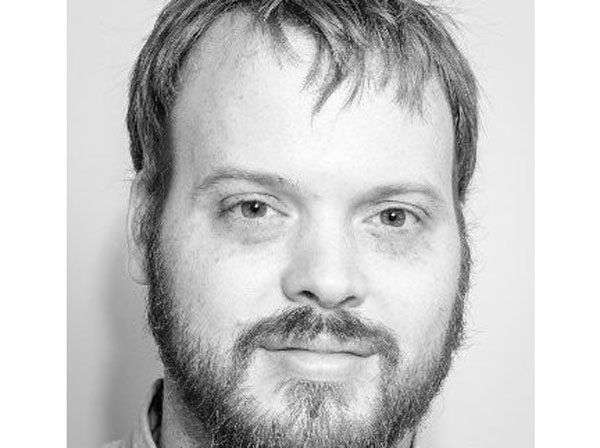
Click to learn more about author Trevor Anderson.
2020 will forever be known as the year the coronavirus pandemic swept the globe. Coping with the crisis pushed organizations across industries (as well as consumers) into drastic shifts. In some cases, trends that were expected to play out over a decade accelerated to just a couple of quarters. With consumers forced to stay home, the penetration of online commerce doubled from 15 percent of retail to over 30 percent in just three months, with digital banking and payments seeing similar growth. For instance, PNC Bank’s sales jumped from 25 percent digital to nearly 75 percent digital during COVID-19, while Visa reported a staggering 150 percent increase in contactless payments for the 12 months ending March 2020.
Online commerce companies struggled to varying degrees to incorporate the sudden behavioral changes brought on by the pandemic into their fraud and risk mitigation strategies, some more than others. By now, most have settled into the “new normal.” Heading into 2021, looking back at how companies dealt with the crisis can provide some useful lessons for dealing with yet another series of changes that are likely to come as we slowly transition into a post-COVID world.
COVID-19 Introduced New Fraud Patterns
The COVID-19 outbreak brought more people into the digital realm for the first time. According to Visa, more than 13 million cardholders in Latin America alone made their first-ever online transaction in the March quarter of this year. With a record number of individuals shopping and banking online for the first time, new account openings also skyrocketed. But with the influx of new customers opening online accounts, companies found that the lack of transaction histories made it much more difficult to verify the identity of individuals behind the account and detect potential fraud.
The pandemic also had a massive impact on the financial and housing circumstances of many people. In an effort to help customers impacted by the pandemic, phone companies across the world offered new prepaid packages with increased data and mobile hotspots. While this gave consumers more options for affordable phone service, it also introduced new phone data inputs online that can appear suspicious to fraud detection platforms. Because prepaid phones are also popular with fraudsters (since they’re not as easy to tie to an identity), a spike in prepaid phone numbers can be a red flag.
Similarly, many individuals who lost their jobs or homes started living with friends and relatives, while others sublet rooms in their houses to help cover rent. In both cases, people were living in places where their name didn’t appear on a lease or a utility plan. New data from these sorts of circumstances made good customers look suspect, resulting in more false positives for fraud.
The Impact of COVID-19 on Fraud Risk Machine Learning Models
Supervised machine learning (ML) involves training an algorithm to map an input to the correct output, based on examples of input-output pairs. A core assumption is that the examples you train with are good representations of the cases you will see in the future. The huge impact of COVID-19 means this assumption is no longer true. Past data is no longer representative of the future.
In response to this shift, companies deployed three approaches:
- Underfit ML Models: If companies did not change their machine learning approach, then they ended up overfitting their models because past examples were no longer a good representation of the future. One way companies mitigated this was to intentionally under-fit models. For them, a modest but reliable model was preferable to one that looks great on paper but leads to surprises such as flooded manual review queues or more chargebacks and fraud.
- Rules-Based Models: Some companies reverted their ML systems back to rules-based systems. These systems require less historic data and are built to involve much more human intuition and supervision — an enticing option for teams attempting to respond to the sudden ups and downs of the pandemic. However, this approach also requires multiple steps for verification, which can impede the user experience.
- Manual Review: Other companies realized early on that they needed to do more manual review, but with COVID-19-related furloughs and hiring challenges, they couldn’t just hire more people. Companies that saw success were those that better leveraged the teams they already had by providing them with better training and tools. Relying on human judgment was the best they could do to respond to new fraud patterns. Yet, there’s a reason organizations use machine learning to stop fraud. It’s not just the difficulty of scaling a human workforce, but it’s also that machines are simply better and faster at detecting patterns. Human beings just can’t analyze enough data to get a firm grasp of the fraud patterns at play.
Companies that have done the human judgment piece well over the course of the pandemic are better prepared to start building better machine learning models for tomorrow. But how long will it take until companies have sufficient training data to start building machine learning models again?
Machine Learning Fraud Models in 2021
The amount of time it will take for machine learning models to “catch up” depends on how much training data a company needs. This dictates how far back they need to look to get enough data. Some of it also depends on how strong of a model they are looking to achieve. Some credit companies, for instance, want to model years’ worth of data, while others make do with less. Initially, in the chaotic period when COVID-19 first hit and people started suddenly shopping online much more, merchants were able to get by with much less training data and still have confidence that they understood the level of fraud risk.
There is still a lot of uncertainty in the year ahead, even as vaccines roll out and consumers return to physical stores and more typical shopping patterns. Some behaviors may never return to what they were before COVID-19. While uncertainty will continue in 2021, it won’t be as dramatic week by week or month by month. Companies that have started understanding behavioral patterns better in the last year will be better prepared to hit the ground running at the beginning of the new year and start building stronger machine learning models again. But to keep models flexible for any uncertainty that lies ahead, they will still need to continue using more recent data, assessing their progress each step of the way, and making changes to their models as circumstances change.