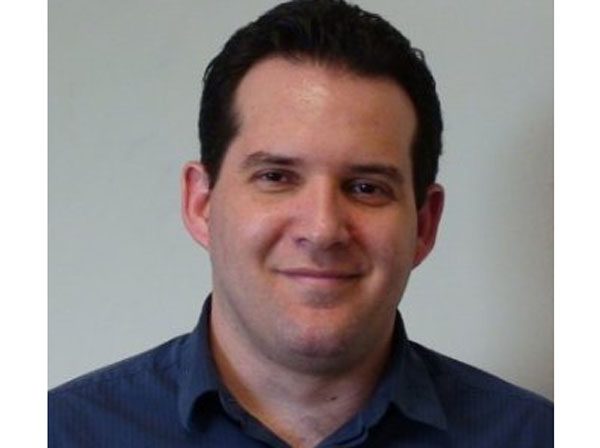
Click to learn more about author David Talby.
A shortage of technical talent has long been a challenge for getting AI projects off the ground. While research shows that this may still be the case, it’s not the end-all-be-all and certainly not the only reason so many AI initiatives are doomed from the start.
Deloitte’s recent State of AI in the Enterprise survey found the type of talent most in-demand — AI developers and engineers, AI researchers, and data scientists — was fairly consistent across all levels of AI proficiency. However, business leaders, domain experts, and project managers fell lower on the list. While there’s no disputing that technical talent is valuable and necessary, the lack of attention on the latter titles should be a bigger part of the conversation.
It’s likely that the technical skills gap will persist for the next few years, as university programs play catch up to real-world applications of AI, and organizations implement internal training or opt for outsourcing entirely. That doesn’t mean businesses can wait for these problems to solve themselves or for the talent pool to grow. In order to avoid being one of the 85 percent of AI projects that fail to deliver on their intended promises, there are three areas organizations can focus on to give their projects a fighting chance.
1. Organizational Buy-In: AI-Driven Product, Revenue, and Customer Success
Understanding how AI will work within a professional and product environment and how it translates to a better customer experience and new revenue opportunities is critical — and that spans far beyond the IT team. Being able to train and deploy accurate AI models doesn’t address the question of how to most effectively use them to help your customers. Doing this requires educating all organizational disciplines — sales, marketing, product, design, legal, customer success — on why this is useful and how it will impact their job function.
When done well, new capabilities unlocked by AI enable product teams to completely rethink the user experience. It’s the difference between adding Netflix or Spotify recommendations as a side feature versus designing the user interface around content discovery. More aspirationally, it’s the difference between adding a lane departure alert to your new car versus building a self-driving vehicle that doesn’t have pedals or wheels. Cross-functional collaboration and buy-in on AI projects is a vital part of the success and scaling and should be a priority from the get-go.
2. Realistic Expectations: The Lab vs. the Real World
We’re at an exciting juncture for AI development, and it’s easy to get caught up in the “new shiny object” mentality. While eagerness to implement new AI-enabled efficiencies is a good thing, jumping in before setting expectations is a sure-fire way to end up disappointed. A real instance of the challenges organizations face when implementing and scaling AI projects comes from a recent Google Research paper about a new deep learning model used to detect diabetic retinopathy from images of patients’ eyes. Diabetic retinopathy, when untreated, causes blindness, but if detected early, it can often be prevented. As a response, scientists trained a deep learning model to identify early stages of the disease symptom to accelerate detection and prevention.
Google had access to advanced machines for model training and data from environments that followed proper protocols for testing. So, while the technology itself was as accurate, if not more so than human specialists, this didn’t matter when applied to clinics in rural Thailand. There, the quality of the machines, lighting in the rooms in the clinic, and patients’ willingness to participate for a host of reasons were quite different than the conditions the model was trained on. The lack of appropriate infrastructure and understanding of practical limitations is a prime example of the discord between Data Science success and business success.
3. The Right Foundation: Tools and Processes to Operate Safely
Successful AI products and services require applied skills in three layers. First, data scientists must be available, productively tooled, and have domain expertise and access to relevant data. While AI technology is becoming well understood, from bias prevention, explainability, concept drift, and similar issues, many teams are still struggling with this first layer of technical issues. Second, organizations must learn how to deploy and operate AI models in production. This requires DevOps, SecOps, and newly emerging “AI Ops” tools and processes to be put in place, so models continue working accurately in production over time. Third, product managers and business leaders must be involved from the start in order to redesign new technical capabilities and how they will be applied to make customers and end-users successful.
There’s been tremendous progress in education and tooling over the past five years, but it’s still early days for operating AI models in production. Unfortunately, design and product management are far behind, and becoming one of the most common barriers to AI success. This is why it might be time for respondents of the aforementioned Deloitte survey to start putting overall business success and organizational buy-in before finding the top technical talent to lead the way. The antidote for this is investing in hands-on education and training, and fortunately, from the classroom to technical training courses, these are becoming more widely available.
Although a relatively new technology, AI has the power to change how we work and live for the better. That said, like any technology, AI success hinges on proper training, education, buy-in, and well-understood expectations and business value. Aligning all of these factors takes time, so be patient, and be sure to have a strategy in place to ensure your AI efforts deliver.