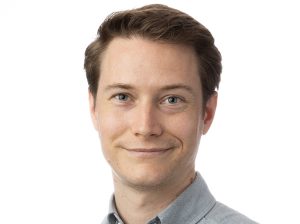
As we step into 2024, one trend stands out prominently on the horizon: the rise of retrieval-augmented generation (RAG) models in the realm of large language models (LLMs). In the wake of challenges posed by hallucinations and training limitations, RAG-based LLMs are emerging as a promising solution that could reshape how enterprises handle data.
The surge in popularity of LLMs in 2023 brought with it a wave of transformative possibilities, but it wasn’t without its hurdles. “Hallucinations” – instances where the model generates inaccurate or fictional information – and constraints during the training phase raised concerns, particularly in enterprise data applications.
However, the advent of RAG models promises to mitigate these challenges, offering a robust solution that could revolutionize data accessibility within organizations.
RAG models offer a solution to combat the challenges of hallucinations by providing auditable and up-to-date information. These models enable access to external data stores, ensuring the information provided is not only reliable but also current.
For businesses relying on data-driven insights, embracing RAG-based LLMs could be a game-changer. These models enhance the reliability and relevance of the information derived, providing auditable, up-to-date data that is crucial for informed decision-making.
The crux of RAG models lies in housing subject-matter expertise outside the model, often in vector databases, knowledge graphs, or structured data tables. This setup creates a sophisticated and low-latency intermediate layer between data stores and end-users. However, it also amplifies the consequences of inaccurate data, necessitating a robust data observability framework.
As enterprises increasingly shift towards deploying RAG models in production use cases, the need for data observability also becomes paramount. Organizations will need to more heavily invest in comprehensive data auditing processes to ensure the reliability of information being referenced by RAG-based LLMs.
One of the industry leaders placing a significant bet on RAG models is Databricks. In a recent fireside chat at Money 2020, Ali Ghodsi, co-founder and CEO of Databricks, revealed their customers are actively embracing RAGs, with 60% of their use cases involving LLMs being built upon this architecture. The company sees this new technology as a cornerstone for future advancements in data observability within LLMs.
In 2024 and beyond, RAG-based LLMs will become a driving force in the evolution of data processing and analysis. It’s imperative for businesses to not only embrace this technology but also fortify their data observability practices to harness the true potential of RAG-based LLMs in the ever-expanding landscape of artificial intelligence.