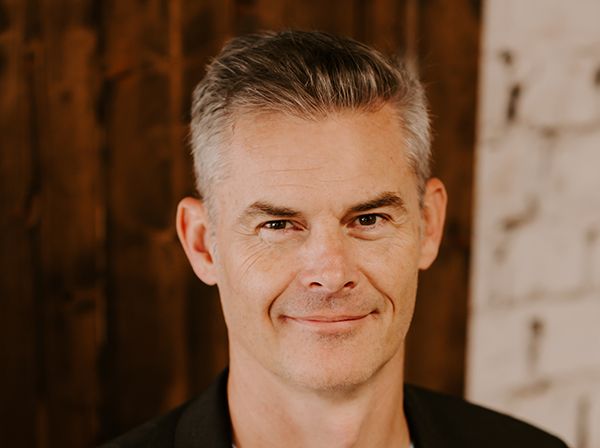
While the health care industry has lagged a bit behind other industries in adopting artificial intelligence (AI) and machine learning (ML) – and rightfully so, considering very valid security and safety concerns – its leaders have undergone a mindset shift, recognizing the value of technological innovations and data analytics.
Since its implementation, data analytics has completely transformed health care, influencing how organizations do their jobs and provide care – and changing how researchers, policy advocates, and patients operate within the system. This data has improved health care delivery in myriad ways, informing the execution of medical studies, improving patient understanding of health insurance and medical test costs, and guiding physicians in their preventative recommendations.
Health care leaders have found another valuable application for this data:
- Understanding patient pain points along their health care journey
- Identifying training needs for call center agents
- Uncovering insights from customer experiences (CX) and marketing initiatives
To avoid a looming data deluge in a world expected to generate and store over 200 zettabytes in the cloud by 2025, health care organizations need a reliable, efficient strategy for collecting, evaluating, and analyzing data. This strategy should help leadership gather and use insights for informed decision-making.
Enter AI and ML tools, which will continue to see their use in analytics increase. Health care organizational leadership should leverage this technology to extract valuable, actionable data insights for delivering a better CX. Here’s why.
1. Listening at scale helps solve common issues.
AI and ML empower organizations to listen and understand customer voices more effectively, pinpoint stumbling blocks and resolve common challenges or barriers – like the Eddy Effect – interfering with CX and customer interactions.
Machine learning relies on training and learning datasets – and inaccurate inputs generate inaccurate results and predictions. The most effective ML models are accurate 70% to 90% of the time. And that accuracy relies on relevant, representative, unbiased, comprehensive training data generated from unstructured data.
The health care industry captures unstructured data from health-care-focused conversations like a customer calling with questions about a procedure or an insurance bill. While it’s impossible for people to analyze each patient conversation (often happening in the thousands) every day, AI and ML tools can analyze every single conversation. With the right technology, health care organizations can collect and analyze conversational data at scale.
2. Conversational intelligence offers deeper insights than survey-based data.
Surveys like net promotor scores (NPS) and customer satisfaction (CSAT) have been the gold standard for decades. But they’re unable to get more granular and examine root causes of customer concerns – nor were they ever intended to serve that function.
Conversely, conversational intelligence offers a holistic look at CX because of its ability to leverage AI and ML to capture unsolicited, bidirectional customer data. This tool captures 100% of unstructured data generated from customer conversations to yield deeper insights across the whole continuum of CX.
3. Leveraging AI adds value to data analytics.
AI-driven tools extract valuable, actionable data insights that health care leaders can use to improve CX. AI and ML empower health care organizations to listen more effectively to their customers’ voices and understand the barriers and issues causing frustration.
However, many industries have relied on models trained using non-industry-specific data, leading to inaccurate interpretations because the nuances of the intent or meaning behind words are lost. ML reliability depends on how models are trained. AI trained by health-care-specific conversations is capable of:
- Extracting the most significant value from health care conversations
- Deeply and thoroughly understanding the health care industry’s regulatory nature
- Building ML models to bring speed and value to health care organizations
4. Integrating qualitative and quantitative insights provides opportunity.
Data immersion empowers organizations to tell a powerful story. Data-backed storytelling combines qualitative and quantitative data to enrich concepts, provide meaning, and help people connect.
Quantitative data provides concrete information in number form. Qualitative data enhances quantitative data by inviting the exploration of ideas and helping organizations identify and respond to issues. When used together, these analyses create a more comprehensive, holistic picture by presenting the “what” and the “why” in one place.
In one use case, a life sciences company wanted to standardize call center messaging and improve its CX. The company adopted and used AI technology to analyze all conversational data from when customers began their journey through their final communication. The analysis focused explicitly on customer experiences with high call volumes, helping the company identify where:
- A lack of consistency in agent messaging resulted in customer stress or confusion.
- Customers became confused or lost (the Eddy Effect) within their experience.
- The organization had opportunities to offer additional support to customers.
Storytellers (those analyzing the data) used qualitative and quantitative analyses to evaluate the collected data and identify specific customer challenges. These disparate data types complemented each other and enabled the organization to tell a more contextual, data-backed story of the customer journey.
Humans Will Always Play an Important Role in Analytics
It’s a fallacy to think AI will take over the world of analytics, completely replacing the human element. What it can do, however, is manage substantial data volumes more effectively and efficiently than people – and free up humans to handle other challenges requiring critical thinking.
Health care organizations once lacked the technology to effectively manage the almost limitless quantities of complex, unstructured data produced every day. But the evolution of conversational intelligence has made it possible to bring data to life, tell compelling stories, uncover deeper insights, and guide strategic decision-making by listening at scale.