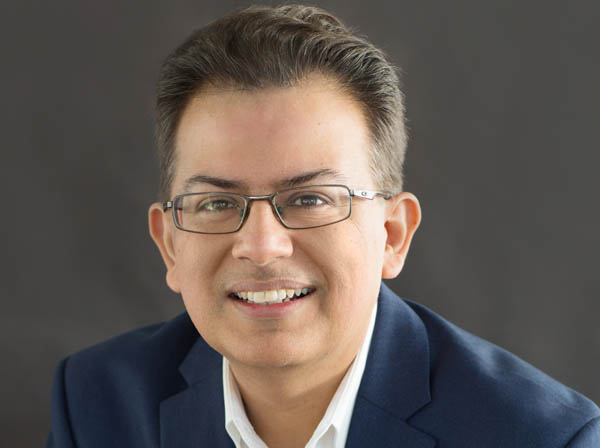
There’s been a lot of talk about the modern data stack recently. Much of this focus is placed on the innovations around the movement, transformation, and governance of data as it relates to the shift from on-premise to cloud data warehouse-centric architectures. When it comes to the analytics layer – which sits on top of the modern data stack – the conversation ends with the traditional ways that data is consumed, whether it be through dashboards, SQL query, or the building of Data Science models.
However, data-driven organizations should not be content with these same-old approaches to data consumption, as there are more modern and evolved ways for organizations to consume and analyze data today. It’s similar to the evolution of the internet, which didn’t just improve the quality of phone calls, but also revolutionized social interactions and put every song and movie in everyone’s hands. The same reimagination of user experiences should come for consumers of data and analytics.
Now let’s break down how we get there.
The Modern Data Stack
Adoption of the modern data stack has been driven not only by the shift to the cloud and the limitless availability of storage and computer power, but also by more practical ways to deploy cloud technology: on-demand/do-it-yourself (DIY) availability, consumption-based pricing models, and non-vendor-lock-in.
The layers of the modern data stack, and examples of each layer, include:
- Data sources: applications, databases, APIs, and files
- Ingestion and data pipelines: Fivetran, Stitchdata, Segment
- Storage: data warehouses such as Snowflake, Amazon Redshift, and Google BigQuery; data lakes, such as Databricks and Amazon S3
- Data transformation: dbt, Matillion
- Analytics: business intelligence platforms such as Looker, Data Science platforms such as DataRobot, as well as the new breed of modern decision intelligence tools
In the analytics layer, the workflow tends to consist of the following: business users consuming dashboards, analysts querying data using SQL, and data scientists building machine learning models. These are fairly old approaches, and reinforce two processes that are decades-old; first, business teams waiting on data experts to generate reports as these dashboards, and second, the inability for business users to get the answers they need to deeper questions – such as “why” sales may be down in a specific region. The result: slower, less-informed decision-making, which has a direct impact on the business’ bottom line.
With a new breed of analytics tools powered by AI and machine learning, organizations can generate smarter insights that are driven by automated data analysis and leverage the innovation of the modern data stack.
Modernizing Via Ad Hoc Data Exploration and Automated Insights
Every consumer expects to get answers through a free-form search interface, initiate transactions by tapping on a screen, and have relevant information pushed to them without asking. Data analysis should be no different from these everyday technology experiences. Business users are more data-savvy than ever and want to be able to explore, drill, and analyze more data on their own – and with fewer guardrails.
If the promise of a cloud data warehouse is to store all the unaggregated data, data-driven organizations should push the boundaries of making the data more easily accessible. And, with so much data available in the cloud, automation is the fastest, simplest way to allow technical and non-technical users to get answers from their data. While manual querying of data will always be an essential tool for analyst teams, augmenting the discovery of insights with the application of automated statistical analysis and machine learning algorithms enables analytics teams to get deeper, more granular insights without having to inspect every combination and cross-section of data manually. This augmentation represents the future of how analysts will get answers easier, iterate on insights discovery much faster, and even involve business users in the process.
Making AI and Machine Learning Accessible to Business Analysts
The modern data stack should also enable the upskilling of the spectrum of data analyst personas, allowing all to participate in building machine learning models for analysis and predictions. As governed data becomes more widely available, machine learning techniques should become more accessible to those who are not expert data scientists. When coupled with automated feature engineering and the explainability of visually oriented outputs, automated machine learning modeling will provide greater flexibility to take advantage of out-of-the-box models in advanced analytics systems, plus the power to inject custom-coded models into the analytics workflow.
In closing, the modern data stack should be not only a technical exercise in rebuilding the suite of tools for Data Management but should make way for a new analytics layer. By offering a modern experience to every analytics persona, automating analysis against more data, and making AI and machine learning accessible to citizen data scientists, organizations can enhance their modern data stacks and accelerate the journey of understanding what is happening in the business, why metrics have changed, and uncovering the specific granular actions to impact business outcomes.