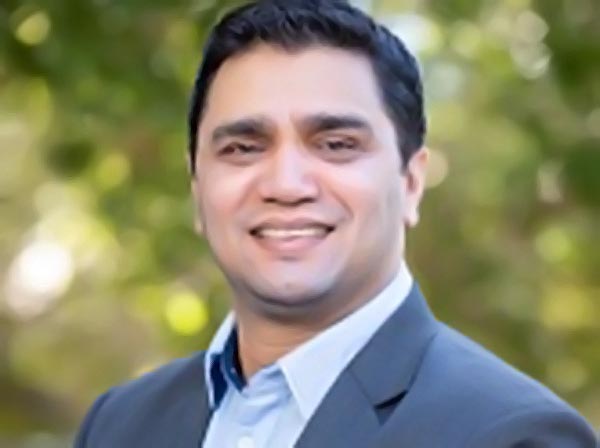
Click to learn more about author Brahmajeet Desai.
With data flowing in from a countless array of applications and devices, extracting insights from the data lake and making them available to business users in an easily can be quite a daunting task. If you moved to a Hadoop or cloud-based Big Data platform with the expectation that it will provide better insights, you would like to bring the benefits of Big Data Analytics to business users as seamlessly and transparently as possible.
However, one of the key challenges of working with Big Data platforms is that it is difficult for users to access and manipulate data. Plugging in existing BI tools onto the Big Data platform leads to several performance issues. Traditional BI tools are designed to work with relational database management systems (RDBMSs) and use SQL for querying data. When connected directly to the Big Data platform, query performance degrades significantly as running interactive SQL queries on large datasets is an extremely time-consuming process.
Though most traditional BI tools have gone through several cycles of enhancements to improve their ability to deal with Big Data, they are still unable to deliver insights at a speed that matches the expectations of today’s fast-moving business environments.
OLAP on Hadoop for Instant Analytics
Online Analytical Processing (OLAP) is a tried-and-tested concept that has been around for over two decades in the world of Business Intelligence. Before the Big Data revolution, it helped users perform multi-dimensional analysis and interact with their business data in meaningful ways. However, conventional OLAP technologies fail to work in the Big Data world as they cannot deal with the massive volumes of data, the explosion of cardinality and dimensions, and the large variety of data sources. They need a complete makeover to make them work on Big Data, and that’s how the concept of OLAP on Hadoop emerged.
OLAP on Hadoop solves the problems of speed and scale associated with Big Data. It involves creating multi-dimensional cubes on massive volumes of data using the unlimited storage and processing power of Hadoop. These cubes can serve complex queries instantly and enable quick analysis of Big Data. Since data retrieval is fast, this technology is optimal for slicing and dicing operations on large datasets.
The OLAP cubes can be easily accessed by traditional BI tools such as Tableau, Qlik, Microstrategy, Excel, or any other preferred tool, making it easy to access and visualize Big Data. As a result, the OLAP layer becomes transparent to the end users, and they can analyze their Hadoop data seamlessly without being constrained by its size or complexity.
The Relevance of OLAP
The ability of OLAP on Hadoop technology to handle multiple dimensions and enable interactive analysis makes it more relevant for businesses today than ever before. As the complexity and volume of data increases, OLAP facilitates in-depth analysis by serving complex queries instantly. You can analyze data across as many dimensions as you need and gain deep insights into the actual problem or an issue.
Imagine having data with hundreds of billions of rows and dimensions with cardinality in the hundreds of millions, and getting a response to your queries within seconds. That’s the power of OLAP on Hadoop. High performance, interactivity, and ease of access are the key factors that influence the usage of Big Data for day-to-day decision-making and help OLAP create a phenomenal impact on the success of the modern business.
Tips for Evaluating your OLAP on Hadoop Solution
OLAP comes in many flavors – ROLAP, MOLAP, and HOLAP. The ROLAP (Relational online analytical processing) methodology fetches data from a relational database using complex SQL queries and creates a dynamic multi-dimensional view for the user. It can handle high data volumes but slows down for complex queries. In the case of MOLAP (Multi-dimensional Online Analytical Processing), aggregation is done in advance to build multi-dimensional cubes that deliver better performance. However, the success of a MOLAP solution depends on the way it handles the combinatorial explosion that happens while processing Big Data. HOLAP or Hybrid OLAP is the middle approach that combines the advantages of both MOLAP and ROLAP. Each method has benefits and limitations and should be evaluated to match your performance expectations and the complexity of your queries.
You need to understand where your OLAP on Hadoop solution processes and builds cubes – in-memory, outside Hadoop, or within Hadoop. In-memory solutions have their limitations and moving data out of Hadoop to another platform for any processing leads to performance and security issues. Only solutions that leverage the compute and storage capacity of Hadoop for OLAP for building and storing cubes can achieve consistent performance and deliver high scalability.
Also, find out how your OLAP solution handles incremental data. A high amount of reprocessing for additional data can be an expensive and time-consuming exercise. It can also lead to latency issues. Besides this, consider factors such as cube designing interface, access mechanisms, caching methodologies, and security protocols while evaluating your options.
To summarize, the possibility of using OLAP for BI on Big Data can change the way organizations access and use their data. However, all OLAP solutions are not the same. To ensure that you get the promised benefits of OLAP on Big Data, choose the solution that can meet your organization’s analytical needs.