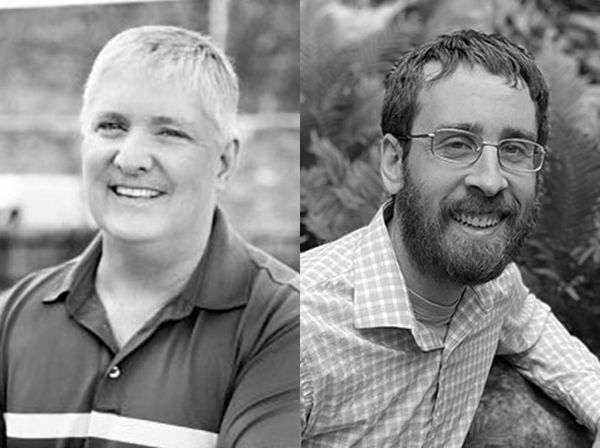
It’s been an exciting year in AI, machine learning, and NLP, with text-to-image generators and large language models delivering some very impressive results and a lot of promise for the future – while noting all of the important caveats about their shortcomings including mitigating societal biases, the possibility of them being used to generate “fake news,” and their environmental impact.
As we embark on the year 2023, we wanted to think about what the new year in AI, machine learning, and NLP will bring.
Jeff Catlin, Head of Lexalytics, an InMoment Company:
AI goes ROI: The slowdown in tech spending will show up in AI and machine learning in two ways: major new AI methodologies and breakthroughs will slow down, while innovation in AI moves toward “productization.” We’ll see AI get faster and cheaper as the innovation moves into techniques to make deep learning less expensive to apply and faster through models like DistilBERT, where accuracy goes down a bit, but the need for GPUs is reduced.
Growing acceptance of hybrid NLP: It’s fairly common knowledge that hybrid NLP solutions that mix machine learning and classic NLP techniques like white lists, queries, and sentiment dictionaries mixed with deep learning models typically provide better business solutions than straight machine learning solutions. The benefit of these hybrid solutions means that they will become a checkbox item in corporate evaluations of NLP vendors.
Paul Barba, Chief Scientist at Lexalytics, an InMoment Company:
The rise of multimodal learning: The wave of image-generating networks like Stable Diffusion and DALL-E demonstrate the power of AI approaches that understand multiple forms of data – in this case, image in order to generate a picture, and text in order to take in descriptions from a human. While multimodal learning has always been a significant research area, it’s been hard to translate into the business world where each data source is difficult to interact with in its own way. Still, as businesses continue to grow more sophisticated in their use of data, multimodal learning jumps out as an extremely powerful opportunity in 2023. Systems that can marry the broad knowledge conveyed in text, image, and video with sophisticated modeling of financial and other numeric series will be the next stage in many companies’ data science initiatives.
The singularity in our sights? A research paper by Jiaxin Huang et al. was published this past October with the attention-grabbing title “Large Language Models Can Self-Improve.” While not yet the singularity, the researchers coaxed a large language model into generating questions from text snippets, answering the self-posed question through “chain of thought prompting,” and then learning from those answers in order to improve the abilities of the network on a variety of tasks. These bootstrapping approaches have historically had a pretty tight bound to improvement – eventually, models start teaching themselves the wrong thing and go off the rails – but the promise of improved performance without laborious annotation efforts is a siren song to AI practitioners. We predict that while approaches like this won’t drive us into a singularity moment, it will be the hot research topic of 2023 and by the end of the year will be a standard technique in all state-of-the-art, natural language processing results.
In summary, 2023 is expected to bring about a shift in the focus of AI and machine learning towards productization and cost-effectiveness, as well as an increased adoption of hybrid NLP solutions. The use of multimodal learning, which involves understanding multiple forms of data such as text, image, and video, is also expected to become more prevalent in businesses. Additionally, research on self-improving large language models is expected to continue to be a major focus in the field, with the potential for these models to become a standard technique in natural language processing. However, it is important to consider the potential challenges and limitations of these advances, such as societal biases and the possibility of misuse.