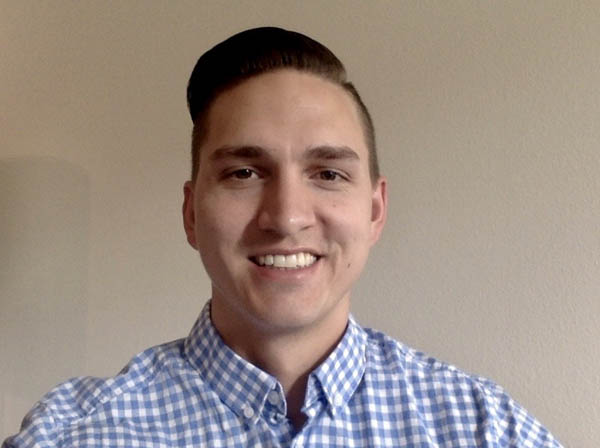
Click to learn more about author Scott Reed.
The true worth of AI is not the allure of advanced and innovative methodologies, but the potential for value to be ultimately added to your business. During development, when you train your model and see strong performance across cross-validation folds, the holdout, and an external prediction dataset, it may be tempting to roll out the red carpet and parade the results. Sure, the potential business value is seen within the model, but how do we protect and nurture it over time? Ongoing model performance can be unpredictable and volatile, with the possibility of changes in the input data or business process. Without an overarching infrastructure to safeguard that value, AI cannot achieve its desired impact.
Creating and sustaining an infrastructure for production models ensures that the value your model provides can persist so long as the enterprise remains diligent. With this system in place, not only can we benefit from the value our model provides, but we can ensure transparency, stability, and guardrails.
There are three pillars of trusted AI: performance, operations, and ethics. Performance includes Data Quality, model accuracy, and speed. In this article, we will look at our second pillar of trust, operations.
Operations relates to the question: “How reliable is the system that my model is deployed on?” This pillar focuses on creating a system with robust governance and monitoring, which incorporates humility into the decision process and provides sufficient transparency to stand up to regulatory requirements. There are five components to operations: governance and monitoring, humility, compliance, security, and business rules. In this article, we will focus on three in particular: governance and monitoring, humility, and compliance.
Governance and Monitoring
Within the context of Data Science and machine learning, proper governance and system monitoring is supported through both tools and processes that ensure stability, establish user-based permissions based on roles and responsibilities, and create approval workflows. We will separate governance and monitoring to show how they contribute to the overall operations architecture.
Let’s start with monitoring. Many things must be monitored in relation to a production model, including but not limited to accuracy tracking, system performance statistics, and the common issue of data drift. Data drift occurs when the scoring data differs (statistically significant difference) from the data used to train the model. The origin is usually a Data Quality issue or a change in feature composition. In the case of data drift, one of many potential indicators through monitoring, our next step would be a model retrain. How do we evaluate this production model change and ensure it doesn’t disrupt the entire system and any downstream components?
Now that we’ve detected production model issues through monitoring, what does the governance workflow look like? The process of training, testing, comparing prospective models, analyzing downstream impacts, and model versioning must be packaged and repeatable. This series of checks and approvals should be managed through an approval workflow and secured with user-based permissions. No signoffs or verifications should be skipped; permissions should be granted only to those who need it along the way. But if governance is about the big picture, how do we support our model operations in real time, at the level of individual predictions?
Humility
One key aspect of designing a system around AI is recognizing that any model’s predictions are probabilistic. For example, in binary classification, our model makes predictions in the form of raw scores between 0 and 1. Based on an optimized threshold, the model predicts either class 0 or class 1. However, there are situations in which the model is not confident in a prediction – for example, when very near to that optimized threshold, in a “low confidence” region. There are other scenarios too when analyzing the scoring data or prediction we may have reason to doubt the veracity of the model prediction. So how do we translate this into real-time protection to ensure our model makes safe and accurate decisions at the level of an individual prediction?
Using a set of triggers, such as identifying outliers or an unseen categorical value, the system can take certain predefined actions to guard against uncertain predictions. Consider a model that predicts whether or not an image is a dog or a wolf. Perhaps the training data was authored by a photographer using professional equipment. A new scoring image is taken by a different photographer with much lower-quality equipment, resulting in a blurry, small image. This leads our model to have a prediction close to the threshold; the system identifies this trigger, and instead of using the predicted value, can default instead to a “safe” value – for example, “dog” – and mark the record for review.
Compliance
An enterprise should be able to generate robust documentation for the current production model. There are key stakeholders that have different responsibilities, backgrounds, and concerns, who must fully understand the model and its surrounding infrastructure. The legal team may need to know where you sourced the data. The analytics center of excellence may need to approve the algorithm you chose and the hyper-parameter selections. The risk department may want to understand how the current version of the model differs from past versions. Finally, your business sponsor may want to understand how error rates translate to dollars and cents. Each of these different personas needs continuous access to up-to-date documentation on your production models from their inception to today.
Now that we know how we can trust our model’s performance and the operations around production models, we will focus next time on trust in ethics, looking at whether your model has unintended consequences and upholds the values of your organization.