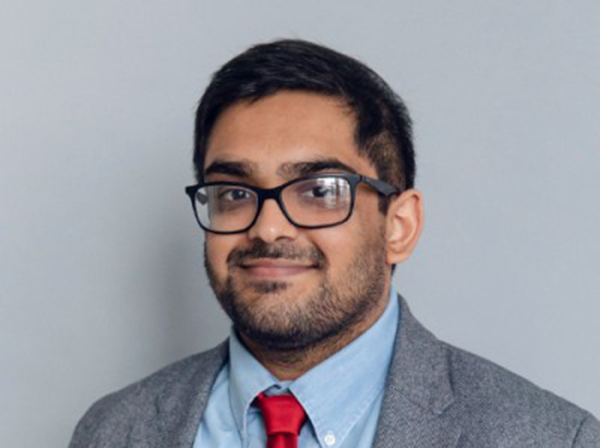
Typical business intelligence implementations allow business users to easily consume data specific to their goals and daily tasks. The ability to analyze both past and present events unlocks information about the current state and is essential for remaining competitive in today’s data-forward market. With that in mind, there are some common business intelligence challenges that many organizations encounter when relying on these tools alone.
Limitation 1: Useful Insights, Trends, and Patterns Arise Only When Looking at the Right Data, Within the Proper Context
The good news is that a modern data warehouse eliminates the risk of reporting on inaccurate or untimely data by organizing information in a manner that enables fast and reliable reporting. That being said, you must also rely on your business users to ask the right questions to develop helpful reports. This often results in a delayed discovery of vital insights and overlooking key data. Additionally, you have higher chances of missing key insights due to human error and the inability for efficient reporting to fully cover all segments of detailed data. Even the best dashboard can exclude important information since they focus solely on specific business questions.
For example, at a logistics company, dashboarding shows every detail around the supply chain and warehouse inventory. With so many variables that could affect the timeliness of your orders (number of employees, truck availability windows, congestion in areas of warehouses, etc.) it’s nearly impossible to combine all of the information and see the bigger picture in a timely manner. Especially when changes are happening in real time. It is also hard for a single person to separate the individual events from the overall effects. With machine learning you can ingest large amounts of data to identify orders at risk of being late based on key variables. Using statistical techniques, you can differentiate the sources of inefficiencies by cutting through all the noise in your data to find systemic issues.
Limitation 2: There Is a Reliance on Static – and Sometimes Arbitrary – Business Rules
Many effective dashboards use benchmark metrics to show if a department is doing well or not. For example, a sales organization has business intelligence tools that use data to track engagement with their leads. Under their current business rules, a lead is considered “cold” if there hasn’t been communication in five days. When a lead goes cold, the sales and management teams are alerted so action can be taken to re-engage the lead. A good dashboard would somehow present the number of cold leads and the number of leads at risk of becoming cold. But how do you really know that five days is the appropriate amount of time? What if millions of data points show that leads are likely to go cold if you haven’t contacted them in two days? That could be a lot of missed opportunities. In such a fast-changing environment, sometimes even based on the context of your business questions, business rules set by people may be misunderstood, inaccurate, or outdated.
Limitation 3: Since Most Business Intelligence Tools Utilize Historical Data, They Lend Themselves to Highlighting Past Events Rather Than Future Ones
Visualizations based on this information are framed around questions of what has happened or is happening. While there is no doubt that understanding the past is essential to improving future decision-making, adding on a layer of predictive analytics would enable a culture of reactive, data-driven decisions to shift toward more forward-thinking and innovative choices.
Using advanced analytics to look toward the future is a practice all businesses should employ. To exemplify the significant impacts this practice can have, we will look to the healthcare industry. Many medical providers track their re-admission rates, how often a patient returns with related health problems after they have been discharged. This metric helps evaluate the quality of care among other factors. Using data science, they can zone in on certain subsets of patients who pose a high risk of readmission. This provides health care providers with real-time knowledge of their most at-risk patients, allowing them to make proactive actions so their patients leave healthy and with less chance of readmission. This proactive approach is much more effective than looking back at historical data to later figure out which subsets of patients had higher readmission.
How to Solve Business Intelligence Challenges with Data Science
Data science helps businesses extract insights from large amounts of data and create outputs to automatically detect significant changes that may arise from patterns spotted in data. In many cases, it is because of the benefits of data science initiatives that companies begin to see significant ROI on their data investments. This is because data science better equips you to:
- Make predictions for future events based on trends in historical data
- Detect significant changes in business events and in determining their outcome
- Assess potential results of business decisions
- Analyze broad sets of data with many inputs to find key insights
- Understand data points that impact the whole company rather than a specific siloed department
While the benefits of data science are undisputed, for many organizations, data science initiatives seem unapproachable. Whether it’s because your data science team finds it difficult to consistently communicate insights, there is a lack of understanding as to how a prediction is being reached, or you don’t know where to start because the process seems so large-scale, your company is not alone. One of the easiest ways to tackle these challenges is to combine your current business intelligence tool and analytics practices with data science.