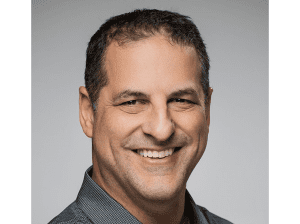
The new wave of data-hungry machine learning (ML) and generative AI (GenAI)-driven operations and security solutions has increased the urgency for companies to adopt new approaches to data storage. These solutions need access to vast amounts of data for model training and observability. However, to be successful, ML pipelines must use data platforms that offer long-term “hot” data storage – where all data is readily accessible for querying and training runs – at cold storage prices.
Unfortunately, many data platforms are too expensive for large-scale data retention. Companies that ingest terabytes of data daily are often forced to quickly move that data into cold storage – or discard it altogether – to reduce costs. This approach has never been ideal, but it’s a situation that’s made all the more problematic in the age of AI because that data can be used for valuable training runs.
This article highlights the urgency of a strategic overhaul of data storage infrastructure for use by large language models (LLMs) and ML. Storage solutions must be at least an order of magnitude less expensive than incumbents without sacrificing scalability or performance. They must also be built to use increasingly popular event-driven, cloud-based architectures.
ML and GenAI’s Demand for Data
The principle is straightforward: the more quality data that’s available, the more effective ML models and associated products become. Larger training datasets tend to correlate with improved generalization accuracy – the ability of a model to make accurate predictions on new, unseen data. More data can create sets for training, validation, and test sets. Generalization, in particular, is vital in security contexts where cyber threats mutate quickly, and an effective defense depends on recognizing these changes. The same pattern also applies to industries as diverse as digital advertising and oil and gas exploration.
However, the ability to handle data volume at scale isn’t the only requirement for storage solutions. The data must be readily and repeatedly accessible to support the experimental and iterative nature of model building and training. This ensures the models can be continually refined and updated as they learn from new data and feedback, leading to progressively better performance and reliability. In other words, ML and GenAI use cases require long-term “hot” data.
Why ML and GenAI Require Hot Data
Security information and event management (SIEM) and observability solutions typically segment data into hot and cold tiers to reduce what would otherwise be prohibitive expenses for customers. While cold storage is much more cost-effective than hot storage, it’s not readily available for querying. Hot storage is essential for data integral to daily operations that need frequent access with fast query response times, like customer databases, real-time analytics, and CDN performance logs. Conversely, cold storage acts as a cost-effective archive at the expense of performance. Accessing and querying cold data is slow. Transferring it back to the hot tier often takes hours or days, making it unsuitable for the experimental and iterative processes involved in building ML-enabled applications.
Data science teams work through phases, including exploratory analysis, feature engineering and training, and maintaining deployed models. Each phase involves constant refinement and experimentation. Any delay or operational friction, like retrieving data from cold storage, increases the time and costs of developing high-quality AI-enabled products.
The Tradeoffs Due to High Storage Costs
Platforms like Splunk, while valuable, are perceived as costly. Based on their pricing on the AWS Marketplace, retaining one gigabyte of hot data for a month can cost around $2.19. Compare that to AWS S3 object storage, where costs start at $0.023 per GB. Although these platforms add value to the data through indexing and other processes, the fundamental issue remains: Storage on these platforms is expensive. To manage costs, many platforms adopt aggressive data retention policies, keeping data in hot storage for 30 to 90 days – and often as little as seven days – before deletion or transfer to cold storage, where retrieval can take up to 24 hours.
When data is moved to cold storage, it typically becomes dark data – data that is stored and forgotten. But even worse is the outright destruction of data. Often promoted as best practices, these include sampling, summarization, and discarding features (or fields), all of which reduce the data’s value vis-a-vis training ML models.
The Need for a New Data Storage Model
Current observability, SIEM, and data storage services are critical to modern business operations and justify a significant portion of corporate budgets. An enormous amount of data passes through these platforms and is later lost, but there are many use cases where it should be retained for LLM and GenAI projects. However, if the costs of hot data storage aren’t reduced significantly, they will hinder the future development of LLM and GenAI-enabled products. Emerging architectures that separate and decouple storage allow for independent scaling of computing and storage and provide high query performance, which is crucial. These architectures offer performance akin to solid-state drives at prices near those of object storage.
In conclusion, the primary challenge in this transition is not technical but economic. Incumbent vendors of observability, SIEM, and data storage solutions must recognize the financial barriers to their AI product roadmaps and integrate next-generation data storage technologies into their infrastructure. Transforming the economics of big data will help fulfill the potential of AI-driven security and observability.