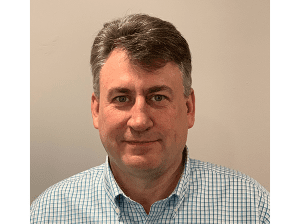
They’ve passed SATs, graduate records exams, and medical licensing exams, and programmers have used them to solve obscure coding challenges in seconds. Undoubtedly, generative AI chatbots’ capabilities are astounding, but this doesn’t mean they get it right every time.
Despite their success in providing contextually relevant and, for the most part, accurate answers, can generative AI truly replicate human conversation? The short answer is no, but it comes pretty close.
Generative AI chatbots, powered by large language models (LLMs) like OpenAI’s ChatGPT and Google’s Bard, lack human nuance identification and critical-thinking skills – vital when giving financial advice or handling personal health, for example. Yet, OpenAI points out that fixing hallucinations and ethical decision-making is difficult because there often isn’t a source of truth in the training data.
Still, with explicit prompts, carefully governed training data, and validation techniques that vary by use case, reliable human-like interactions might not be so far off. Let’s take a closer look at what teams using AI can do to deliver more human-like conversations in real time.
Determine Human Intent
For generative AI chatbots to provide relevant and helpful responses, they must understand and accurately address user intent.
Ambiguous user questions were less of an issue with traditional chatbots that offered users a limited “menu tree” of questions. In contrast to the predefined set of options, generative AI models are freeform; you can ask them anything, which can cause problems. For instance, understanding sarcasm, irony, or humor requires understanding social context, and a simple comma can make all the difference: “Did you eat my friend?” Or, “Did you eat, my friend?”
Explicit prompts, therefore, are critical to enhance the effectiveness of chatbots. Users must consider words with multiple meanings and whether the context could be taken in numerous ways.
One solution brands can offer to support users with intent is asking the user two or three questions before generating a response. This could be an effective way to not only drive intent but also guardrail against providing unhelpful information.
Understand the AI’s Knowledge Set
Another strategy to enhance the human-like nature of interactions with AI chatbots is by connecting them to the most appropriate knowledge set.
There are various LLMs, as well as open-source tools, available for developers to integrate with their chatbots. This includes vertical-specific AI datasets for industries like healthcare that contain specialist knowledge – beneficial for startups with limited owned data. Data quality directly impacts the AI model’s performance, so with intricate use cases, training models on specific medical data, such as scar tissue, COVID-19, or other patient symptoms, is essential.
Within the LLM provider, there are also variations of the dataset. For example, a company might use OpenAI ChatGPT-3.5 for first-level responses. Still, if ChatGPT-3.5 doesn’t provide adequate information, they may use ChatGPT-4’s more advanced and broader training data set.
Evaluate Your Use Case
How well AI can replicate human interactions will depend significantly on the context in which it is used. For example, human intervention and oversight are critical when using AI in highly regulated industries or those involving significant risks, such as healthcare and finance – whereas there are other scenarios in which it would be appropriate for chatbots to take a more prominent role and where their capabilities could more closely mimic human behavior.
For instance, you may create a feature on your grocery store app that allows customers to ask things like, “What should I cook tonight?” prompting the tool to list recipes based on what you sell in your store. Or you may build in clickable prompts that ask the user some general food preferences before the chatbot responds with some suggestions.
Factors to Consider with AI-Replicated Conversation
Whether your AI-powered chatbot is supplying you with information about a detailed product query, helping you gather information for a research project, or providing a variety of dinner suggestions, the relevance of its answer will ultimately depend on the data source it pulls from.
When thinking more broadly about generative AI content and its use in real-time communication with humans, we should consider various factors: What data was used to train the model? Are there any biases in the model as a result of this data? Has the model been programmed to have a specific perspective or agenda? Is the information being provided by the chatbot in the conversation verifiable?
AI-generated chatbots reflect those who create them along with the positive and negative aspects of the human conversation history it’s trained on. Given the growing power of AI to not only answer questions but also to persuade and influence the behavior of the people with whom it interacts, we need to critically evaluate the training data and the output of these machines.
Wrapping Up
AI is making great strides to replicate human conversation in various use cases to drive greater efficiency and productivity. It can successfully act as a virtual sales assistant for grocers, offering recipe advice and product discovery. However, because generative AI chatbots reflect their training data, we must remain mindful that it inevitably has limitations and may introduce bias. Therefore, its use in high-risk scenarios, such as healthcare, must be carefully regulated. That being said, as long as we continue to review and regulate the potential for generative AI to enhance, we will increasingly welcome it to replace human-managed conversations successfully.