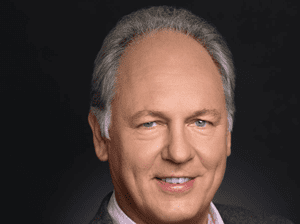
2023 will bring exciting advances in AI and graph technology. One of the most compelling innovations will be the ability for quantum programs to be turned into graphs and vice versa. Natural language understanding will become part of AI models. The adoption of standards-based semantic layers will spike as they enable data selection through business terms. Graph neural networks (GNNs) will become standard in knowledge graphs and causal knowledge graphs will emerge.
Physics-Informed AI
We are entering into an era of physics-informed AI. Based on the realization that quantum computing programs are graphs and therefore, it should be possible to use graphs to create quantum programs. In the next couple of years, we can expect to see quantum compilers that are written in Lisp expressed in a graph. In addition, graphs that can be turned into quantum graph learning solvers will emerge and be used to generate useful solutions that cannot be produced efficiently by classical systems.
Natural Language Understanding
In 2023 we will start to see natural language understanding become possible for AI applications. There will be a transition from simple pattern matching to language understanding within the underlying model. By starting with taxonomies, ontologies, speech technology, and new rule-based approaches – it will be possible to take natural language understanding and instantly turn it into triples that describe the pragmatics of the world. These triples become the underlying ontological description of the world, which is essential to produce high-quality AI using natural language.
Standards-Based Semantic Layers
Data fabrics, data lakes, and data lakehouses contain a surplus of unstructured and semi-structured data from external sources. In 2023 there will be a significant uptick in organizations applying W3C standards-based semantic layers atop these architectures, where data assets are described by metadata in familiar business terms and enable business users to select data through a lens of business understanding. This method will provide a seamless business understanding of data that fosters a culture of data literacy and self-service, while simplifying data integration and improving analytics.
Causal Knowledge Graphs
The next few years will see growth in causal AI starting with the creation of knowledge graphs that discover causal relationships between events. Health care, pharma, financial services, manufacturing, and supply chain organizations will link domain-specific knowledge graphs with causal graphs and conduct simulations to go beyond correlation-based machine learning that relies on historical data. Causal predictions have the potential to improve the explainability of AI by making cause-and-effect relationships transparent.
Graph Neural Networks
Graph neural networks (GNNs) excel at predicting events, explaining them and classifying entities at scale to deliver striking accuracy. In the coming year and beyond, companies will use GNNs as an AI approach for knowledge graph enrichment via text processing for news classification, question and answer, search result organization, and much more.