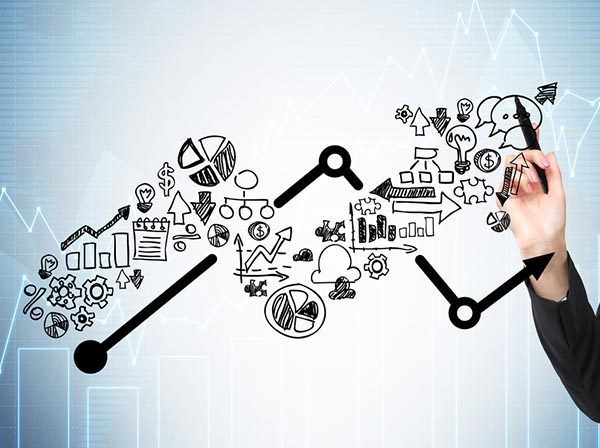
In 2023, organizations that switch to advanced analytics will be better positioned to leverage new opportunities in their respective industries. Increasingly, with the use of automated data analytics, companies are turning to analytics systems and advanced analytics use cases for strategy transformation and digital strategy.
Advanced analytics systems are gradually upgrading many companies, creating business value, and looking at how companies can better use their data products. As the world moves towards a more data-driven economy, the need to bring growing challenges to the forefront of data science will remain a growing priority.
In 2023, advanced analytics use cases will be implemented to better automate processes, enable more accurate predictions, increase workflow efficiencies, and empower workforces. Machine learning (ML) and natural language processing (NLP) will be used to process data in a manner similar to how humans work and provide data-driven insights.
Advanced analytics helps data teams develop new ideas and strategies that bring continuing shifts in how they can better serve their customers. Some keys for business analytics trends for 2023 include:
- The rise of cloud-native market for business analytics
- As the insurance industry makes a digital shift, nearly 90% of insurers are expected to leverage artificial intelligence and machine learning for better customer engagement
- Data access methods more democratized
- Automation in data storing and cleaning processes
- Continued empowerment of whole workforces through self-service analytics
- The integration of artificial intelligence (predictive analytics) into insurance applications
- Demand for more data literacy across business users
- Use of big data to gain insights into business customers, products, operations, and markets
- Increased use of AI in data analytics to analyze customer behaviors, business processes, and enterprise data
- Data Governance will move beyond managing unstructured data to managing huge data traffic
Data regulations will shape new types of data stores
Production AI systems will also become more advanced, allowing businesses to optimize their IT systems and have greater control over decision-making
Modern self-service analytics platforms empower the ordinary business user by bringing advanced analytics tools to their desktop. The business user today does not require the help of a technical team member to discover trends and patterns, make accurate predictions about the future, or drive a data-driven culture in the organization. Within the AI category, “explainable AI” seems to be a hot topic these days as well. Augmented analytics, continuous intelligence, and explainable AI are completely disrupting the business analytics landscape right now,
According to Rita Sallam, research vice president at Gartner:
“Data and analytics leaders must examine the potential business impact of these trends and adjust business models and operations accordingly, or risk losing competitive advantage to those who do.”
In the augmented and advanced analytics scenario, most of the critical tasks like Data Quality Management, Data Integration, Master Data Management, and Metadata Management are automated to a large extent. Donald Feinberg, vice president and distinguished analyst at Gartner, thinks that the “digital disruption” has created as many challenges as opportunities.
Advanced Analytics with IoT Data: Use Cases
More modern companies are realizing the potential of big data in analyzing digital information and using advanced models to simulate different scenarios in order to make more accurate decisions in market contexts. Advanced analytics systems can help organizations make more appropriate decisions based on the types of data available without any human intervention.
Advanced analytics use cases include:
- Investing in data culture and data literacy throughout an enterprise to reap the maximum rewards of data analytics
- Innovating improved data-access methods to empower common users within an organization
- Development of user-friendly interfaces for analytics systems, which allow users easy access to their insights without requiring complex technical know-how
- Development of AI tools to equip both data engineers and data scientists with automated tools to work more efficiently
- Leveraging analytics-managed-services to simplify business review tasks and provide relevant analytics
- Service tools such as Infor AI Datamarts can be used to manage data and make it easier for teams to share data across the organization
Some popular applications of IoT data analytics include:
- Product usage analysis
- Common analytics for consumers and business users
- Sensors and cameras working in conjunction for analyzing co-occurring or connected events
- Video analytics for surveillance and safety steps
- Social analytics
The critically important aspect of IoT data analytics is that though business users may believe that modern hardware innovations like wireless, sensors, and mobile are driving business value, in reality it is the high quality of sensor data and advanced analytics technologies like big data that are contributing to value-added analytics.
Advanced Analytics Use Cases by Industry
Recently, business leaders and managers have been paying a lot of attention to building an advanced analytics vision and strategy, which involves serious considerations about the applicability of such analytics exercises. The advanced analytics vision and strategy are often closely linked to the overall business strategy, so that the primary goal of achieving operational efficiency is achieved.
Modern AI platforms offer many automated or semi-automated tools that may be easily used by finance, insurance, or health care business professionals to transform data into information for smarter decision-making and enhanced profitability. As Data Governance is also very important in these three industry sectors, machine learning (ML) enabled AI platforms offer even greater opportunities for more accurate and more efficient decision-making. These advanced analytics technologies are helping businesses to stand apart from their competitors.
To find out how predictive analytics will help make decisions for the future, review Predictive Analytics Use Cases, which suggests that the true power of advanced analytics rests as much with trained experts as with advanced tools.
Retail
- Users can apply predictive analysis in conjunction with prescriptive analysis on high volumes of market and consumer data to arrive at actionable intelligence
- Predictive analytics tools can help users predict sales outcomes for the immediate future
- Past sales performance data, when used in predictive analytics, can help retailers to forecast growth due to particular factors such as change in market trends or consumer behavior
- Big data analytics helps retailers study product-distribution channel data to reduce costs
Insurance and Financial Services
- Advanced predictive analytics is used for churn management
- Big data, along with predictive analytics, can help in forecasting demand
- Big data analytics plays a vital role in fraud prevention
- Risk analysis with big data helps to determine credit-worthiness of clients
- Data mining, NLP, and text analysis are used together to deliver better product and customer insights
Health Care
Advanced analytics platforms like Health Catalyst helps in predicting risk of diabetic ketoacidosis (DKA), an acute case of diabetes, which allows early intervention.
Marketing
Understanding consumer behavior is the key to marketing success. In the digital era, marketing data is gathered from a wide variety of input channels and customer touchpoints. Along with structured chat transcripts or emails, many unstructured sources of data, such as sensors, click streams, and social media generate piles of consumer data.
Conclusion
In 2023, more organizations than ever have implemented advanced analytics use cases for gathering data and cleansing it. The role of workforces is also evolving with the implementation of AI and ML in analytics, so that data scientists and business analysts can focus more on higher-level tasks such as deriving insights from data rather than cleaning it manually.
Advanced analytics use cases should also be able to provide recommendations on cost reduction and optimization based on real-time analysis of the data set, thus helping businesses remain competitive in an ever-changing market environment.
In 2023, the focus for many businesses will be to show their investments in the data and analytics arena. Predictions for the next few years suggest a “recessionary environment”; however, businesses can still continue to use data analytics to secure greater reliability and focus on number-driven decision-making.
Image used under license from Shutterstock.com