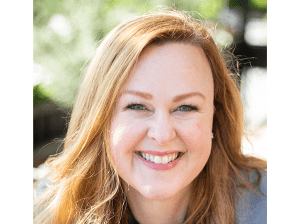
In the past year or so, generative AI has received more media attention than any other type of technology. Up until this point, data science has been at the center of these innovation stories, and rightfully so: None of the next-generation technology tools can work without a strong data science program.
As soon as generative AI tools like ChatGPT and DALL-E hit the mainstream, opinions from experts and the public ran the gamut from AI skeptics to those who believe AI will automate the vast majority of work in the future.
As technologists, we know: The truth really falls somewhere in the middle of these extremes.
But there are likely others on your team, in your company, on your board who might not know the finer points of what’s hype and what’s reality. Further, the existence of these myths could become a barrier for building advocacy for well-designed, thoughtful generative AI solutions within your company.
I believe digital experts should make new technologies in the workplace tangible for leaders and colleagues. Here are three generative AI myths you might encounter in the year ahead, and how to bust those myths:
Myth 1: Generative AI can automate everything – and may replace our jobs.
When generative AI burst into mainstream awareness, the question on everyone’s lips was: “Is AI coming for my job?”
I’m all for a little bit of drama, but this exaggerated view of AI is a myth. AI is not coming for your job, it’s coming to your job. As data scientists and technology experts, we’ve been using tools like predictive models, decision engines, and fraud detection for years with fantastic results. Generative AI is the final piece of the puzzle that will pair up with these other intelligent tools and help businesses achieve real digital transformation.
But lurking below the surface, there’s another myth to bust about the practical application of AI in the workplace.
Given AI’s powerful capabilities, everyone wants to set AI tools to the task of solving super-complex problems. But why start with the hard problems when AI is more capable of tackling the easy ones?
Generative AI has proven to be capable of taking on the administrative burden of humans in the workplace, but it can’t make judgement calls for the business – and it likely never will. Generative AI won’t automate everything because humans need to be there to make informed, solid decisions.
A true win for generative AI in the workplace is setting the models to the task of handling all the low-hanging fruit on employees’ to-do lists, leaving employees to focus on the tasks that require judgment calls, decision-making, and a sensitive, human touch.
In this way, the business process can change, and companies can achieve new levels of efficiency and growth.
Myth 2: Your data isn’t safe within a large language model.
A concern I’ve heard frequently in the past year from colleagues and clients alike is about the safety of data and documents when used in a large language model (LLM).
With data privacy at top of mind for many and cybersecurity threats at an all-time high, it’s understandable to fear that your data and documents will become part of the public domain when used in an LLM.
Hypothetically, that can be true: In the case of IP leaks or data breaches, company data can become part of the public domain.
But, with proper cybersecurity infrastructure, as well as a strong policy and practice governance, companies can secure and safeguard against the public sharing of data and documents within an LLM. Data must remain isolated and encrypted within your own network, and companies must ensure a secure transfer of data between storage and LLMs as these tools are leveraged. Many available LLMs today – for example, OpenAI’s ChatGPT – can be installed within a company’s ecosystem to ensure that tools can be used, but the data and learnings from generative AI do not become a part of future training or machine learning within that LLM. The saying goes: “Data can check in, but it can never leave.” These safeguards can be implemented with the proper security controls and assertions from your LLM vendor.
Fortunately, regulatory guidelines are catching up to the AI sector. We will soon see more legislation, such as those now underway in the EU, regarding the use of generative AI tools (and possibly AI tools in general), with the purpose of protecting users of these tools. Companies that do not comply or whose cybersecurity infrastructure is insufficient will likely face significant consequences.
Myth 3: Generative AI can be applied to any kind of problem.
In August, The Wall Street Journal published an article entitled “AI Botched Their Headshots.” The story recounted the effect that women of color experienced when generative AI applied its concept of “professional.” Upon requesting that generative AI edit their own photos to produce a professional headshot, the women who were interviewed found that the results went awry. The generative AI tool showcased the concept of technology-driven bias in LLMs when the technology changed skin tone and facial features in its interpretation of what should be considered a more professional image.
What ultimately went wrong in this example? Generative AI was asked to make an interpretation based on what it learned from human content in the digital space. We know that, unfortunately, biases exist against professional women in all kinds of spaces so it’s no surprise the AI model, which had been trained on what it learned from content created by humans, interpreted that bias as well.
It’s clear that whether queries are as simple as generating an image or complex as solving a business challenge across a global company, generative AI cannot be tasked to solve every problem.
Don’t ask generative AI to make an interpretation. Instead, when examining what kinds of problems should be a candidate for generative AI, keep it to just the facts.
When leaders leverage generative AI tools from a place where the AI does not have an opportunity to interpret and apply bias, the tools will function much more effectively for workplace and administrative applications.
The future is bright for generative AI tools in the workplace. Through iterative prompt engineering, technology teams will come together with their business partners to design solutions that work and are accepted by operational teams. As leaders “buy in” to proposed AI solutions and as trust and acceptance of generative AI grows, these tools will spread rapidly.
Technology teams and data scientists share responsibility for making technology tangible for leaders and highlighting touchpoints for how digitization fits into their workflow. It all starts with busting myths like these and walking leaders, one step at a time, toward a more efficient future.