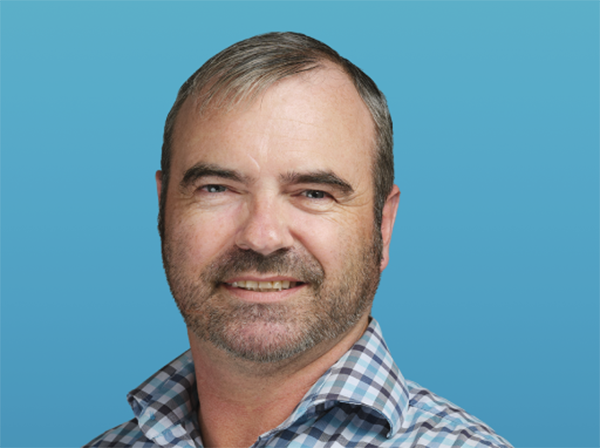
In today’s insight-informed world, businesses of all sizes need to be able to access and analyze their data in order to make informed decisions. However, data is often siloed in different systems and difficult to access, making it challenging for businesses to get the insights they need. The demand for help to streamline these operations is becoming increasingly critical. With fixed budgets and time limitations, it can be difficult for enterprises to innovate and keep up with the competition. This is where artificial intelligence (AI) comes in. AI can help businesses democratize their data by making it more accessible and easier to analyze. Let’s dive into the specific ways AI is helping transform the data analysis landscape.
Current Data Analysis Challenges Plaguing Enterprise Efficiency
Leaders in today’s current business environment encounter numerous challenges that have an impact on enterprise efficiency. Organizations, spanning various industries like visitor attractions, have identified issues such as:
Siloed data sets confined within source systems: This is a prominent issue where many organizations initially perceive a scarcity of data, only to discover a wealth of untapped information upon closer examination. The potential lies in uncovering correlations between disparate data sets, whether it’s the distinction between on-site and online activities or the profound insights concealed within seemingly trivial details like zip codes. Additionally, the opportunity to incorporate external data sources, such as weather data, can provide valuable context. However, the challenge here involves ensuring that this “data liberation” doesn’t inadvertently introduce new barriers, such as data warehouses requiring specialized teams for access.
It is crucial for organizations to achieve data democratization, placing data and analytical power directly in the hands of those who need it. This is where AI comes into play: automating data integration and analysis, enabling organizations to break down data silos, identify correlations and unlock valuable insights without the need for extensive manual data handling or specialized teams.
Nurturing data and building data literacy: One of the most significant hurdles commonly cited by industry leaders is the cultivation of a data-centric environment and the development of data literacy across the organization. Data can be intimidating and even confrontational, making it difficult to break the habit of making decisions without data support. To overcome this obstacle, enterprises can employ advanced strategies, including leveraging technologies such as AI to expedite and automate processes such as data consolidation, connecting different data sources and creating a unified view of all the company’s data. AI can also be used to provide user-friendly analytics tools that allow employees at all levels to explore and analyze data without having to engage in technical processes, including writing code. Additionally, AI can be used to offer personalized insights by tailoring data visualizations and reports to the specific needs of individual users.
Limited time and budget: In an attempt to work within constrained time and budget, organizations often risk entangling themselves in extensive data infrastructure projects, leading to prolonged timelines, higher costs, and resource diversion from critical operations. Instead, companies can adopt a balanced approach, combining commonly available solutions for efficiency and reserving custom development for unique needs. Adopting solutions with AI enhancements can streamline key areas for businesses including forecasting, and the voice of the customer at scale, where analytics objectives that feel like big leaps can instead become quick wins, especially with a productized solution. For example, AI-enhanced forecasting can help businesses reduce costs by automating the forecasting process and improving the accuracy of forecasts. AI-enhanced voice of the customer (VoC) can help businesses save time by automating the process of collecting and analyzing customer feedback.
AI’s Evolving Role in Data Analysis
Data analytics leaders have long integrated AI into data analytics software, with a particular focus on two key AI forms: machine learning (ML) and natural language processing. ML is primarily used for forecasting, such as predicting visitation patterns at site attractions, while natural language processing is employed for insights, such as interpreting visitor attraction reviews.
In recent years, businesses have witnessed significant advancements in both fields, with innovations exemplified by ChatGPT. However, in the realm of data products, user confidence hinges on factors like trust, transparency, and control, which AI-driven bots may not always fully address. Nonetheless, AI continues to make substantial contributions to various, unique aspects of business performance and growth.
AI’s Role in Enhancing Analysis and Forecasting
While there’s been a rush to generative AI applications in analytics, the risk of error in how this technology is applied in this domain is also high. However, for those creating data products, it has already proven useful for addressing specific challenges where accuracy is less a concern, including the generation of mock data – refining how businesses deliver data products. Businesses can leverage AI to generate data such as fake reviews and use that information to test and demo a product. This type of demo data generation helps to create a valuable and practical data product that is quick and efficient.
One of the key benefits of using AI to generate mock data is that it allows businesses to test and demo data products without having to collect real data from users. This can be a valuable tool for businesses that are developing new data products or that are testing new features for existing data products.
For example, my colleagues and I leveraged AI to create fake reviews for a pretend theme park in Antarctica and asked the algorithm for a touch of humor. The results included: “The icicles forming on the rollercoaster were a worry but the wind chill factor on my cheeks was real. 1 star.” Fake transactions from the theme park’s retail store were also generated, including samples that curated: “Make Antarctica Great Again Cap, $25.00.”
When considering the use of data for business forecasting, an analogy my colleagues and I frequently use is “Guess, Grind, or AI.” In this case, business leaders can opt to make educated guesses, trust their instincts, or engage in extensive spreadsheet analysis to address difficult scenarios. These scenarios may involve factors such as historical school holiday data, weather changes, unforeseen events, growth rates, and the impact of events like the pandemic. Or they can use ML.
ML’s Role in Enhancing Analysis and Forecasting
In forecasting, ML delivers highly automated, finely granular, and more accurate predictions than manual projections. It solves the knowledge risk inherent in organizations where projections are based on “gut feel” and “years of experience.” ML can also pick up on the nuances and subtleties of multiple features playing out in parallel that are invisible to the human eye.
For example, a zoo can use ML to forecast visitation by considering current and expected impacting events such as the day of the week, time of year, weather, and local events. ML can also be used to analyze the influence of each factor and determine the residuals between forecast and actual visitation for attribution.
Enhancing, Not Replacing, with AI
AI is a powerful technology that can enhance and optimize data analysis, but it doesn’t replace the essential role of software engineers and human expertise. Great technology demands leadership, creativity, empathy, and the ability to navigate complex ecosystems and stakeholders – a uniquely human capacity.
However, AI can assist businesses, offering several benefits within data analysis. By leveraging AI-enhanced analytics technologies, organizations can make accurate, informed decisions more quickly and efficiently. ML can also greatly improve forecasting accuracy, providing deeper insights into complex scenarios and mitigating knowledge risks.
For organizations reluctant to invest in data analytics and AI, the challenge often stems from a cultural barrier, which can hinder competitiveness. Without embracing these technologies, accountability weakens, leading to uninformed decisions and revenue losses. Manual processes consume excessive time, restricting the creative freedom to explore insights and take meaningful actions. It is time for organizations to recognize all that AI has to offer, as it complements human capabilities, accelerating growth and efficiency when combined with human ingenuity.