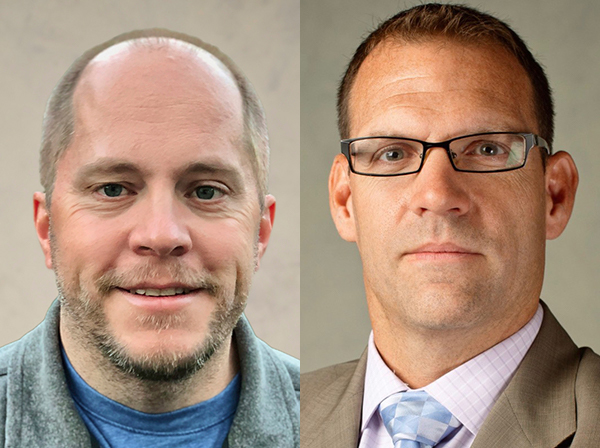
There is no shortage of hype about AI’s potential. But to truly realize that value, we must deploy it in the field repeatedly and reliably in an ever-changing world – and therein lies the challenge. For AI to work sooner, better, and faster, organizations must operationalize more AI programs so they can start collecting and learning from real-world data. This is essential to moving algorithms from the lab to the field, scaling them, and improving AI readiness. One solution? AI engineering.
AI engineering is both a necessity and a game-changer for ROI. In fact, according to a prediction from David Groombridge, research vice president at Gartner, “By 2025, the 10% of enterprises that establish AI engineering best practices will generate at least three times more value from their AI efforts than the 90% of enterprises that do not.”
Our team has been working with clients in the federal government to build a more robust and repeatable AI engineering approach. How do you achieve sustainability in your AI efforts? How do you make AI a coordinated effort? Most importantly, where should you invest and what frameworks should you deploy to scale AI? Here are three approaches to consider:
1. Move AI Applications from the Cloud to the Edge
Cloud computing is one of the most disruptive technologies in recent years and will continue to play a critical role supporting AI moving forward. However, we see the rise of edge computing as a complement to cloud, filling gaps where cloud may not be well-suited.
Edge computing refers to computing workloads executed at the point of data collection. This is often expressed as a machine learning process that extracts useful insights from raw data, collected through sensors such as a mobile phone, satellite, or camera. Moving analytics closer to the point of data collection is crucial because it reduces the time from data to decision. Given the recent and dramatic increase in data due to IoT networks, expanding digital footprints, the emerging metaverse, and more, organizations must be able to move more AI applications from the cloud to the edge.
This increase in data has been followed by a decrease in the response rate required for AI-supported decisions at the point of application, and brittleness of edge infrastructure. By reducing latency, 5G addresses some of this challenge, enabling AI applications to send data back to the cloud for storage and processing. Yet storage and processing costs for this volume of data may be prohibitive, and certain edge deployments, like autonomous vehicles, will still require processing at the point of application.
Going forward, AI applications must move and operate effectively at the edge with fewer resources, such as storage, memory, and compute. This will also result in increased use of federated learning due to the requirements of training models in a distributed way at the edge, while also meeting data security requirements for sensitive data by mitigating the need for massive co-aggregation.
2. Increase the Use of Reinforcement Learning in the Metaverse
Scaling AI requires massive amounts of data. We expect to see an increased use of reinforcement learning in the metaverse, using synthetic environments to generate data to support AI training. Why? Many AI problems with readily available data have already been addressed today, but to allow the development of additional AI solutions without readily available data, synthetic data – data created in digital worlds rather than collected from or measured in the real world – is required.
Through the use of synthetic environments, applying reinforcement learning, it will be possible to generate data and conduct iterative development for training AI applications without sufficient data. Moreover, synthetic data will help augment model training – in particular, helping to reduce inherent bias found in real data and increase overall precision and recall. These models will then be transferred to the real world and refined to meet required performance thresholds while reducing overall model training timelines.
3. Adopt an AI Operations Framework
Scalable AI development and deployment requires an AI Operations (AIOps) framework. Such a framework helps close the gap between conceptual innovation and real-world deployment and ensures that critical ethics, security, and privacy components are prioritized early in development.
This framework, employed by a dedicated AI team focused on responsible AI and human-centered design, should have several key components, including mission engineering; DataOps, MLOps, and DevSecOps; systems and reliability engineering; infrastructure and cybersecurity engineering; and operational feedback loops. AIOps can bring many technical benefits to an organization, including reducing the maintenance burden on individual analysts while maximizing subject matter experts’ productivity and satisfaction.
Ultimately, AI’s end game is to drive broad adoption, so our most critical future challenge is repeatably and reliably developing and deploying AI. Deploying these AI engineering best practices will be a crucial part of rising to this challenge. After all, in the global competition for AI supremacy, the capabilities that give the U.S. an edge today will not be enough to win in the future.